Deep Collective Classification in Heterogeneous Information Networks.
WWW '18: The Web Conference 2018 Lyon France April, 2018(2018)
摘要
Collective classification has attracted considerable attention in the last decade, where the labels within a group of instances are correlated and should be inferred collectively, instead of independently. Conventional approaches on collective classification mainly focus on exploiting simple relational features (such ascount andexists aggregators on neighboring nodes). However, many real-world applications involve complex dependencies among the instances, which are obscure/hidden in the networks. To capture these dependencies in collective classification, we need to go beyond simple relational features and extract deep dependencies between the instances. In this paper, we study the problem of deep collective classification inHeterogeneous Information Networks (HINs), which involves different types of autocorrelations, from simple to complex relations, among the instances. Different from conventional autocorrelations, which are given explicitly by the links in the network, complex autocorrelations are obscure/hidden in HINs, and should be inferred from existing links in a hierarchical order. This problem is highly challenging due to the multiple types of dependencies among the nodes and the complexity of the relational features. In this study, we proposed a deep convolutional collective classification method, called GraphInception to learn the deep relational features in HINs. The proposed method can automatically generate a hierarchy of relational features with different complexities. Extensive experiments on four real-world networks demonstrate that our approach can improve the collective classification performance by considering deep relational features in HINs.
更多查看译文
关键词
Collective Classification, Graph Convolution, Heterogeneous Information Networks, Graph Mining, Deep Learning
AI 理解论文
溯源树
样例
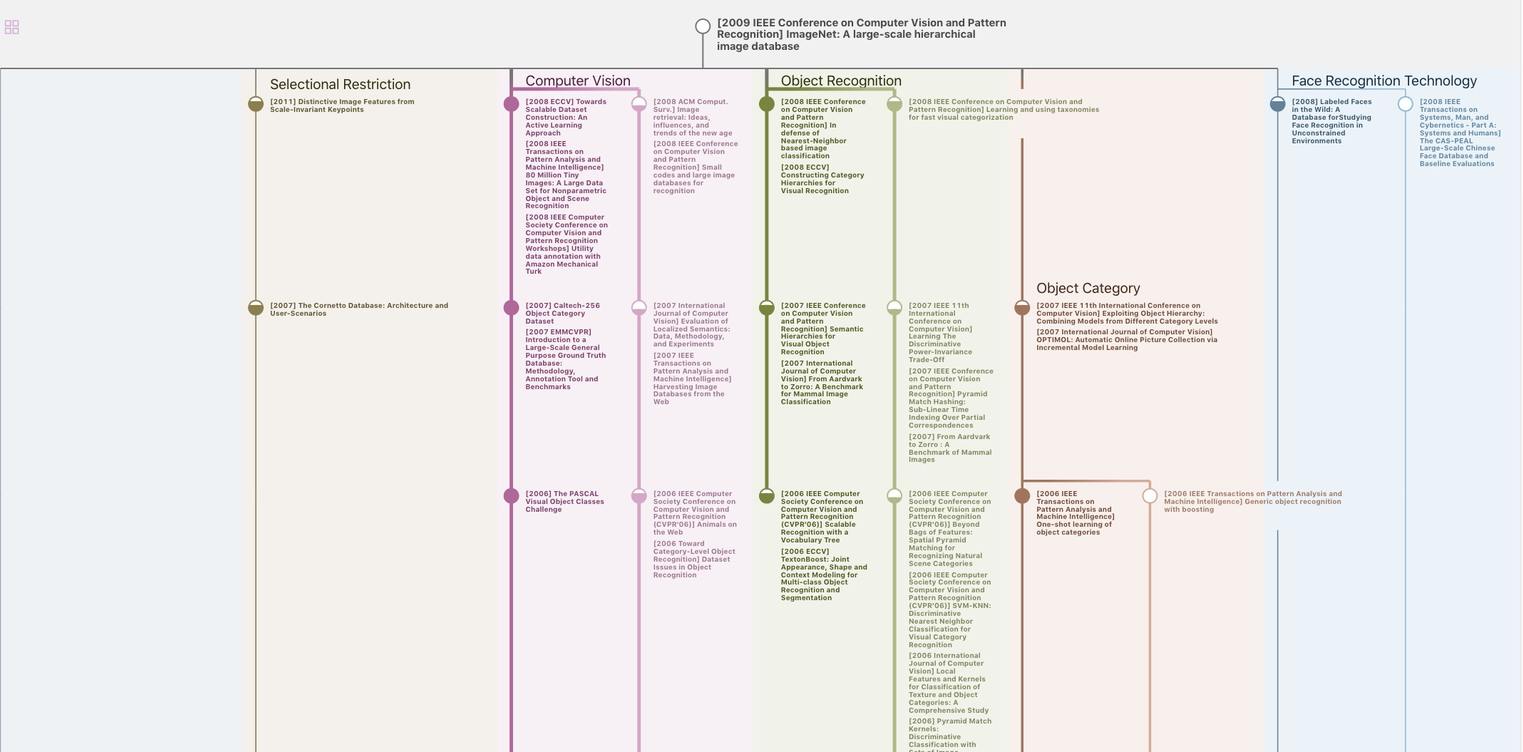
生成溯源树,研究论文发展脉络
Chat Paper
正在生成论文摘要