DORA The Explorer: Directed Outreaching Reinforcement Action-Selection.
ICLR(2018)
摘要
Exploration is a fundamental aspect of Reinforcement Learning. Two key challenges are how to focus exploration on more valuable states, and how to direct exploration toward gaining new world knowledge. Visit-counters have been proven useful both in practice and in theory for directed exploration. However, a major limitation of counters is their locality, considering only the immediate one step exploration value. While there are a few model-based solutions to this difficulty, a model-free approach is still missing. We propose $E$-values, a generalization of counters that can be used to evaluate the propagating exploratory value over state-action trajectories. We compare our approach to commonly used RL techniques, and show that using $E$-value improves learning and performance over traditional counters. We also show how our method can be implemented with function approximation to learn continuous MDPs.
更多查看译文
AI 理解论文
溯源树
样例
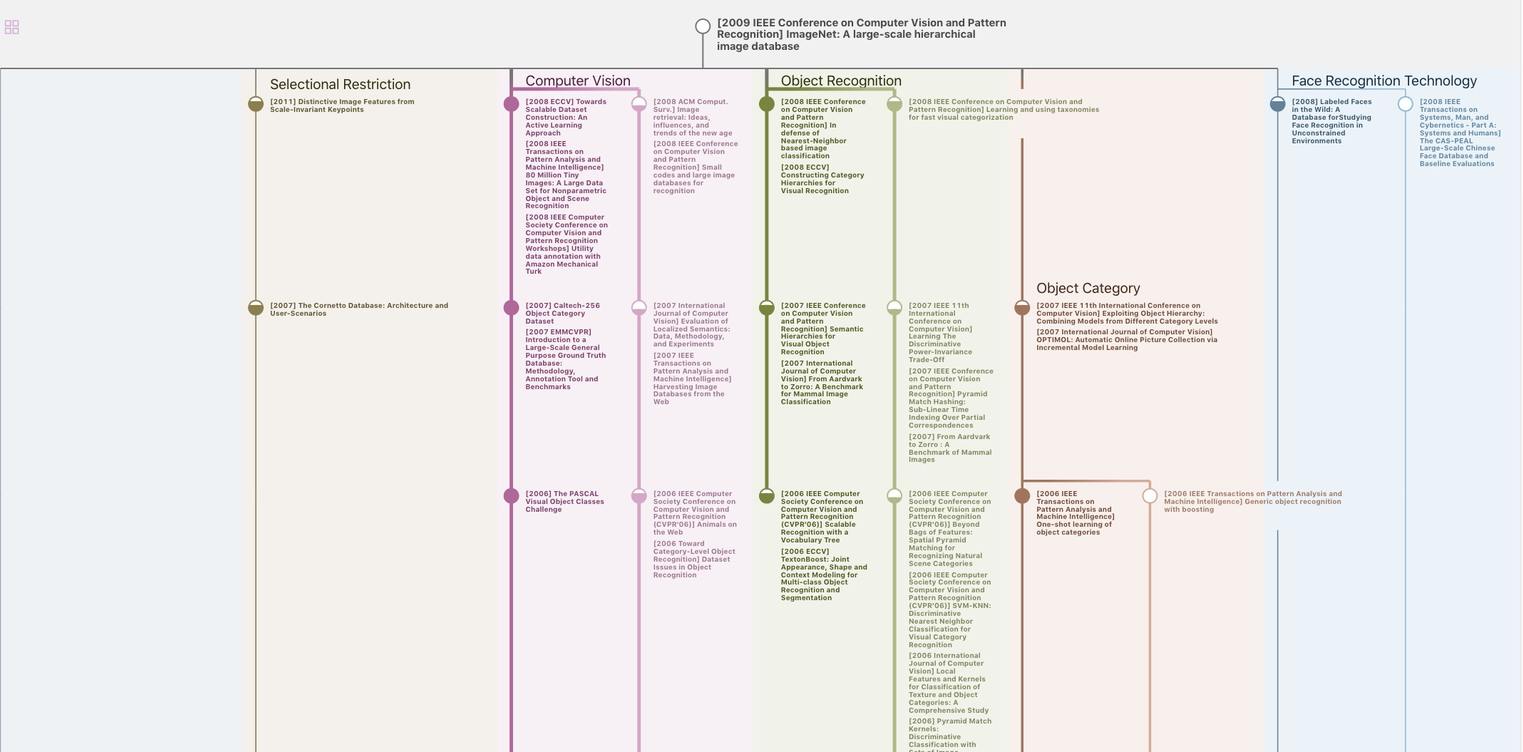
生成溯源树,研究论文发展脉络
Chat Paper
正在生成论文摘要