Using Grouped Linear Prediction and Accelerated Reinforcement Learning for Online Content Caching
2018 IEEE INTERNATIONAL CONFERENCE ON COMMUNICATIONS WORKSHOPS (ICC WORKSHOPS)(2018)
摘要
Proactive caching is an effective way to alleviate peak-hour traffic congestion by prefetching popular contents at the wireless network edge. To maximize the caching efficiency requires the knowledge of content popularity profile, which however is often unavailable in advance. In this paper, we first propose a new linear prediction model, named grouped linear model (GLM) to estimate the future content requests based on historical data. Unlike many existing works that assumed the static content popularity profile, our model can adapt to the temporal variation of the content popularity in practical systems due to the arrival of new contents and dynamics of user preference. Based on the predicted content requests, we then propose a reinforcement learning approach with model-free acceleration (RLMA) for online cache replacement by taking into account both the cache hits and replacement cost. This approach accelerates the learning process in non-stationary environment by generating imaginary samples for Q-value updates. Numerical results based on real-world traces show that the proposed prediction and learning based online caching policy outperform all considered existing schemes.
更多查看译文
关键词
accelerated reinforcement learning
AI 理解论文
溯源树
样例
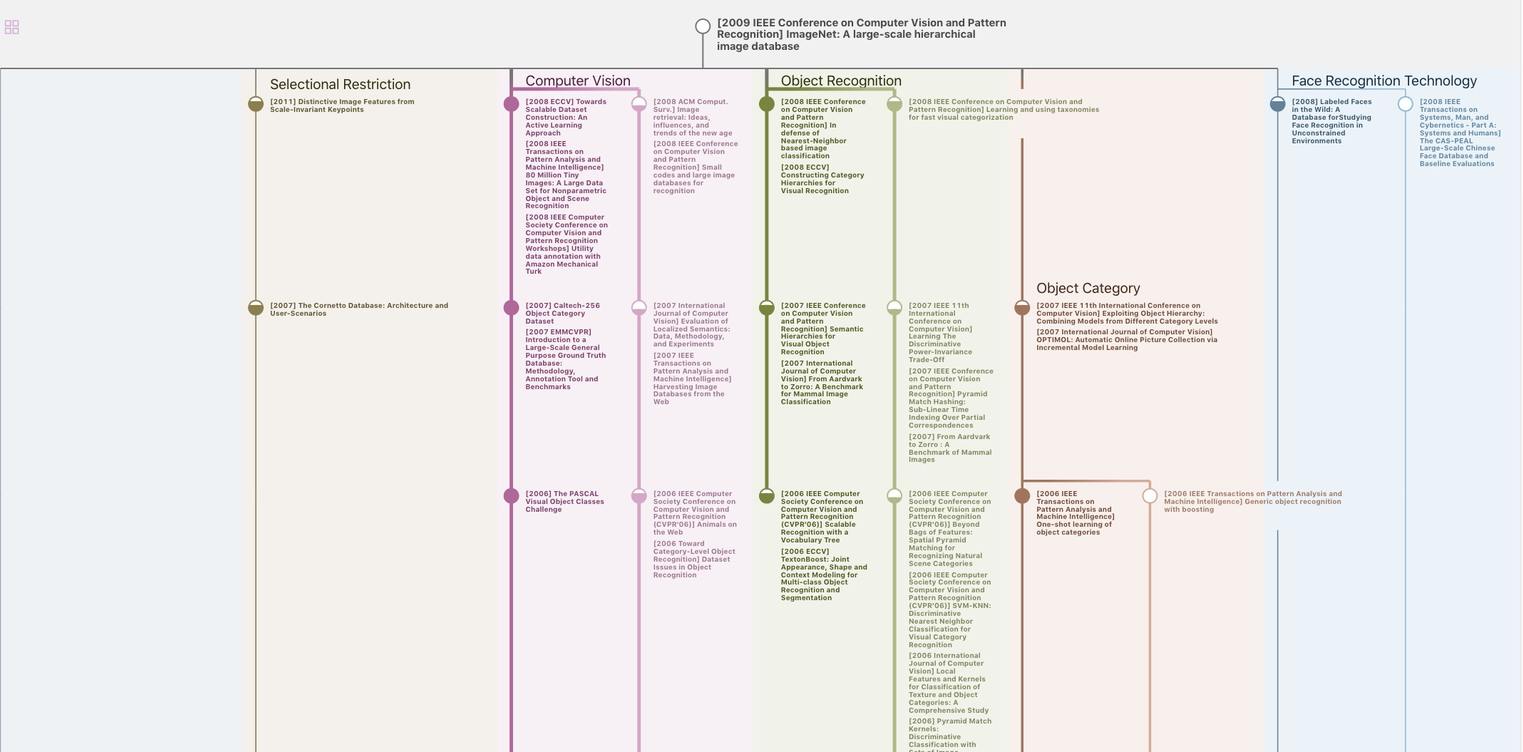
生成溯源树,研究论文发展脉络
Chat Paper
正在生成论文摘要