Selecting Relevant Educational Attributes for Predicting Students' Academic Performance.
ISDA(2017)
摘要
Predicting students’ academic performance is one of the oldest and most popular applications of educational data mining. It helps to estimate the unknown evaluation of a student’s performance. However, a huge amount of data with different formats and from multiple sources may contain a large number of features supposed as not-relevant that could influence the prediction results. The main objective of this paper is to improve the effectiveness of a predictive model for students’ academic performance. For this purpose, we propose a methodology to carry out a comparative study for evaluating the influence of feature selection techniques on the prediction of students’ academic performance. In our study, F-measure parameter is used to evaluate the effectiveness of the selected techniques. Two real data sources are used in this work, Mathematics and language courses. The outcomes are compared and discussed in order to identify the technique that has the best influence for an accurate predictive model.
更多查看译文
AI 理解论文
溯源树
样例
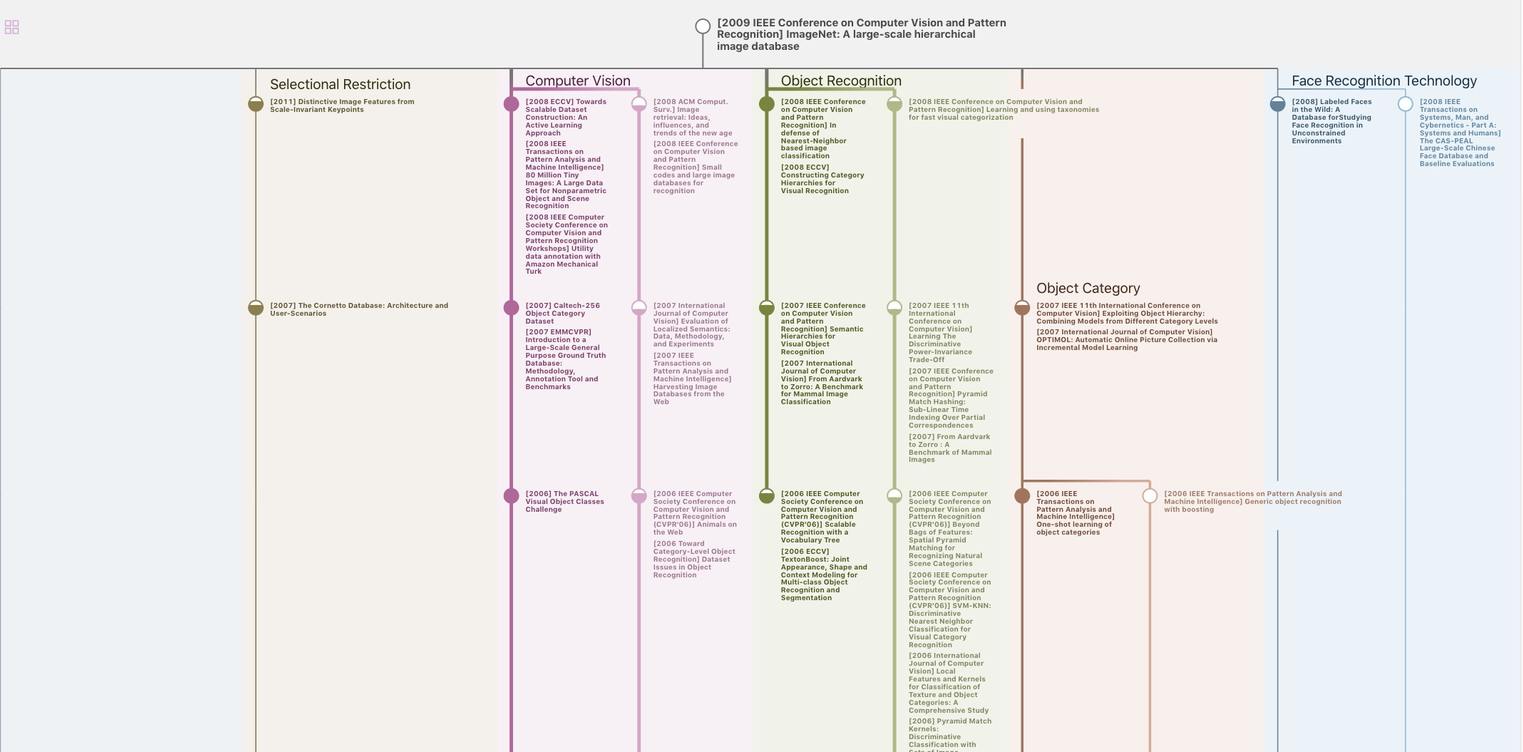
生成溯源树,研究论文发展脉络
Chat Paper
正在生成论文摘要