Learning to Search via Self-Imitation.
CoRR(2018)
摘要
We study the problem of learning a good local search policy for solving combinatorial optimization problems such as mixed integer linear programs. To do so, we propose the self-imitation learning setting, which builds upon imitation learning in two ways. First, self-imitation uses feedback provided by retroactive analysis of demonstrated search traces. Second, the policy can learn from its own decisions and mistakes without requiring repeated feedback from an external expert. Combined, these two properties allow our approach to iteratively scale up to larger problem sizes than the initial problem size for which expert demonstrations were provided. We showcase the effectiveness of our approach on the challenging problem of risk-aware planning.
更多查看译文
关键词
search,learning,self-imitation
AI 理解论文
溯源树
样例
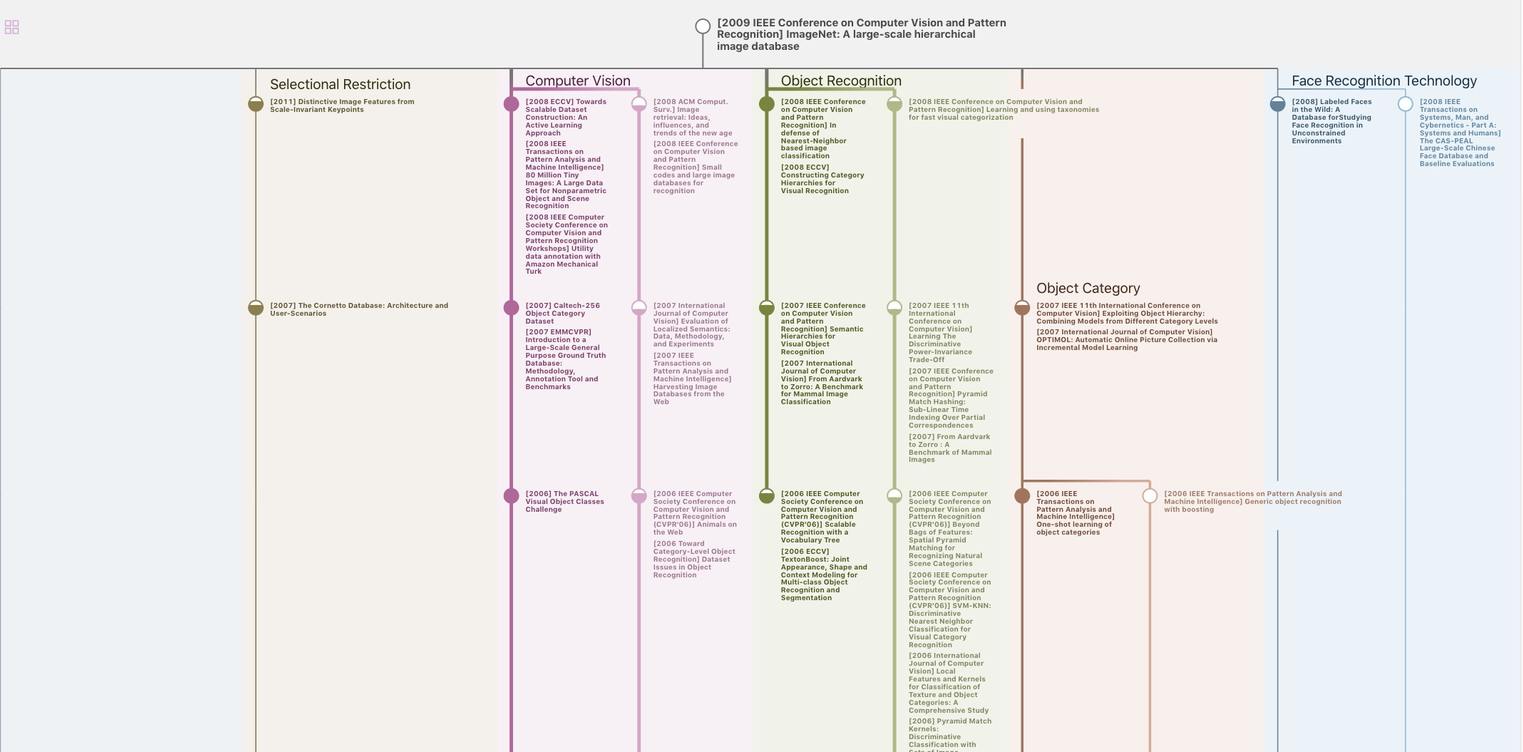
生成溯源树,研究论文发展脉络
Chat Paper
正在生成论文摘要