Bio-inspired learning approach for electronic nose
Computing(2018)
摘要
The high sensitivity, stability, selectivity and adaptivity of mailman olfactory system is a result of a large number of olfactory receptors feeding into extensive layers of neural processing units. Olfactory receptor cells (ORC) contribute significantly in the sense of smells. Bloodhounds have 4 billion ORC making them ideal for tracking while human has about 30 million ORC. E-nose stability, sensitivity and selectivity have been a challenging task. We hypothesize that appropriate signal processing with an increased number of sensory receptors can significantly improve odour recognition in e-nose. Adding physical receptors to e-nose is costly and can increase system complexity. Therefore, we propose an artificial olfactory receptor cells model inspired by neural circuits of the vertebrate olfactory system to improve e-nose performance. Secondly, we introduce and adaptation layer to cope with drift and unknown changes. The major layers in our model are the sensory transduction layer, sensory adaptation layer, artificial olfactory receptors layer and artificial olfactory cortex layer. Each layer in the proposed system is biologically inspired by the mammalian olfactory system. The experiments are executed using chemo-sensory arrays data generated over a 3-year period. The proposed model resulted in a better performance and stability compared to other models. However, e-nose stability, selectivity and sensitivity issues remain unsolved problems. Our paper provides a new approach to improve e-nose pattern recognition over a long period of time.
更多查看译文
关键词
E-nose,Receptors,Drift,Biological,Neural,Noise,Classification,Adaptive,Sensors,Chemical,68
AI 理解论文
溯源树
样例
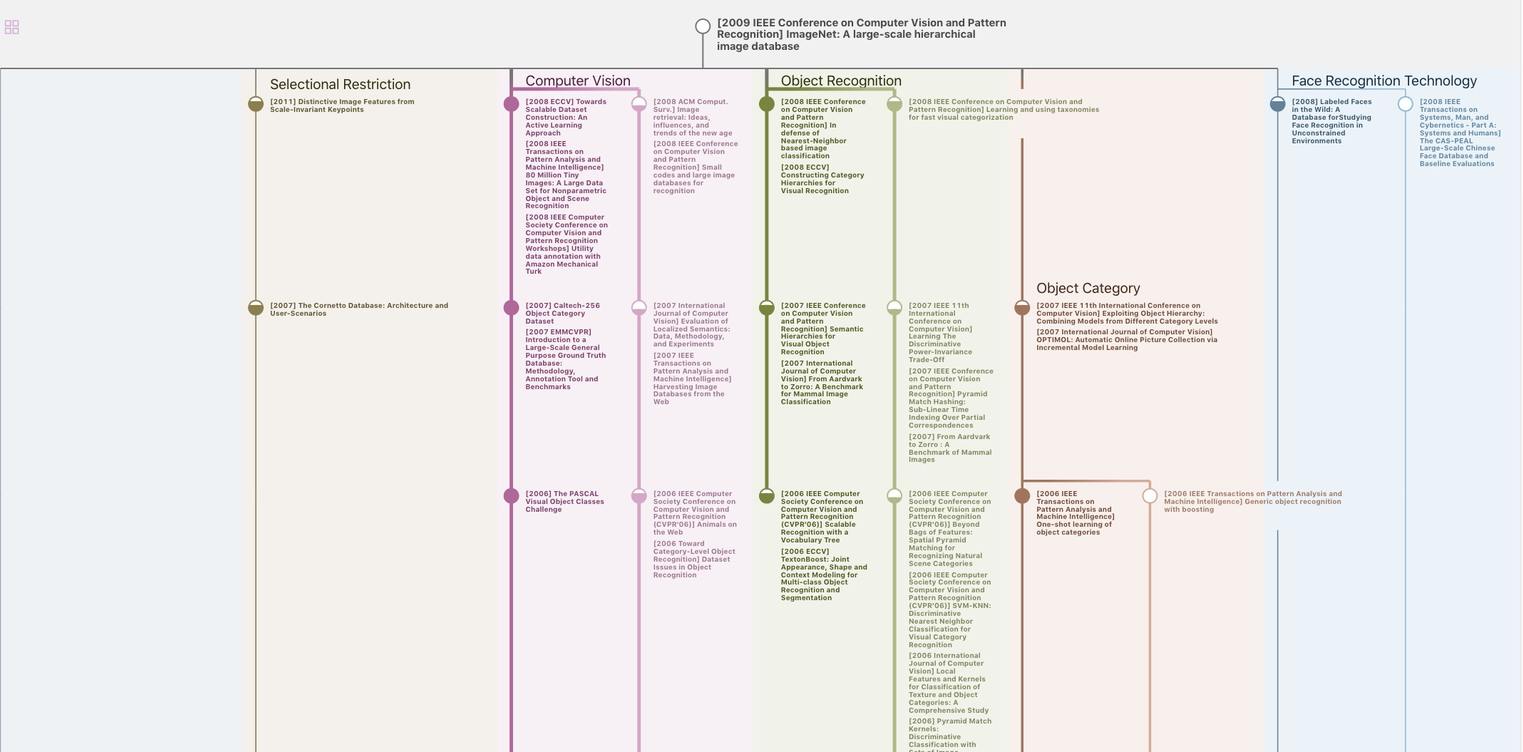
生成溯源树,研究论文发展脉络
Chat Paper
正在生成论文摘要