Localizing Faults in Cloud Systems
2018 IEEE 11th International Conference on Software Testing, Verification and Validation (ICST)(2018)
摘要
By leveraging large clusters of commodity hardware, the Cloud offers great opportunities to optimize the operative costs of software systems, but impacts significantly on the reliability of software applications. The lack of control of applications over Cloud execution environments largely limits the applicability of state-of-the-art approaches that address reliability issues by relying on heavyweight training with injected faults. In this paper, we propose LOUD, a lightweight fault localization approach that relies on positive training only, and can thus operate within the constraints of Cloud systems. LOUD relies on machine learning and graph theory. It trains machine learning models with correct executions only, and compensates the inaccuracy that derives from training with positive samples, by elaborating the outcome of machine learning techniques with graph theory algorithms. The experimental results reported in this paper confirm that LOUD can localize faults with high precision, by relying only on a lightweight positive training.
更多查看译文
关键词
fault localization,cloud
AI 理解论文
溯源树
样例
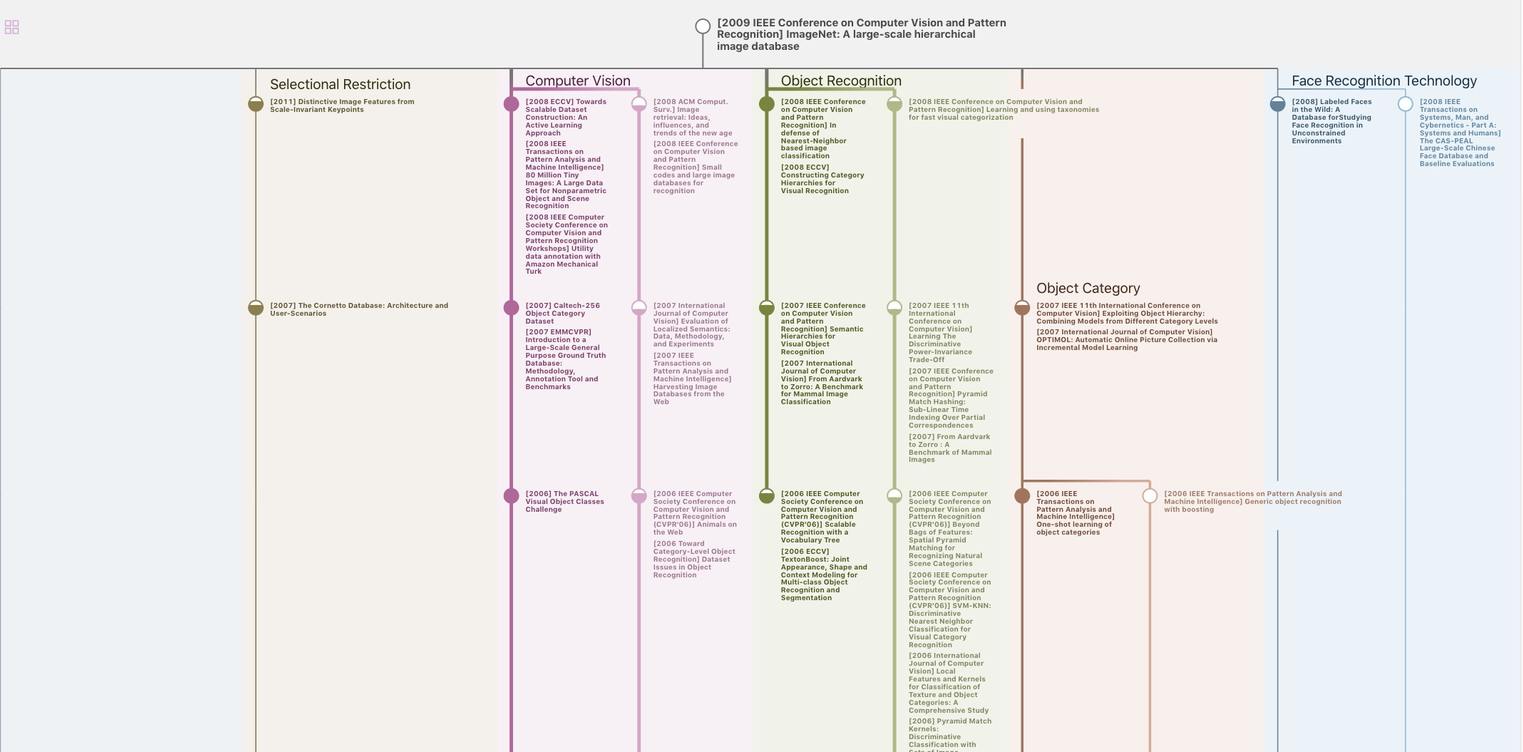
生成溯源树,研究论文发展脉络
Chat Paper
正在生成论文摘要