Enhancing social network privacy with accumulated non-zero prior knowledge
Information Sciences(2018)
摘要
With the fast development of social networks, privacy protection on social network data has already attracted more and more research attention. If an adversary has collected enough information by intentionally querying a social network, the privacy of social network data might be successfully inferred based on the previous query results, which is seldom investigated by most existing approaches. In this paper, we first formulate the correlated query results as the non-zero prior knowledge, then propose a novel differential privacy approach to enhance privacy of social network data from being inferred. The corresponding ϵ-difference is re-formulated as well as the calculation of the sensitivity between two “neighboring” social graphs. Particularly, the calculation of sensitivity is proposed for three widely adopted queries on social networks, i.e., node degree distribution query, cut set query and the shortest path query. To evaluate our approach, rigorous experiments have been performed on three real world data sets. The promising experimental results have demonstrated that the proposed approach is superior to the state-of-the-art privacy protection approaches with respect to data privacy and data utility.
更多查看译文
关键词
Differential privacy,Social network analysis,Privacy protection,Privacy preserving data mining
AI 理解论文
溯源树
样例
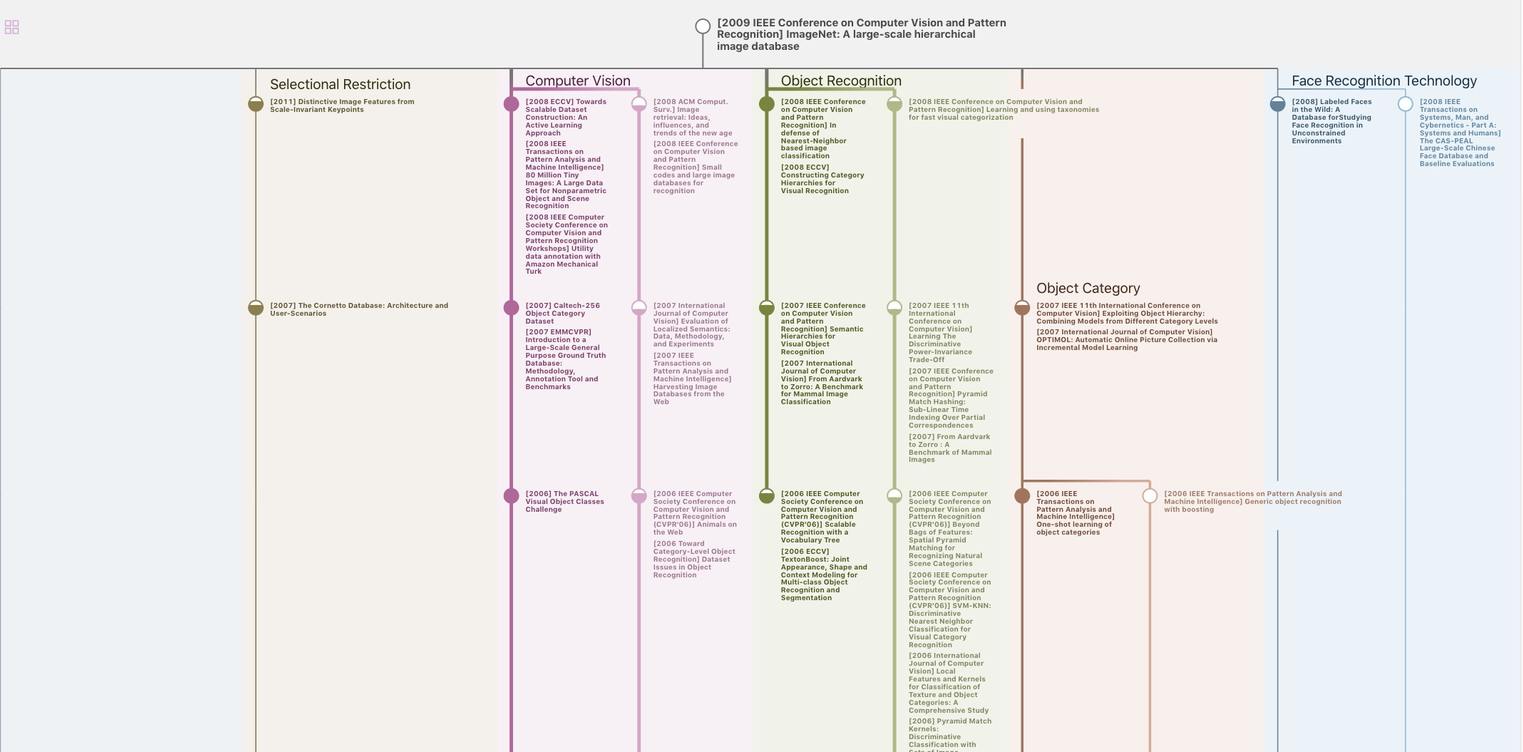
生成溯源树,研究论文发展脉络
Chat Paper
正在生成论文摘要