Special Issue of BICS 2016
Cognitive Computation(2018)
摘要
Brain-inspired cognitive models and algorithms are important components driving artificial intelligence (AI). Deep neural networks are currently considered the most effective models to yield high perception and inference performance by learning from big data. However they manifest inferior generalization, robustness, interpretability, and adaptability when compared to the human brain. Despite neural circuits and cognition mechanisms of the brain having many unknowns, they continue to inspire AI in different ways. The International Conference on Brain Inspired Cognitive System (BICS) has been organized since 2004 to stimulate interdisciplinary research and exchanges in brain-inspired cognitive systems and applications in diverse fields. The 8th International Conference on Brain Inspired Cognitive System (BICS 2016) was held in Beijing, China, November 28–30, 2016. This special issue aims to report new advances since BICS 2016, by including expanded versions of selected conference papers and also new contributions. Until April 20, 2017, the special issue received 18 submissions, most of which were expanded versions of BICS 2016 conference papers, along with a few new submissions. Following a rigorous peer review process, nine papers were accepted for publication in this special issue. The nine papers present contributions in brain information processing, braininspired cognitive models, and algorithms for decision, learning, vision, and applications. In BAnatomical Pattern Analysis for Decoding Visual Stimuli in Human Brains,^ Yousefnezhad and Zhang propose Anatomical Pattern Analysis (APA) for decoding visual stimuli in the human brain. This framework develops a novel anatomical feature extraction method and a new imbalance AdaBoost algorithm for binary classification. Further, it utilizes an Error-Correcting Output Codes (ECOC) method for multiclass prediction. APA can automatically detect active regions for each category of the visual stimuli. Moreover, it enables us to combine homogeneous datasets for applying advanced classification. Experiments on four visual categories in fMRI data demonstrate the effectiveness of the proposed method.
更多查看译文
AI 理解论文
溯源树
样例
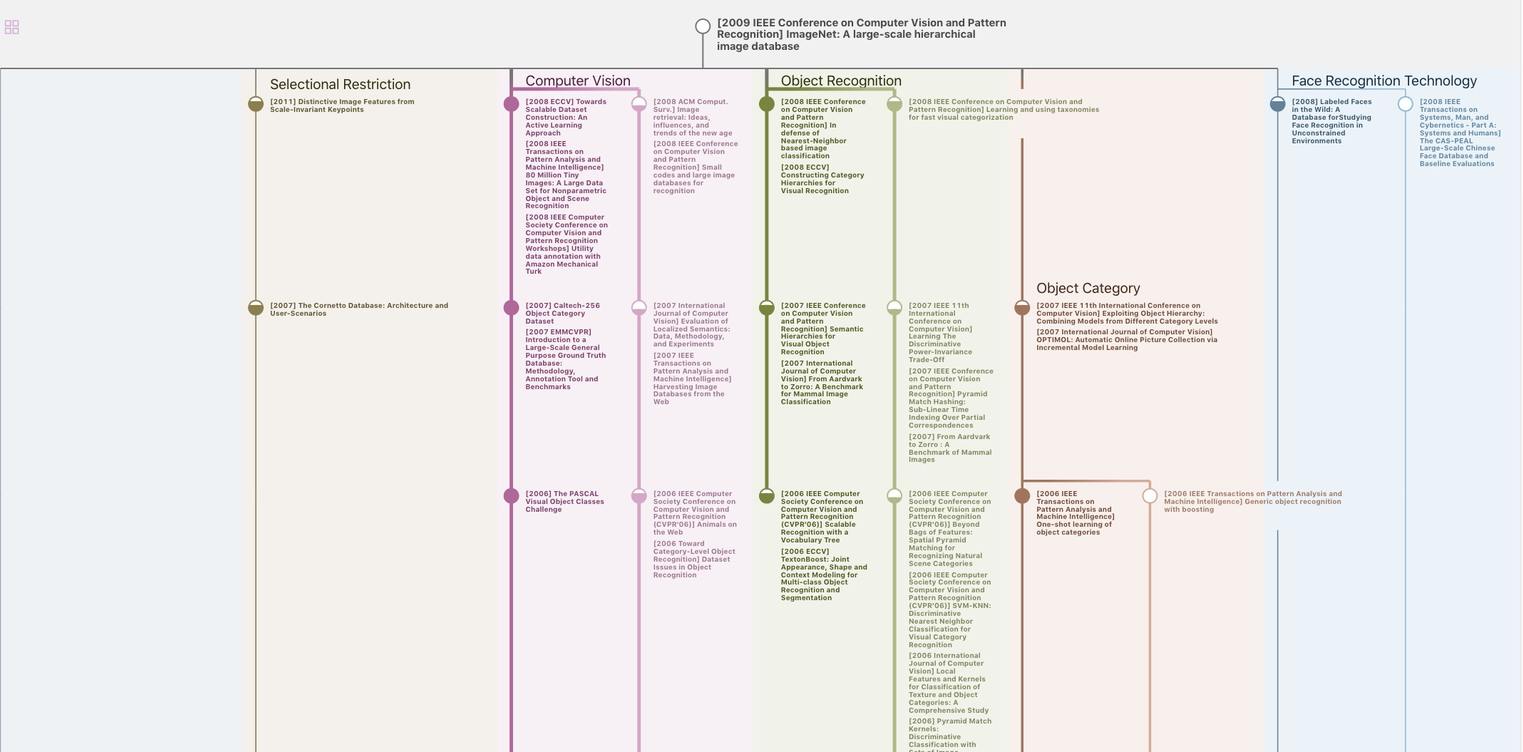
生成溯源树,研究论文发展脉络
Chat Paper
正在生成论文摘要