Dynamic Online Pricing with Incomplete Information Using Multi-Armed Bandit Experiments
MARKETING SCIENCE(2019)
摘要
Pricing managers at online retailers face a unique challenge. They must decide on real-time prices for a large number of products with incomplete demand information. The manager runs price experiments to learn about each product's demand curve and the profitmaximizing price. In practice, balanced field price experiments can create high opportunity costs, because a large number of customers are presented with suboptimal prices. In this paper, we propose an alternative dynamic price experimentation policy. The proposed approach extends multiarmed bandit (MAB) algorithms from statistical machine learning to include microeconomic choice theory. Our automated pricing policy solves this MAB problem using a scalable distribution-free algorithm. We prove analytically that our method is asymptotically optimal for any weakly downward sloping demand curve. In a series of Monte Carlo simulations, we show that the proposed approach performs favorably compared with balanced field experiments and standard methods in dynamic pricing from computer science. In a calibrated simulation based on an existing pricing field experiment, we find that our algorithm can increase profits by 43% during the month of testing and 4% annually.
更多查看译文
关键词
dynamic pricing,e-commerce,online experiments,machine learning,multiarmed bandits,partial identification,minimax regret,nonparametric econometrics,A/B testing,field experiments
AI 理解论文
溯源树
样例
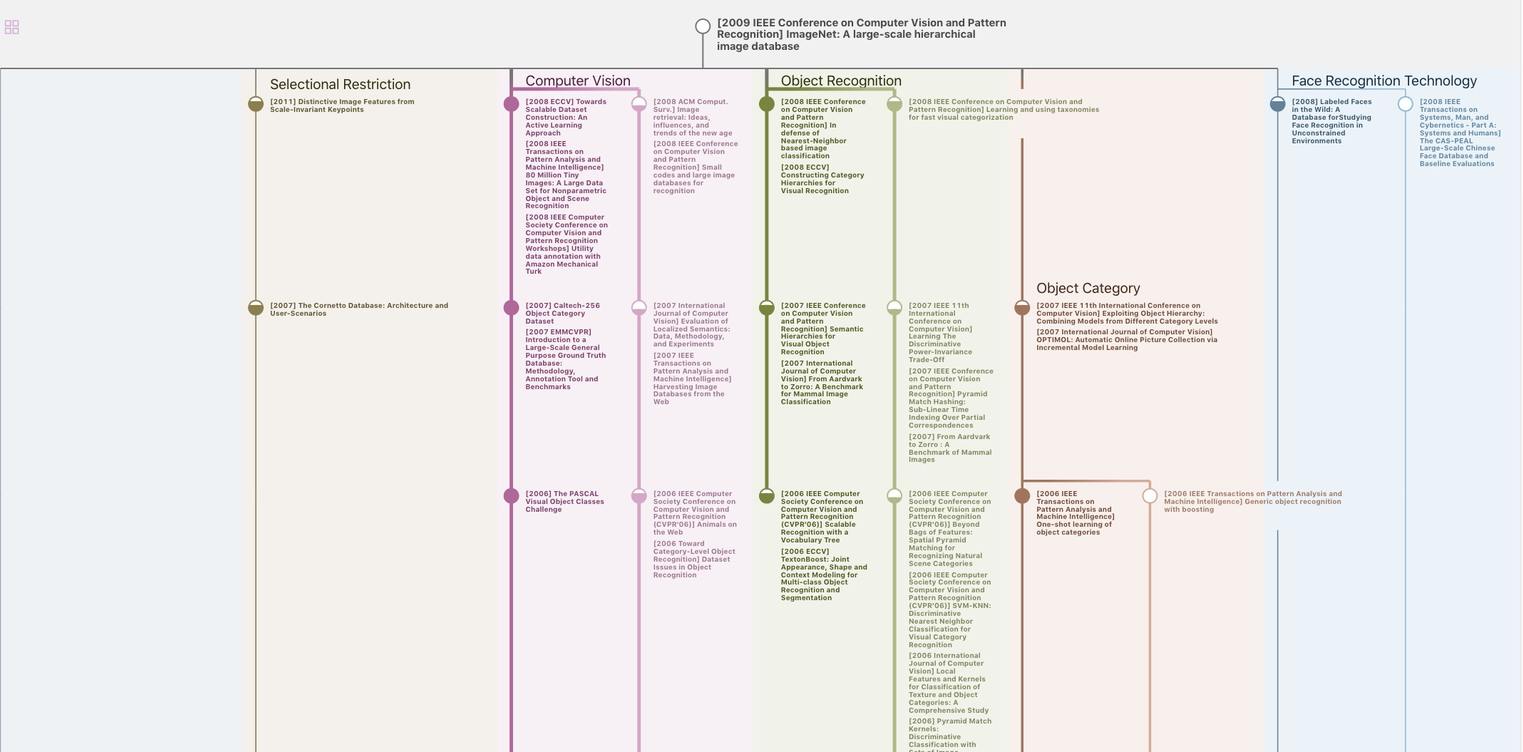
生成溯源树,研究论文发展脉络
Chat Paper
正在生成论文摘要