Longitudinal And Multi-Modal Data Learning For Parkinson'S Disease Diagnosis
2018 IEEE 15TH INTERNATIONAL SYMPOSIUM ON BIOMEDICAL IMAGING (ISBI 2018)(2018)
摘要
In this paper, we propose a joint regression and classification scheme for Parkinson's disease (PD) diagnosis using longitudinal multi-modal neuroimaging data. Specifically, we devise a new feature selection method imposing three kinds of relational constraints in a unified multi-task feature selection model. These relations (e.g., relations among features, responses, and subjects) are consolidated to represent the similarities among them. The baseline data is utilized to establish the feature selection model exploiting five regression variables (four clinical scores and a clinical label) to jointly select the most discriminative features. Namely, we exploit baseline data to train four regression models for clinical scores prediction and a classification model for discriminating the clinical label in the future time point. Extensive experiments are conducted to demonstrate the effectiveness of the proposed method on the Parkinson's Progression Markers Initiative (PPMI) dataset. The experimental results demonstrate that our proposed method can enhance the performance in clinical scores prediction and class label identification in longitudinal data and outperforms the state-of-art methods as well.
更多查看译文
关键词
Parkinson's disease, feature selection, classification, score prediction, longitudinal data
AI 理解论文
溯源树
样例
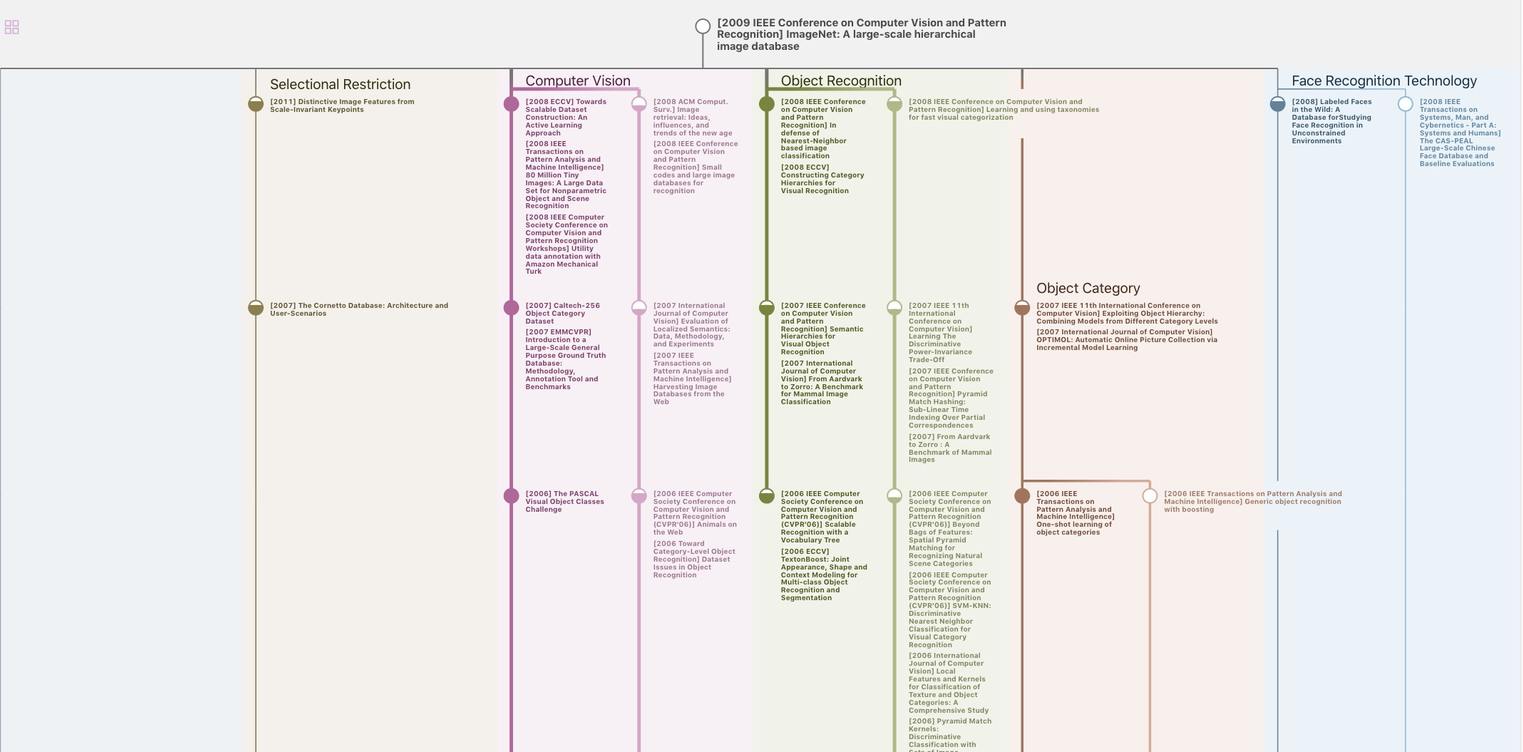
生成溯源树,研究论文发展脉络
Chat Paper
正在生成论文摘要