Deep Multi-Task And Task-Specific Feature Learning Network For Robust Shape Preserved Organ Segmentation
2018 IEEE 15TH INTERNATIONAL SYMPOSIUM ON BIOMEDICAL IMAGING (ISBI 2018)(2018)
摘要
Fully convolutional network (FCN) has shown potency in segmenting heterogeneous objects from natural images with high run-time efficiency. This technique, however, is not able to produce continuous, smooth and shape-preserved regions consistently due to complex organ structures and occasional weak appearance information commonly observed in various anatomical structures in medical images. In this paper, we propose a deep end-to-end network with two task-specific branches to ensure continuousness, smoothness and shape-preservation in segmented structure without additionally sophisticated shape adjustment, e.g., dense conditional random fields. The novelties of the proposed method lie in three aspects. First, we formulate the organ segmentation as a multi-task learning process that combines both region and boundary identification tasks, which can alleviate spatially isolated segmentation errors. Second, we use boundary distance regression to ensure the smoothness of the segmented contours, instead of formulating boundary identification as a classification problem [1]. Third, our deep network is designed to have a "Y" shape, i.e., the first half of the network is shared by both region and boundary identification tasks, while the second half is branched for each task independently. This architecture enables the task-specific feature learning for better region and boundary identification, and offers information for segmentation refinement based on a fusion scheme using energy functional. Extensive evaluations are conducted on a variety of applications across organs and modalities, e.g., MR femur, CT kidney, etc. Our proposed method shows better performance compared to the state-of-the-art methods.
更多查看译文
关键词
Deep end-to-end network, multi-task and task-specific learning, shape preserved organ segmentation
AI 理解论文
溯源树
样例
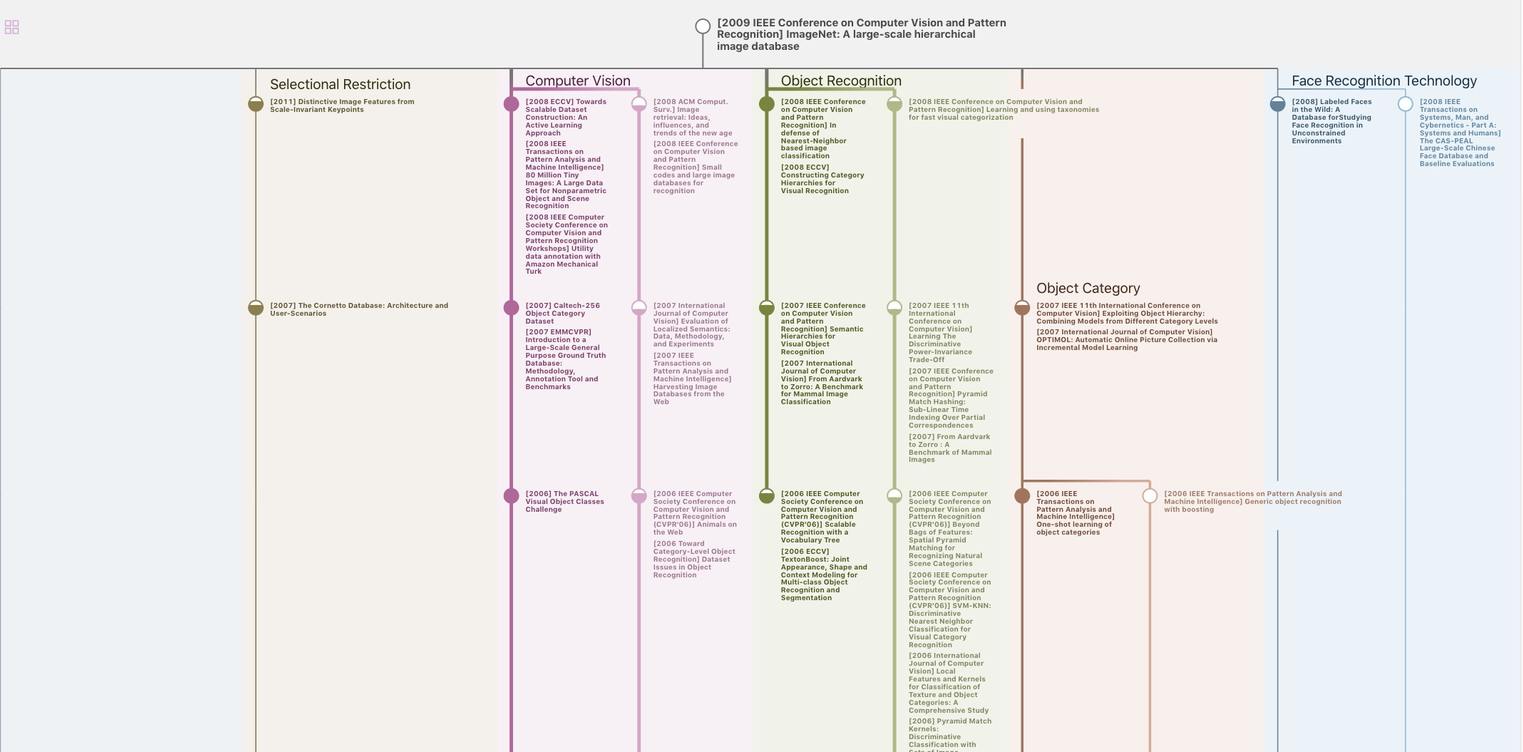
生成溯源树,研究论文发展脉络
Chat Paper
正在生成论文摘要