Diagonalwise Refactorization: An Efficient Training Method for Depthwise Convolutions
arXiv (Cornell University)(2018)
摘要
Depthwise convolutions provide significant performance benefits owing to the reduction in both parameters and mult-adds. However, training depthwise convolution layers with GPUs is slow in current deep learning frameworks because their implementations cannot fully utilize the GPU capacity. To address this problem, in this paper we present an efficient method (called diagonalwise refactorization) for accelerating the training of depthwise convolution layers. Our key idea is to rearrange the weight vectors of a depthwise convolution into a large diagonal weight matrix so as to convert the depthwise convolution into one single standard convolution, which is well supported by the cuDNN library that is highly-optimized for GPU computations. We have implemented our training method in five popular deep learning frameworks. Evaluation results show that our proposed method gains $15.4\times$ training speedup on Darknet, $8.4\times$ on Caffe, $5.4\times$ on PyTorch, $3.5\times$ on MXNet, and $1.4\times$ on TensorFlow, compared to their original implementations of depthwise convolutions.
更多查看译文
关键词
Acceleration,convolutional neural network,depthwise convolution
AI 理解论文
溯源树
样例
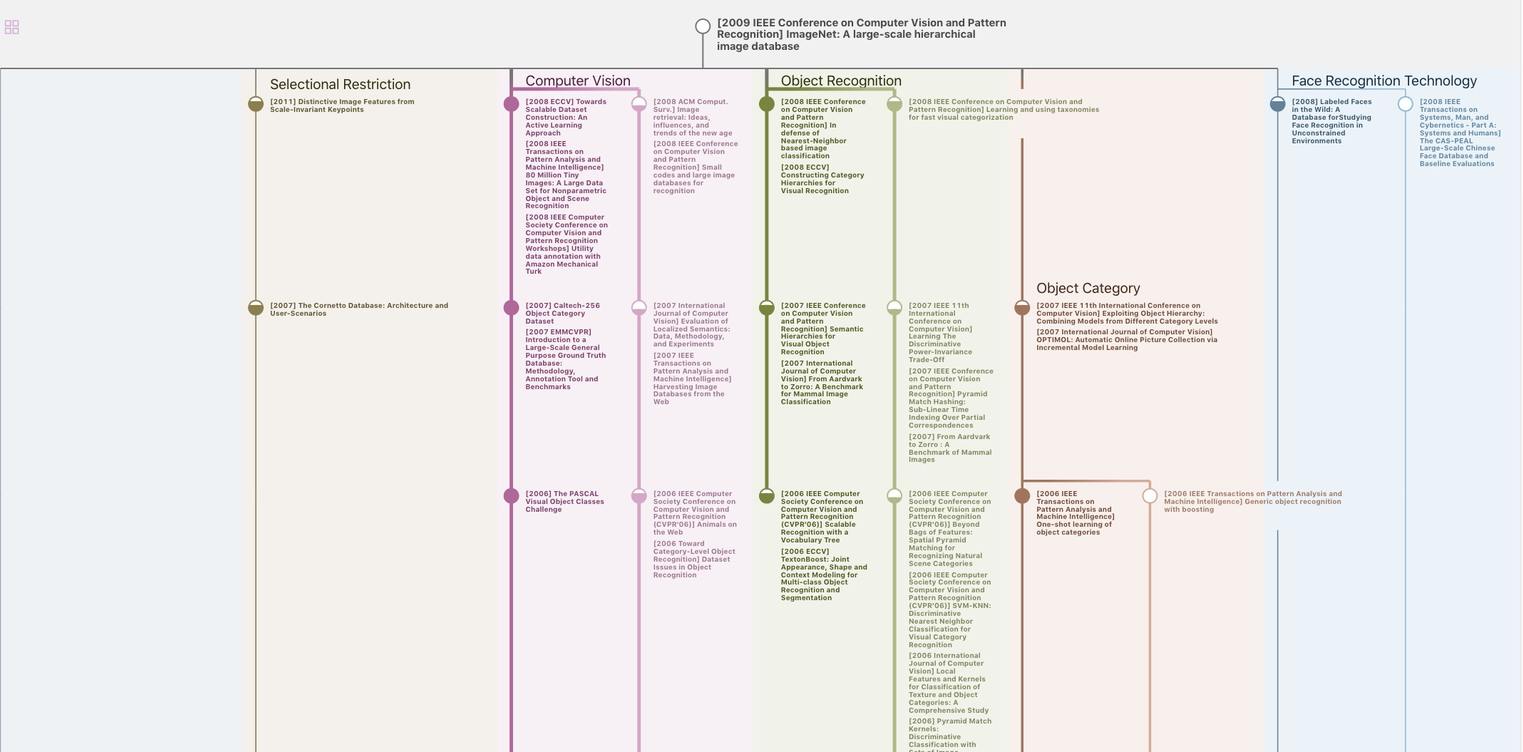
生成溯源树,研究论文发展脉络
Chat Paper
正在生成论文摘要