Faster Anomaly Detection via Matrix Sketching.
arXiv: Learning(2018)
摘要
We present efficient streaming algorithms to compute two commonly used anomaly measures: the rank-$k$ leverage scores (aka Mahalanobis distance) and the rank-$k$ projection distance, in the row-streaming model. We show that commonly used matrix sketching techniques such as the Frequent Directions sketch and random projections can be used to approximate these measures. Our main technical contribution is to prove matrix perturbation inequalities for operators arising in the computation of these measures.
更多查看译文
关键词
faster anomaly detection,matrix sketching,anomaly detection
AI 理解论文
溯源树
样例
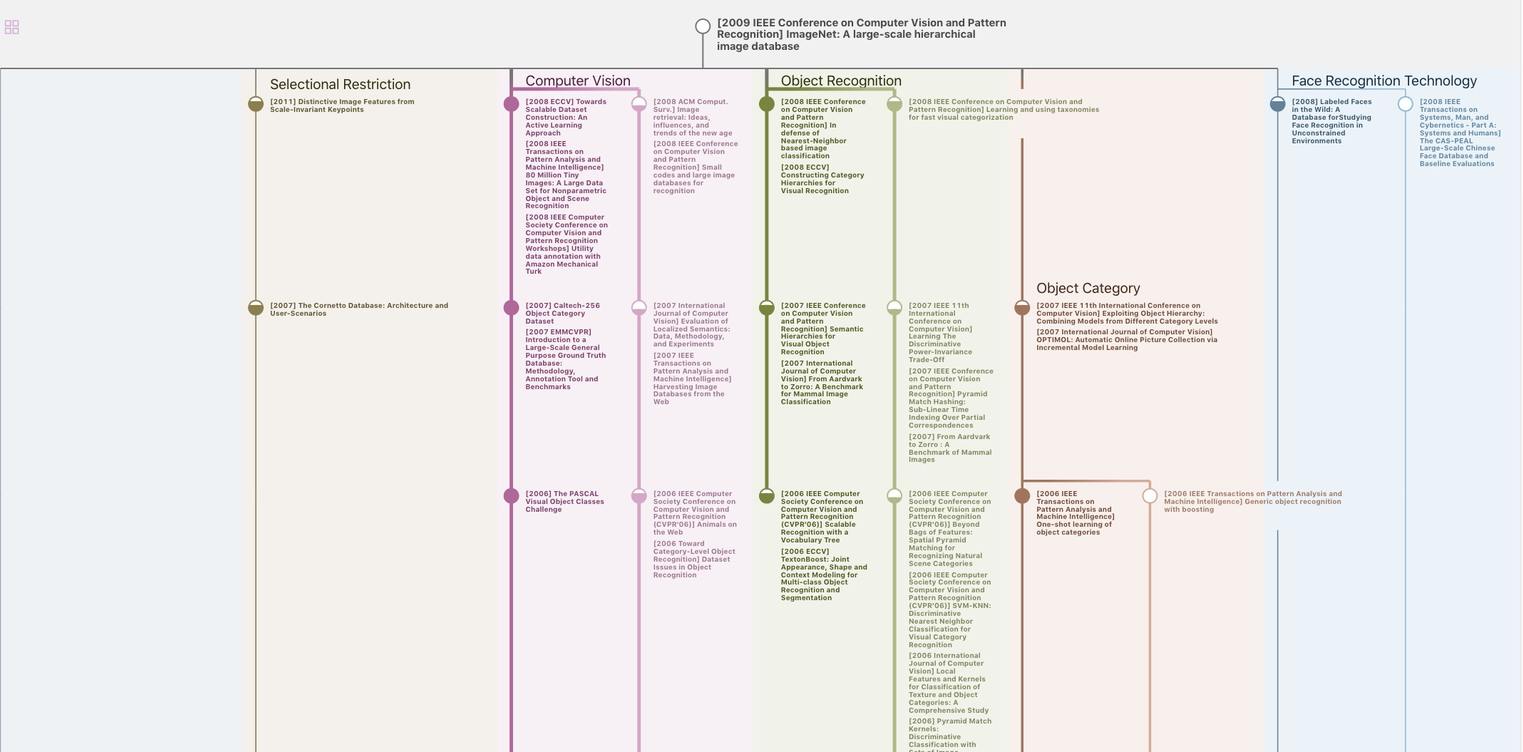
生成溯源树,研究论文发展脉络
Chat Paper
正在生成论文摘要