Hyperspectral and Multispectral Image Fusion Based on Low Rank Constrained Gaussian Mixture Model.
IEEE ACCESS(2018)
摘要
This paper attempts to fuse a multispectral image and an auxiliary hyperspectral image (HSI) with no requirement of image registration. Most previous studies solve this problem with sparsity-based methods. However, in this paper, a novel fusion framework is developed based on a Gaussian mixture model (GMM): First, the GMM is adopted to extract the spectral information from the input HSI. Low-rank constraints are imposed on the covariance matrices of the model to solve the computational problem in the expectation-maximization approach. Second, considering the spatial self-similarity, a structure-similarity regularization term is designed to further enhance the quality of the reconstructed image. To that end, a forward-backward splitting method is adopted to cut down the computational complexity of the optimization. The proposed method does not require two well-aligned images, thus, it will not be influenced by the registration errors between two fusing images. Experimental results of a simulated data set and an actual satellite (EO-1/Hyperion/ALI) data set show that the proposed method displays a stable performance and outperforms many state-of-the-art methods with acceptable computational complexity, when registration errors are taken into consideration.
更多查看译文
关键词
Hyperspectral image fusion,Gaussian mixture model,low rank constraint,local and nonlocal similarity,registration errors
AI 理解论文
溯源树
样例
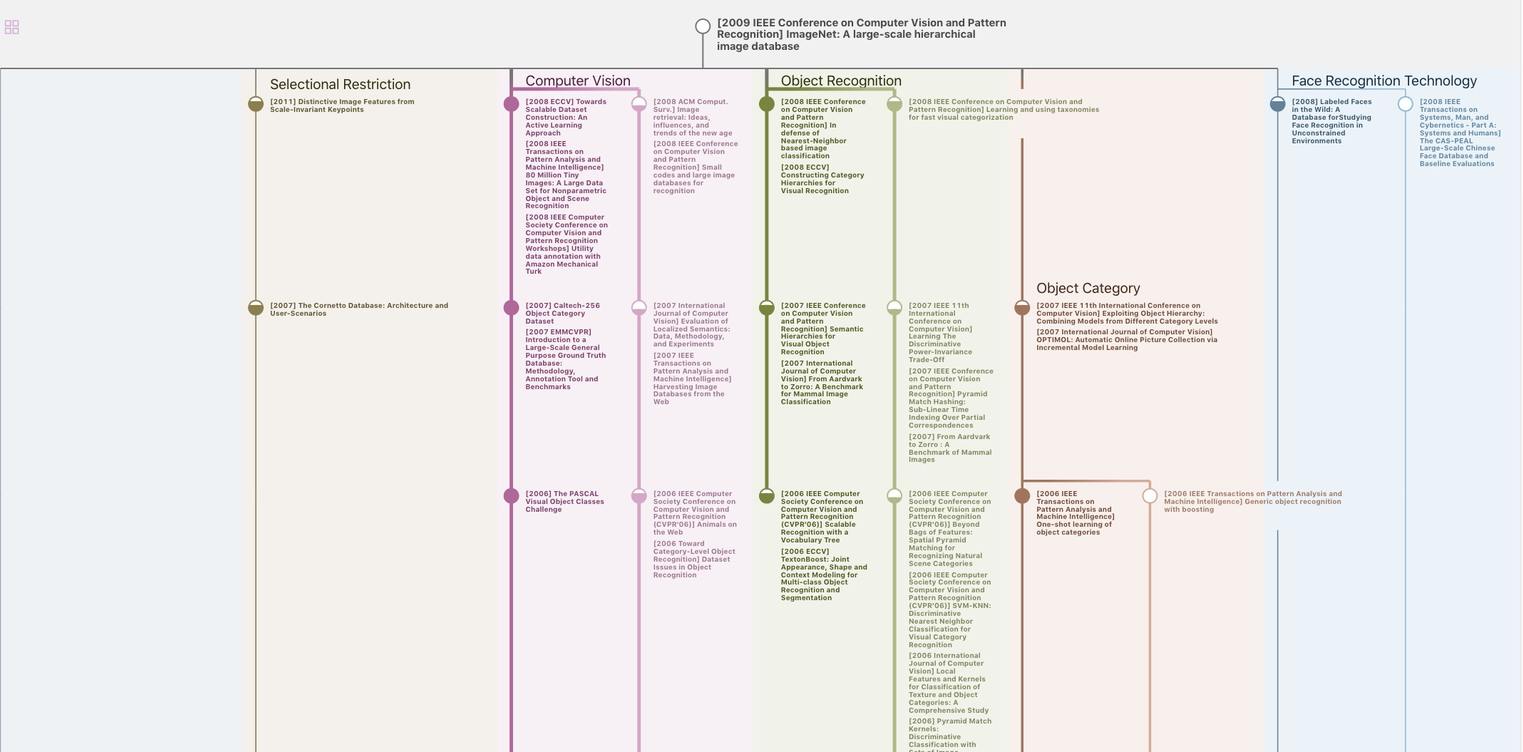
生成溯源树,研究论文发展脉络
Chat Paper
正在生成论文摘要