Verification of Dynamic Behaviour in Qualitative Molecular Networks Describing Gene Regulation, Signalling and Whole-cell Metabolism.
FUNDAMENTA INFORMATICAE(2018)
摘要
We present a tool for the verification of qualitative biological models. These models formalise observed behaviours and interrelations of molecular and cellular mechanisms. During its development a model is continuously verified. Predicted behaviours are compared with behaviours observed in experimental data. Moreover, the model must not exhibit behaviours which contradict existing knowledge about capabilities of the biological system under investigation. Model development is an iterative process involving many rounds of prediction, verification and refinement. Due to the complexity of biological systems this process is laborious and error prone, which motivates the development of "model debugging" tools. The qualitative models we investigate represent large-scale molecular interaction networks describing gene regulation, signalling and whole-cell metabolism. We integrate a steady state model of whole-cell metabolism with a dynamic model of gene regulation and signalling represented as a Petri net. This Quasi-Steady State Petri Net (QSSPN) representation allows the generation of dynamic sequences of molecular events satisfying substrate, activator, inhibitor and metabolic flux requirements at every state transition. The reachability graph of the dynamic part of the model is examined and for every transition in this graph the satisfaction of metabolic flux requirements is verified by well-established linear programming techniques. Our approach is based on network connectivity alone and does not require any kinetic parameters. We demonstrate the applicability of our method by analysing a large-scale model of a nuclear receptor network regulating bile acid homeostasis in human hepatocyte. To date, simulation and verification of QSSPN models have been performed exclusively by Monte Carlo simulation. Random walks through the state space were used to find examples of behaviour satisfying properties of interest. Here, we provide for the first time for QSSPN models an exhaustive analysis of the state space up to a finite depth, which is possible due to several effective optimisations. Contrary to the Monte Carlo approach, we can prove that certain behaviour cannot be realised by the model within a given number of steps. This allows rejection of models which are not capable to reproduce experimentally observed behaviours, as well as verification that biologically unrealistic behaviours cannot occur in the simulation. We show an example of how these features improve identification of problems in large scale network models.
更多查看译文
AI 理解论文
溯源树
样例
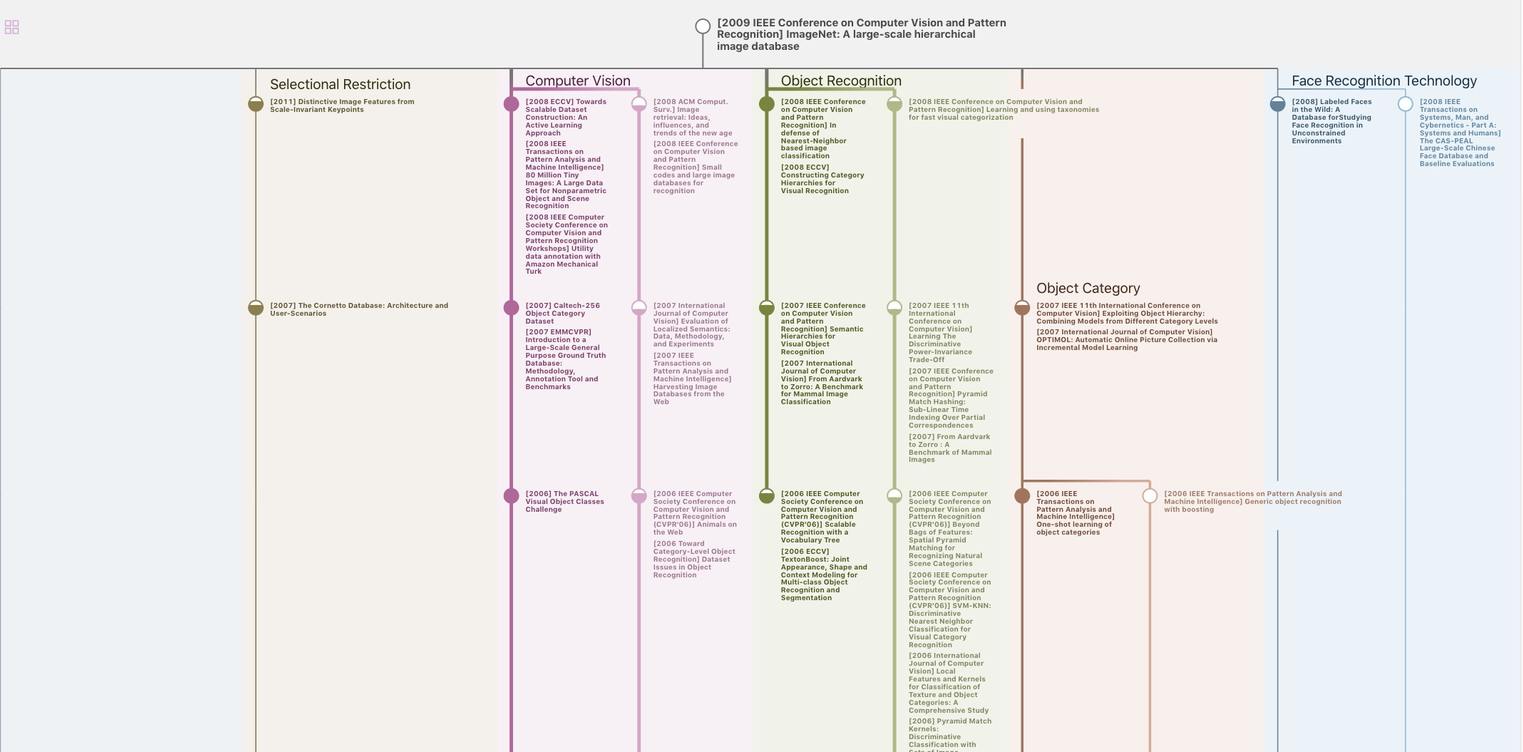
生成溯源树,研究论文发展脉络
Chat Paper
正在生成论文摘要