Weighted group sparse functional connectivity modeling for Alzheimer's disease identification
2018 IEEE 15TH INTERNATIONAL SYMPOSIUM ON BIOMEDICAL IMAGING (ISBI 2018)(2018)
摘要
Constructing functional connectivity/interactions networks enables better understanding of pathological underpinnings of neurological disorders. Functional connectivity network, as a simplified representation of those structural or functional interactions, has been widely used for diagnosis and classification of neurodegenerative diseases. Motivated by recent progress in functional connectivity network analysis for Alzheimer's disease (AD). In this paper, we propose a novel adjusted cosine similarity weighted group sparse (ACS-WGS) representation method for brain functional network construction. Then, we extract functional connectivity as features from the constructed connectivity networks. Finally, we use support vector machine (SVM) for classification. The experimental results on ADNI2 dataset demonstrate outperform performance. Furthermore, compared with the conventional connectivity network-based methods, our proposed method have further improved the classification performance and discover changed functional connectivity, which could be important for disease diagnosis.
更多查看译文
关键词
Alzheimer's disease, Functional connectivity networks, Weighted Group Sparse
AI 理解论文
溯源树
样例
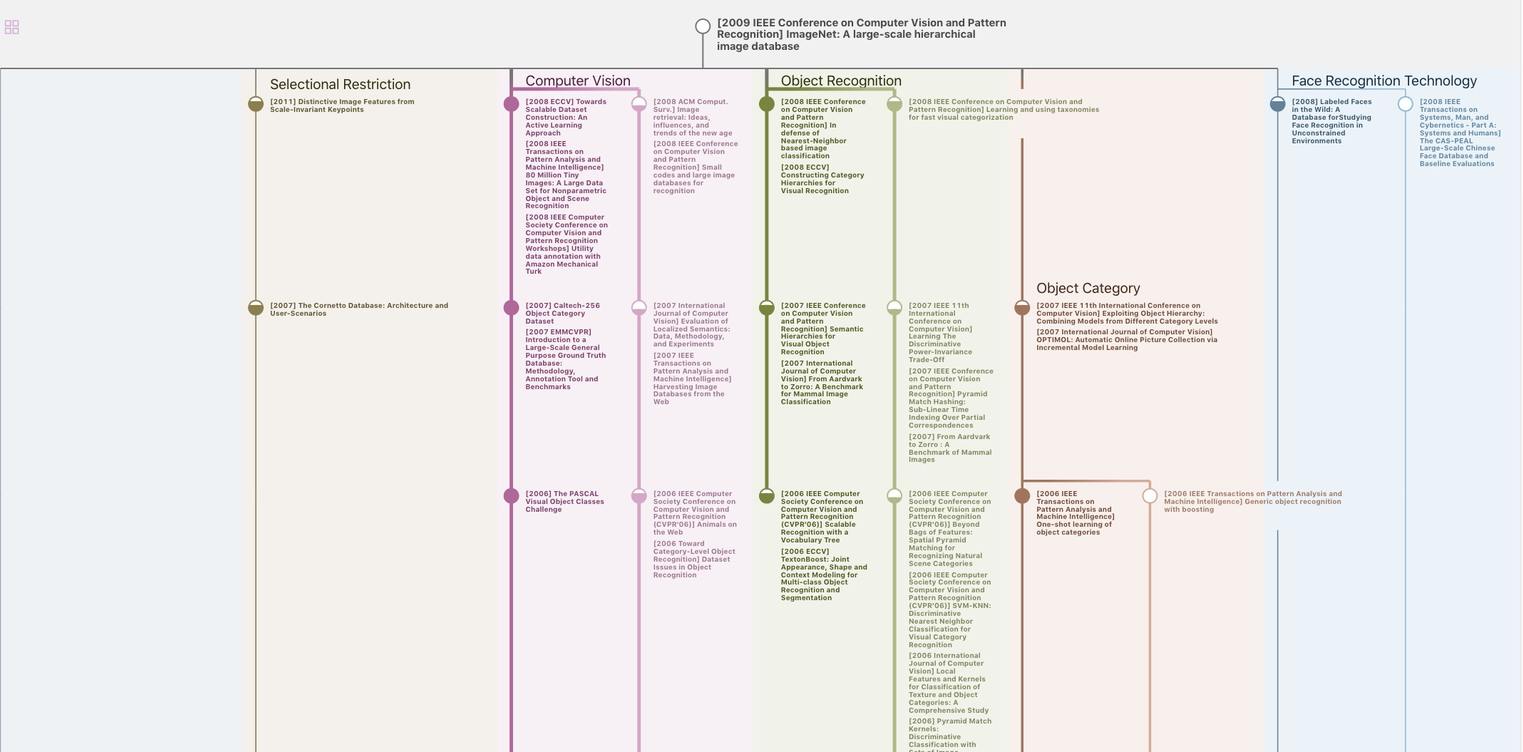
生成溯源树,研究论文发展脉络
Chat Paper
正在生成论文摘要