A Sparse Auto Encoder Deep Process Neural Network Model and its Application
INTERNATIONAL JOURNAL OF COMPUTATIONAL INTELLIGENCE SYSTEMS(2017)
摘要
Aiming at the problem of time-varying signal pattern classification, a sparse auto-encoder deep process neural network (SAE-DPNN) is proposed. The input of SAE-DPNN is time-varying process signal and the output is pattern category. It combines the time-varying signal classification method of process neural network (PNN) and the data feature extraction and hierarchical sparse representation mechanism of sparse automatic encoder (SAE). Based on the feedforward PNN model, SAE-DPNN is constructed by stacking the process neurons, SAE network and softmax classifier. It can maintain the time-sequence and structure of the input signal, express and synthesize the process distribution characteristics of multidimensional time-varying signals and their combinations. SAE-DPNN improves the identification of complex features and distinguishes between different types of signals, realizes the direct classification of time-varying signals. In this paper, the feature extraction and representation mechanism of time-varying signal in SAE-DPNN are analyzed, and a specific learning algorithm is given. The experimental results verify the effectiveness of the model and algorithm.
更多查看译文
关键词
time-varying signal classification,process neural network,deep learning,SAE,training algorithm
AI 理解论文
溯源树
样例
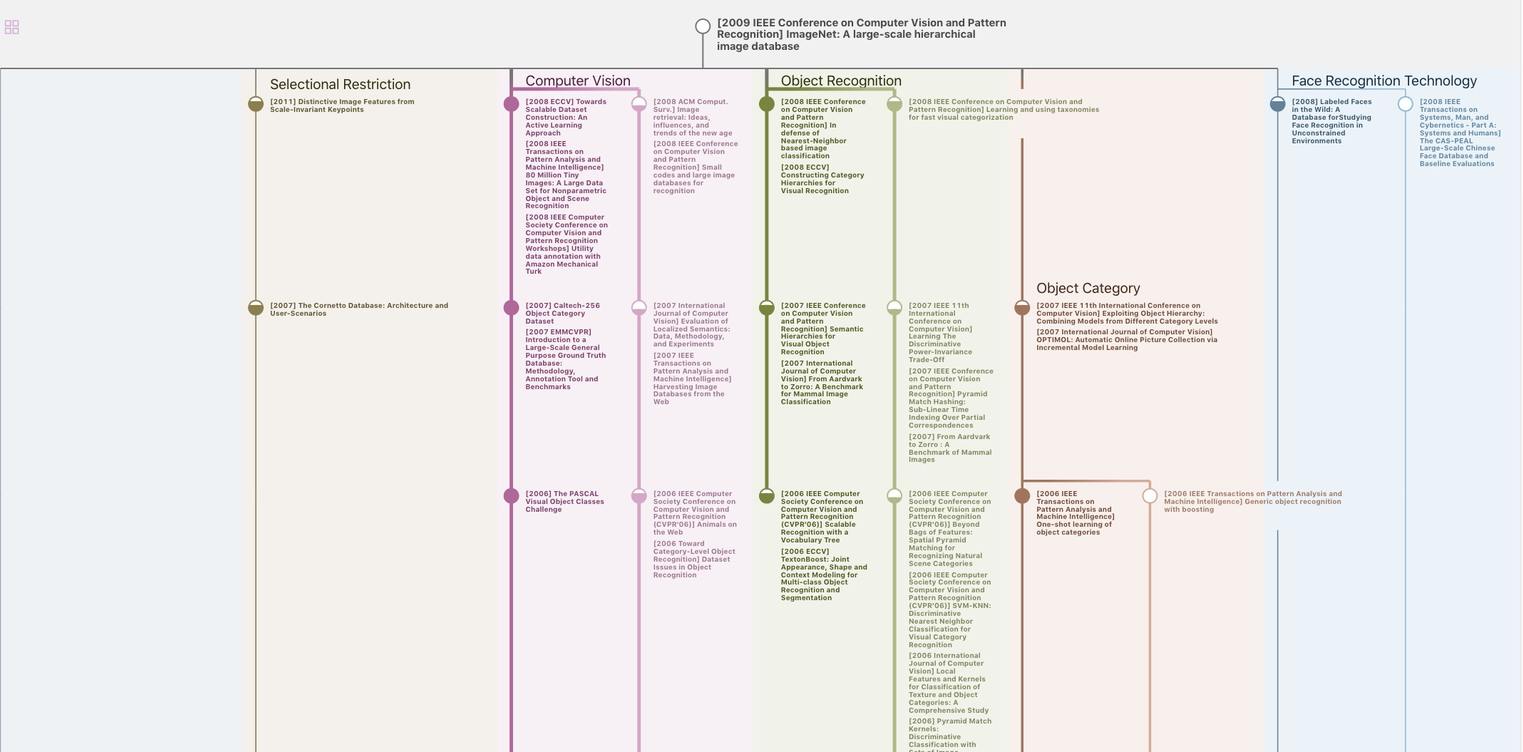
生成溯源树,研究论文发展脉络
Chat Paper
正在生成论文摘要