Multiple Models for Recommending Temporal Aspects of Entities
The Semantic Web: 15th International Conference, ESWC 2018, Heraklion, Crete, Greece, June 3–7, 2018, Proceedings(2018)
摘要
Entity aspect recommendation is an emerging task in semantic search that helps users discover serendipitous and prominent information with respect to an entity, of which salience (e.g., popularity) is the most important factor in previous work. However, entity aspects are temporally dynamic and often driven by events happening over time. For such cases, aspect suggestion based solely on salience features can give unsatisfactory results, for two reasons. First, salience is often accumulated over a long time period and does not account for recency. Second, many aspects related to an event entity are strongly time-dependent. In this paper, we study the task of temporal aspect recommendation for a given entity, which aims at recommending the most relevant aspects and takes into account time in order to improve search experience. We propose a novel event-centric ensemble ranking method that learns from multiple time and type-dependent models and dynamically trades off salience and recency characteristics. Through extensive experiments on real-world query logs, we demonstrate that our method is robust and achieves better effectiveness than competitive baselines.
更多查看译文
AI 理解论文
溯源树
样例
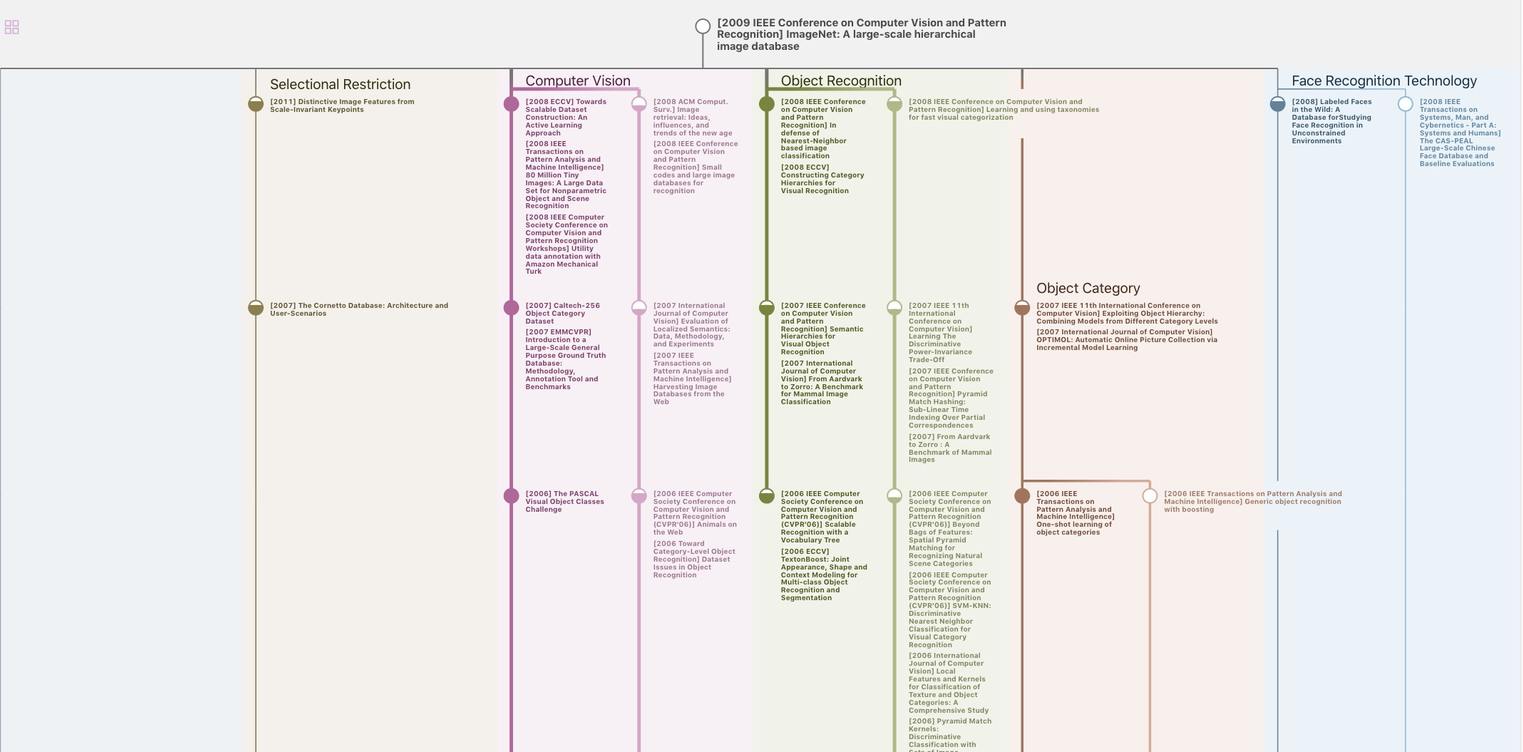
生成溯源树,研究论文发展脉络
Chat Paper
正在生成论文摘要