Clustering Big Cancer Data by Effect Sizes
2017 IEEE/ACM International Conference on Connected Health: Applications, Systems and Engineering Technologies (CHASE)(2017)
摘要
We propose an effect size based approach to compute initial dissimilarities for Ensemble Algorithm of Clustering Cancer Data (EACCD). The proposed method is applied to the colon cancer data from the Surveillance, Epidemiology, and End Results (SEER) Program of the National Cancer Institute and compared with the log-rank approach where initial dissimilarities are computed from the log-rank test statistic. The experimental results show that under the proportional hazards assumption, the effect size approach generates robust results and has a better performance than the log-rank approach.
更多查看译文
关键词
TNM,survival,colon cancer,hierarchical clustering,dendrogram,prognostic system
AI 理解论文
溯源树
样例
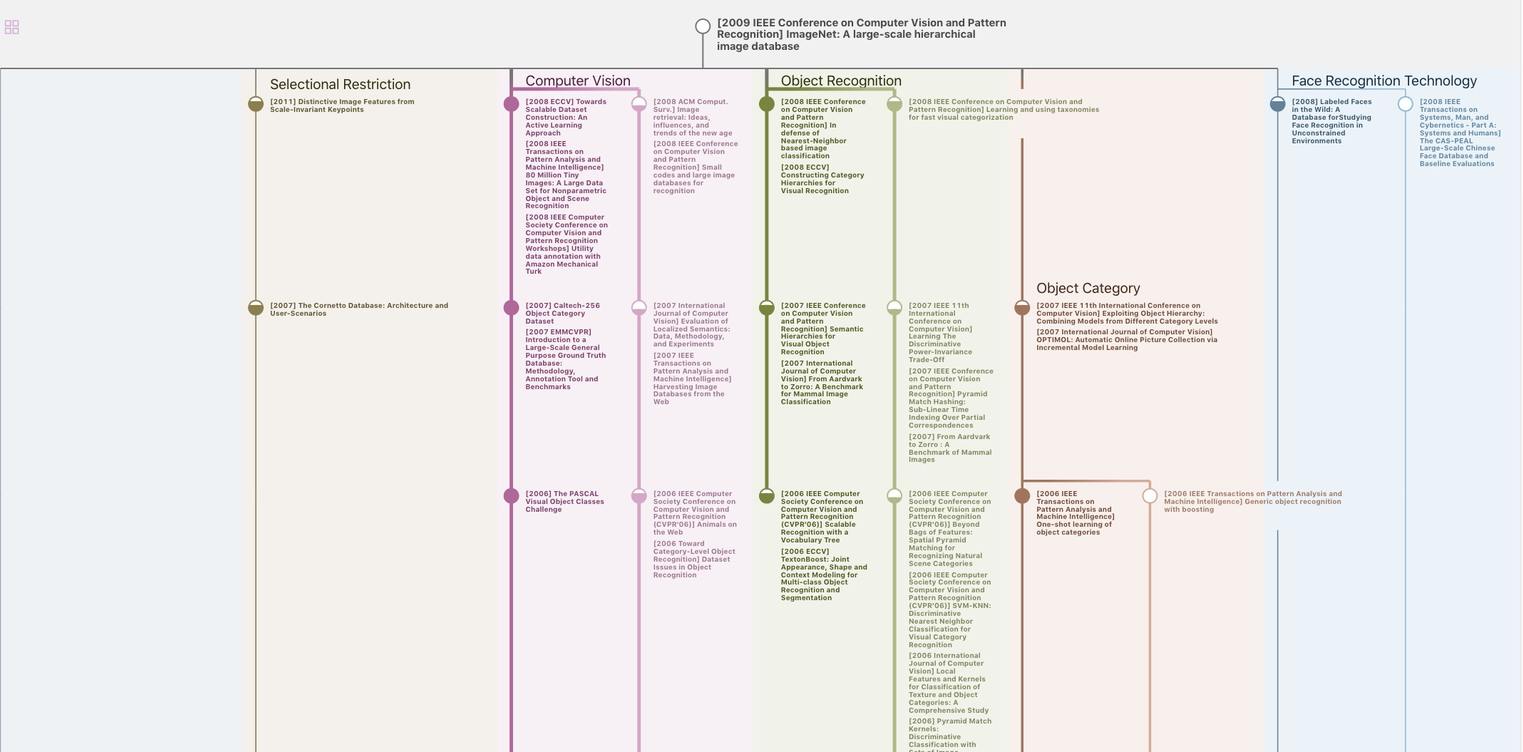
生成溯源树,研究论文发展脉络
Chat Paper
正在生成论文摘要