Coupled Low Rank Approximation for Collaborative Filtering in Social Networks.
IEEE ACCESS(2018)
摘要
Recommending items and friends are equally important in social recommendation applications. Current works usually consider them as two independent tasks and address them separately. This paper presents a new method to collaboratively perform the two tasks, in which three types of sparse data, ratings, trust, and distrust, are complemented with each other by a coupled low-rank approximation model. The data sparsity problems, which greatly decline prediction quality in respective tasks, are well mitigated in this way. The recovery conditions regarding the low-rank approximation are provided. Empirical studies on real data also support our theoretical models and analysis. To the best of our knowledge, this is the first attempt to raise and address such a problem.
更多查看译文
关键词
Recommendation,collaborative filtering,low-rank approximation,matrix factorization
AI 理解论文
溯源树
样例
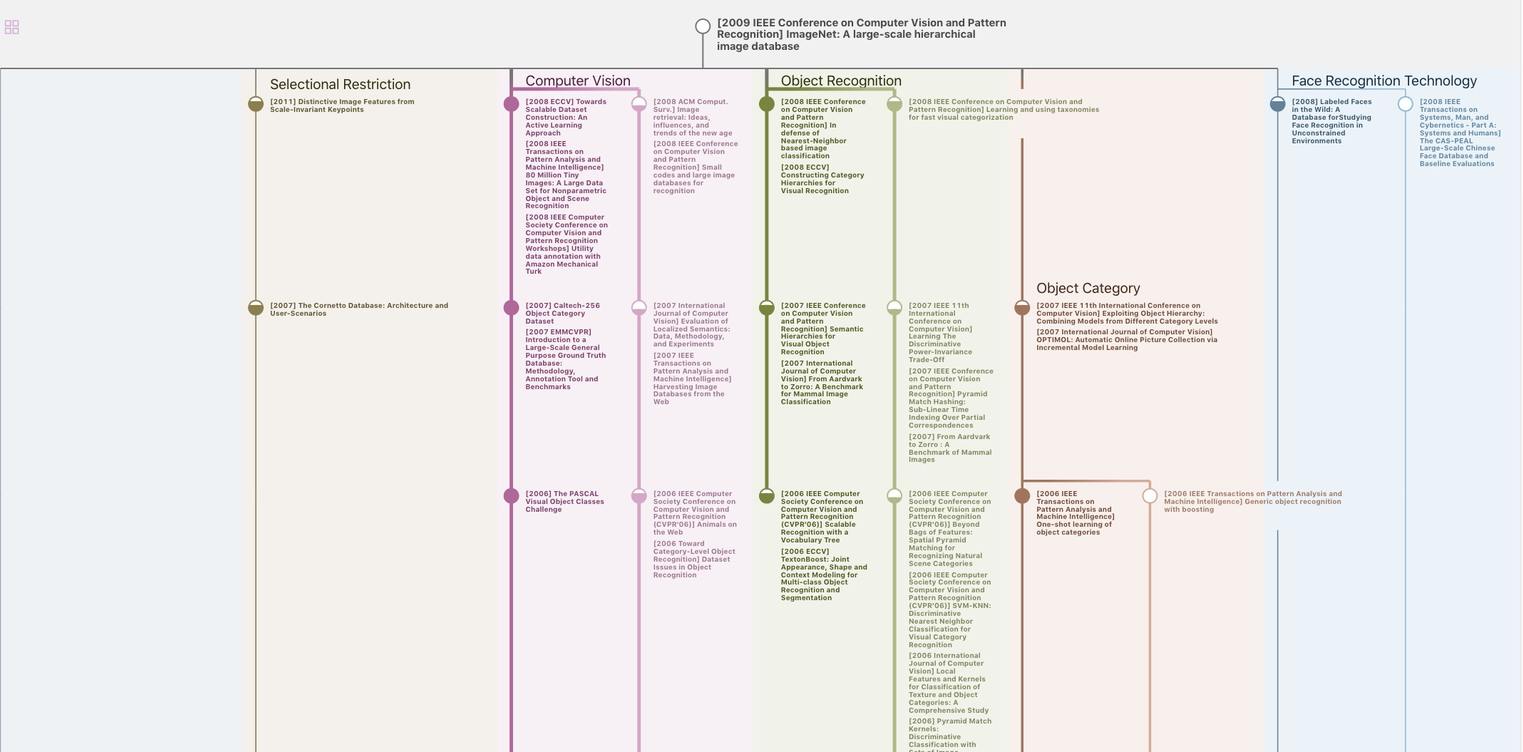
生成溯源树,研究论文发展脉络
Chat Paper
正在生成论文摘要