Verification of the Expected Answer Type for Biomedical Question Answering.
WWW '18: The Web Conference 2018 Lyon France April, 2018(2018)
摘要
Extractive Question Answering (QA) focuses on extracting precise answers from a given paragraph to questions posed in natural language. Deep learning models are widely used to address this problem and can fetch good results, provided there exists enough data for learning. Such large datasets have been released in open domain, but not in specific domains, such as the medical domain. However, the medical domain has a great amount of resources such as UMLS thesaurus, ontologies such as SNOMED CT, and tools such as Metamap etc that could be useful. In this paper, we apply transfer learning for getting a DNN baseline system on biomedical questions and we study if structured resources can help in selecting the answers based on the recognition of the Expected Answer Type (EAT), which has been proved useful in open domain QA systems. This study relies on different representations for LAT and we study if gold standard answers and answers of our model have some positive impact from the LAT.
更多查看译文
关键词
Question-Answering, Neural Network Model, Expected Answer Type
AI 理解论文
溯源树
样例
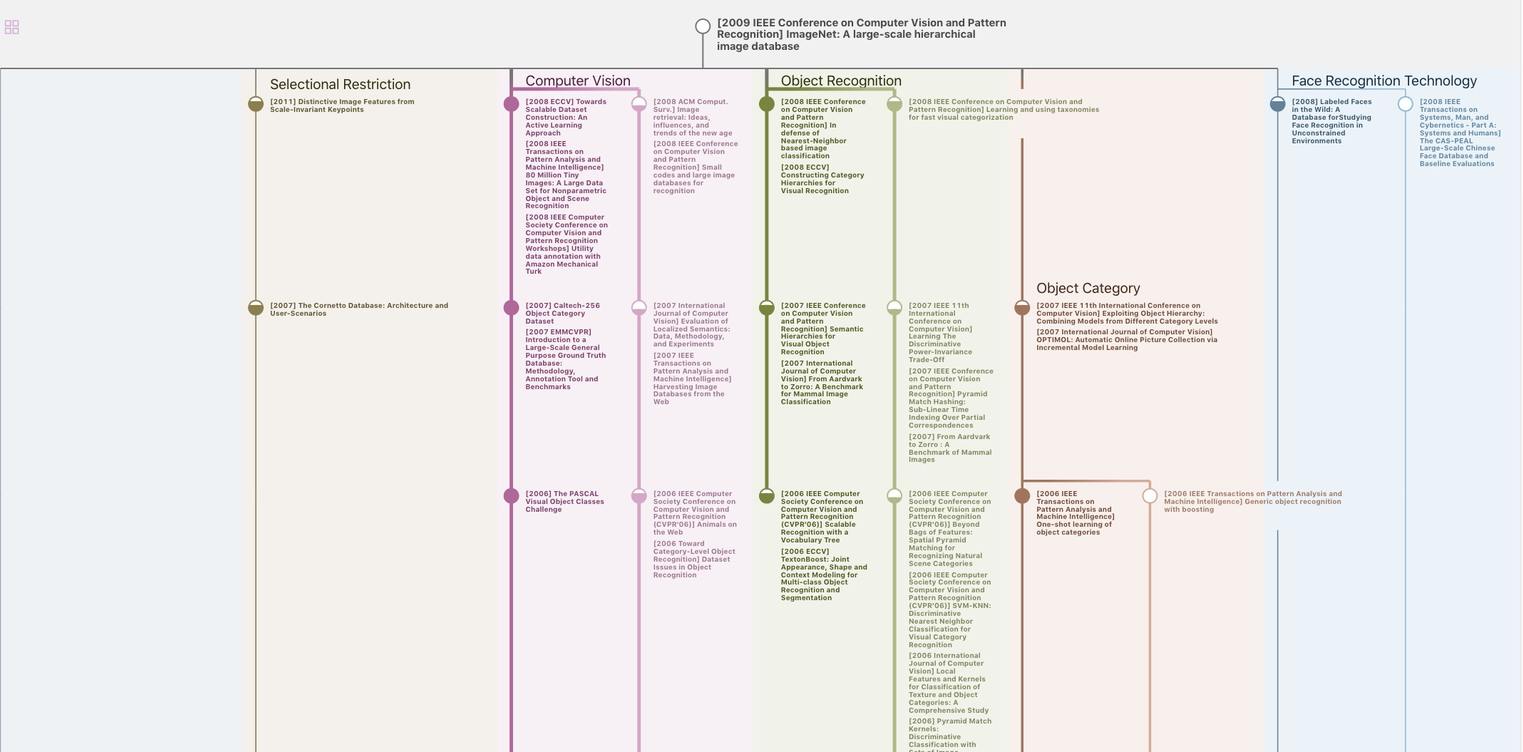
生成溯源树,研究论文发展脉络
Chat Paper
正在生成论文摘要