Deep Neural Networks For Activity Recognition With Multi-Sensor Data In A Smart Home
2018 IEEE 4TH WORLD FORUM ON INTERNET OF THINGS (WF-IOT)(2018)
摘要
Multi-sensor based human activity recognition is one of the challenges in the ambient intelligent environments such as smart home and smart city. Ordinary people in their daily lives usually share a similar and repetitive life pattern, also known as life cycle. Smart home environment and its multi sensors can provide assistance to human by collecting the data sequence of human activities to predict the desired actions. Our goal is to analyze the sequence of activities recorded by a specific resident using deep learning with multiple sensor data. In this paper, we train the multiple sensor data collected by a smart home using several deep neural networks. According to the characteristics of the Recurrent Neural Network (RNN) structure, multiple sensor data of smart home is suitable for RNN because it has a sequence data in time. To support our assumption, we proposed the Residual-RNN architecture to predict future activities of a resident. Furthermore, we also utilized attention module to filter out the meaningless data to have more effective results than the one without. To verify our proposed idea, we used real resident activity in smart home using Massachusetts Institute of Technology (MIT) dataset. After our experiments, our proposed model with attention mechanism outperform the Long Short-Term Memory (LSTM) and Gated Recurrent Unit (GRU) model in terms of predicting the desired activities of a smart home resident.
更多查看译文
关键词
Recurrent neural networks, Attention, Deep learning, Human activity recognition, Activity of daily life (ADL), Smart home
AI 理解论文
溯源树
样例
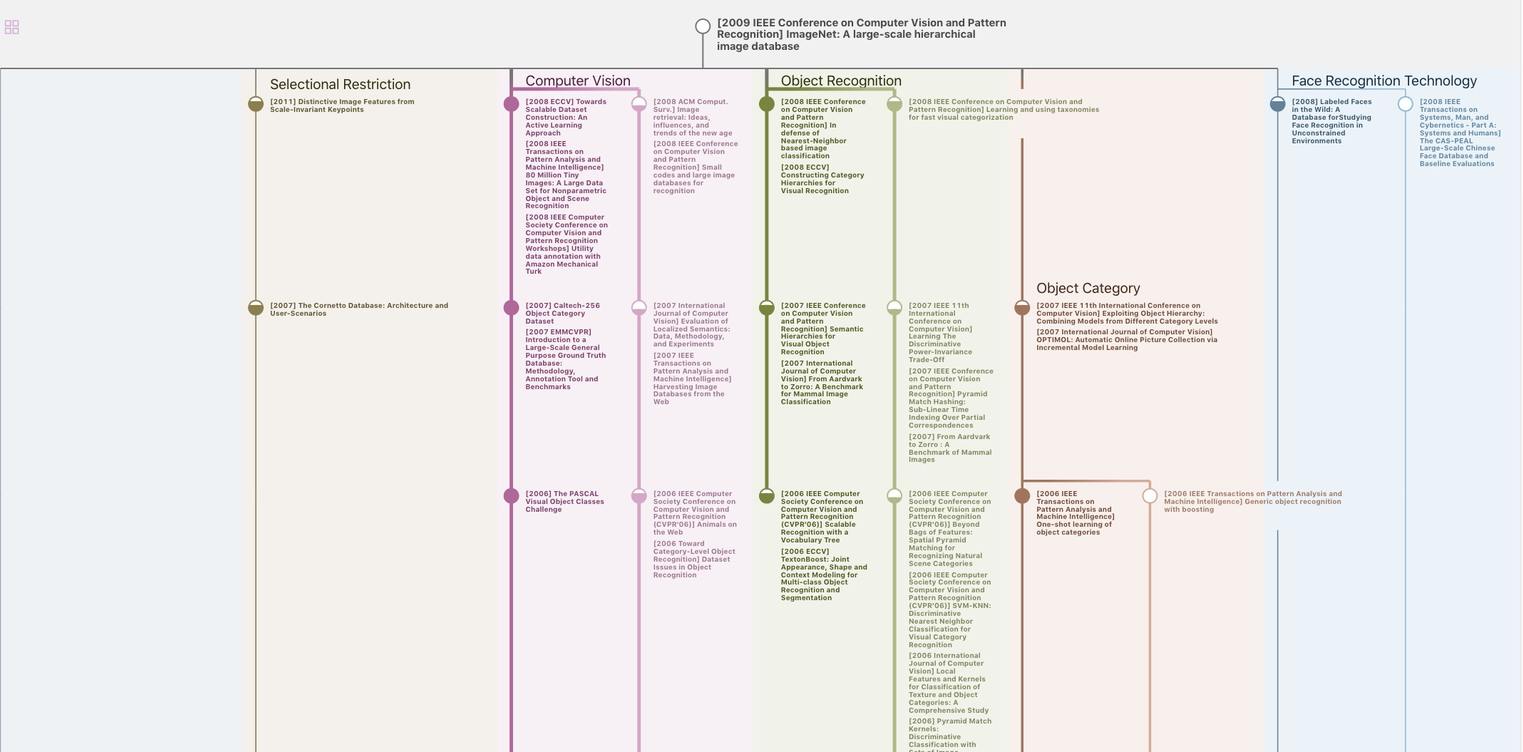
生成溯源树,研究论文发展脉络
Chat Paper
正在生成论文摘要