Learning with Correntropy-induced Losses for Regression with Mixture of Symmetric Stable Noise.
Applied and Computational Harmonic Analysis(2020)
摘要
In recent years, correntropy and its applications in machine learning have been drawing continuous attention owing to its merits in dealing with non-Gaussian noise and outliers. However, theoretical understanding of correntropy, especially in the learning theory context, is still limited. In this study, we investigate correntropy based regression in the presence of non-Gaussian noise or outliers within the statistical learning framework. Motivated by the practical way of generating non-Gaussian noise or outliers, we introduce mixture of symmetric stable noise, which include Gaussian noise, Cauchy noise, and their mixture as special cases, to model non-Gaussian noise or outliers. We demonstrate that under the mixture of symmetric stable noise assumption, correntropy based regression can learn the conditional mean function or the conditional median function well without resorting to the finite-variance or even the finite first-order moment condition on the noise. In particular, for the above two cases, we establish asymptotic optimal learning rates for correntropy based regression estimators that are asymptotically of type O(n−1). These results justify the effectiveness of the correntropy based regression estimators in dealing with outliers as well as non-Gaussian noise. We believe that the present study makes a step forward towards understanding correntropy based regression from a statistical learning viewpoint, and may also shed some light on robust statistical learning for regression.
更多查看译文
AI 理解论文
溯源树
样例
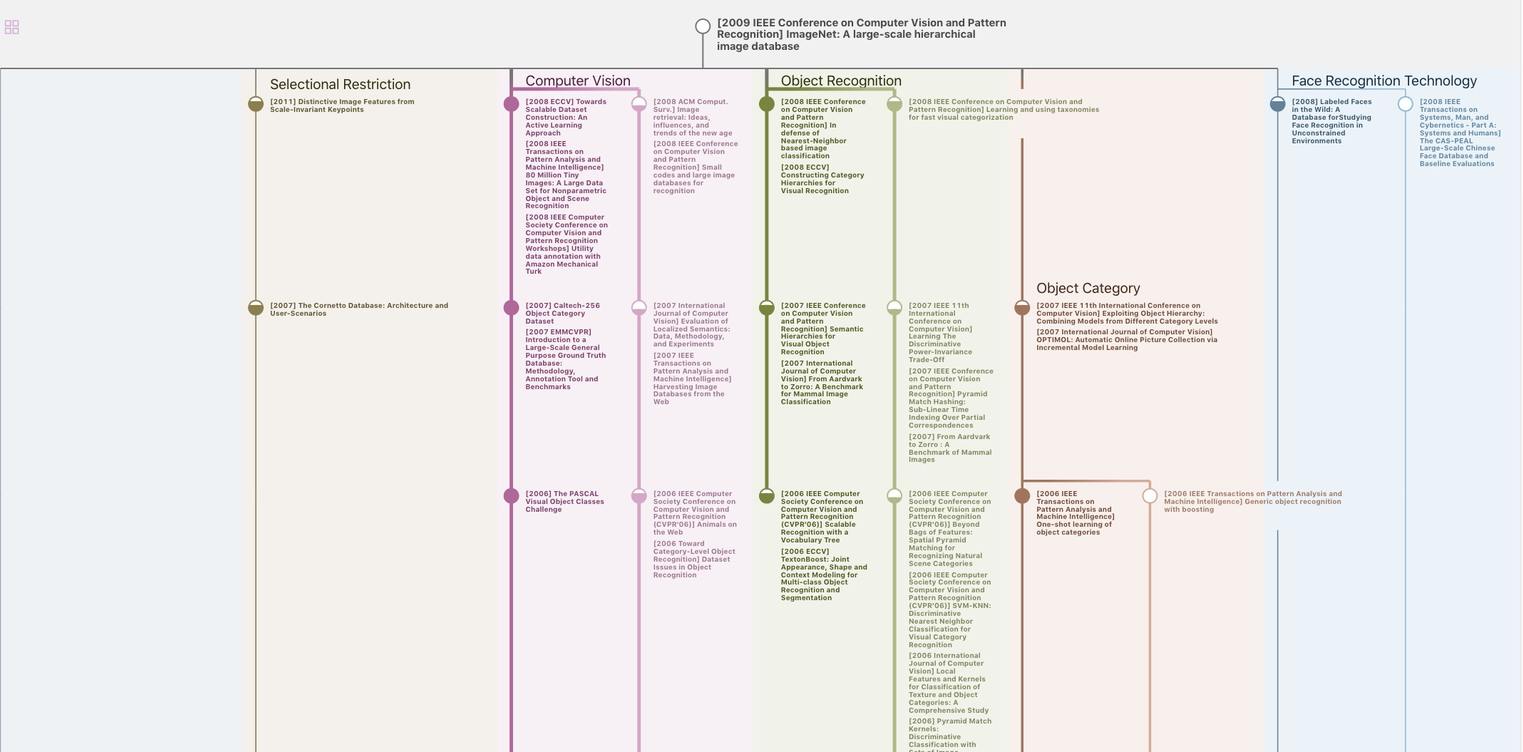
生成溯源树,研究论文发展脉络
Chat Paper
正在生成论文摘要