Deep Component Analysis via Alternating Direction Neural Networks
COMPUTER VISION - ECCV 2018, PT 15(2018)
摘要
Despite a lack of theoretical understanding, deep neural networks have achieved unparalleled performance in a wide range of applications. On the other hand, shallow representation learning with component analysis is associated with rich intuition and theory, but smaller capacity often limits its usefulness. To bridge this gap, we introduce Deep Component Analysis (DeepCA), an expressive multilayer model formulation that enforces hierarchical structure through constraints on latent variables in each layer. For inference, we propose a differentiable optimization algorithm implemented using recurrent Alternating Direction Neural Networks (ADNNs) that enable parameter learning using standard backpropagation. By interpreting feed-forward networks as single-iteration approximations of inference in our model, we provide both a novel theoretical perspective for understanding them and a practical technique for constraining predictions with prior knowledge. Experimentally, we demonstrate performance improvements on a variety of tasks, including single-image depth prediction with sparse output constraints.
更多查看译文
关键词
Component analysis, Deep learning, Constraints
AI 理解论文
溯源树
样例
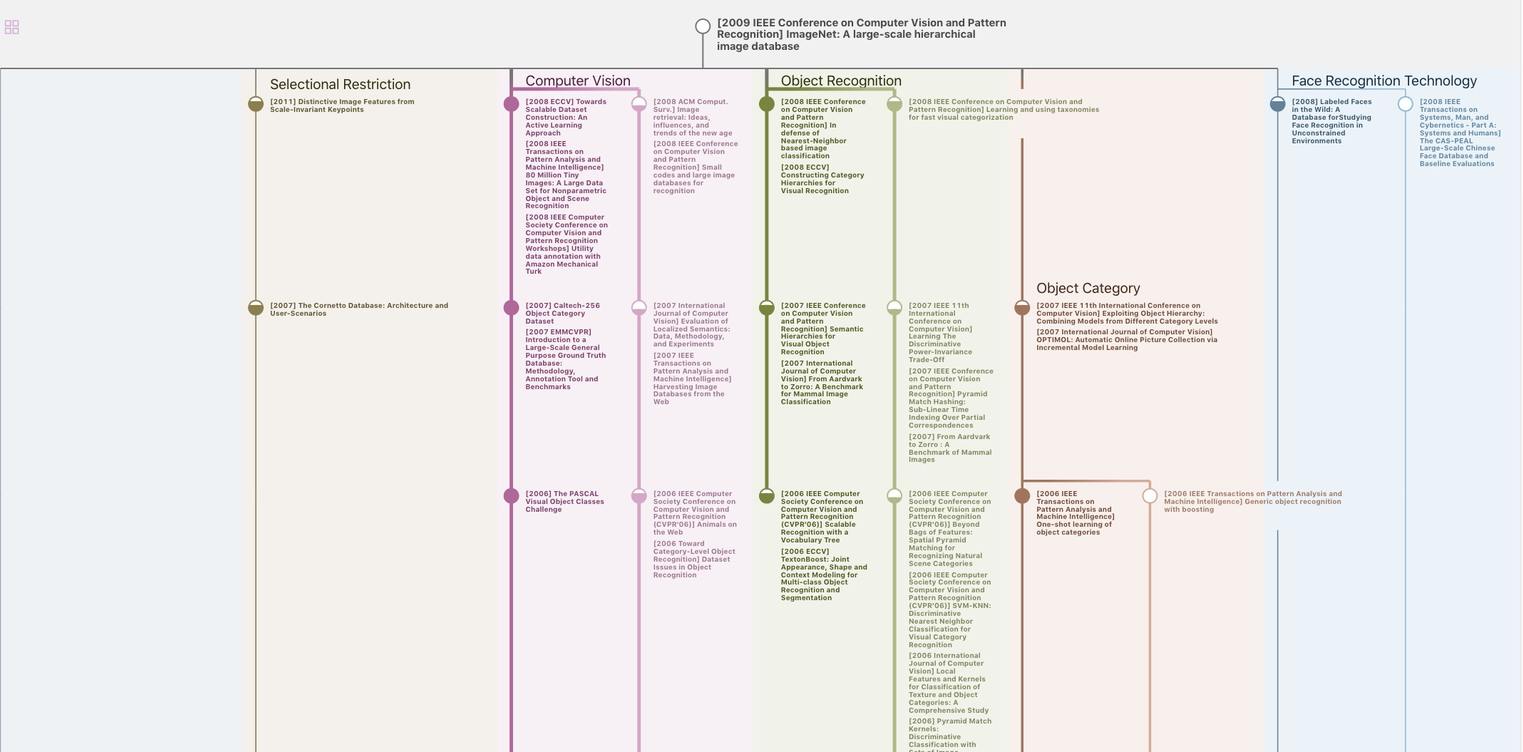
生成溯源树,研究论文发展脉络
Chat Paper
正在生成论文摘要