Gaussian and exponential lateral connectivity on distributed spiking neural network simulation
2018 26th Euromicro International Conference on Parallel, Distributed and Network-based Processing (PDP)(2019)
摘要
We measured the impact of long-range exponentially decaying intra-areal lateral connectivity on the scaling and memory occupation of a distributed spiking neural network simulator compared to that of short-range Gaussian decays. While previous studies adopted short-range connectivity, recent experimental neurosciences studies are pointing out the role of longer-range intra-areal connectivity with implications on neural simulation platforms. Two-dimensional grids of cortical columns composed by up to 11 M point-like spiking neurons with spike frequency adaption were connected by up to 30 G synapses using short- and long-range connectivity models. The MPI processes composing the distributed simulator were run on up to 1024 hardware cores, hosted on a 64 nodes server platform. The hardware platform was a cluster of IBM NX360 M5 16-core compute nodes, each one containing two Intel Xeon Haswell 8-core E5-2630 v3 processors, with a clock of 2.40 G Hz, interconnected through an InfiniBand network, equipped with 4x QDR switches.
更多查看译文
关键词
cortical simulation,distributed computing,spiking neural network,lateral synaptic connectivity,hardware/software co-design
AI 理解论文
溯源树
样例
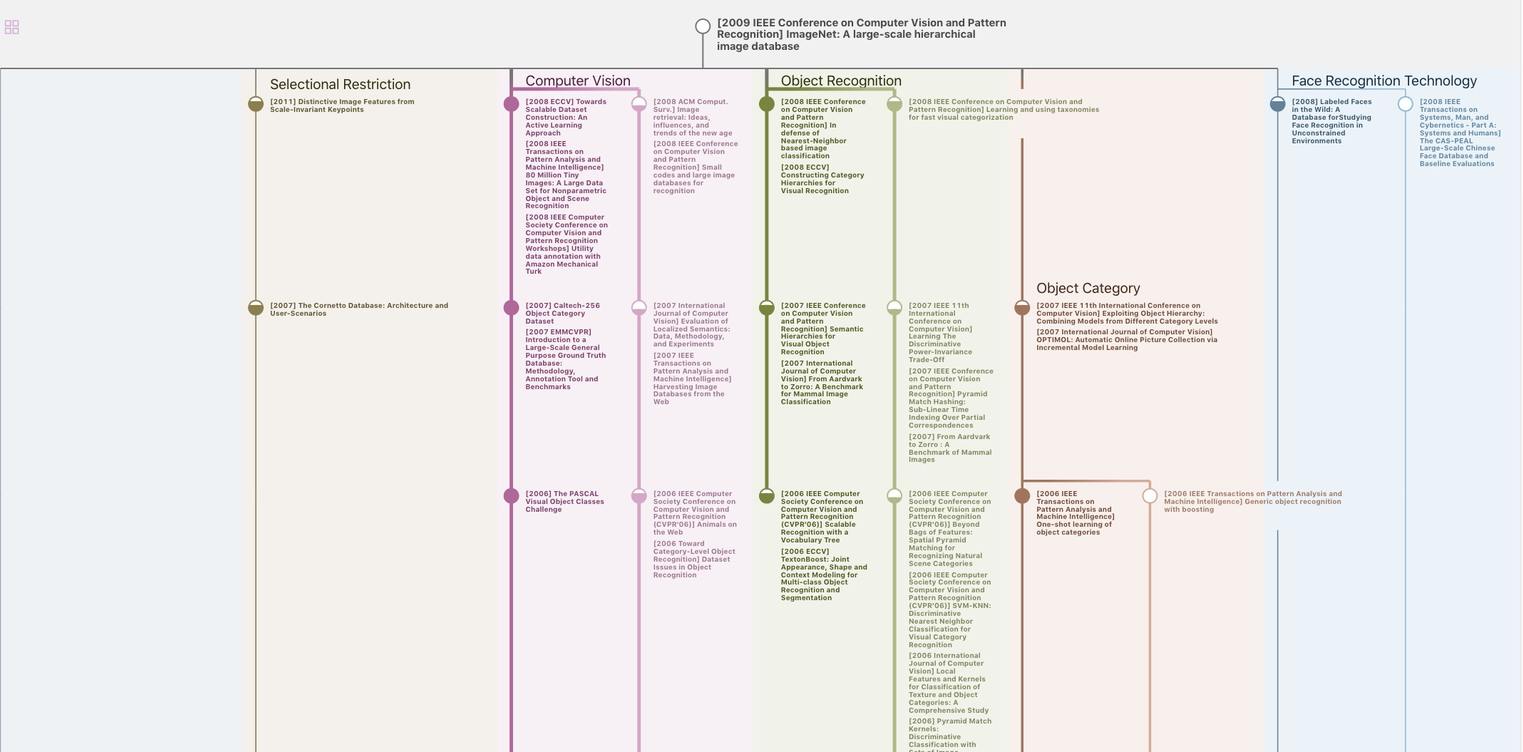
生成溯源树,研究论文发展脉络
Chat Paper
正在生成论文摘要