Practical Privacy-Preserving Friend Recommendations on Social Networks.
WWW '18: The Web Conference 2018 Lyon France April, 2018(2018)
摘要
Making friend recommendations is an important task for social networks, as having more friends typically leads to a better user experience. Most current friend recommendations systems grow the existing network at the cost of privacy. In particular, any given user's friend graph may be directly or indirectly leaked as a result of such recommendations. In many situations this is not desirable, as the friend list may reveal much about the user--from their identity to their sexual orientation and interests. In this work, we focus on the "cold start" problem of making friend recommendations for new users while raising the bar on protecting the privacy of the friend list of all users. We propose a practical friend recommendation framework, tested on the Snapchat social network, that preserves the privacy of users' friends lists with respect to brute-force attacks and scales to millions of users.
更多查看译文
AI 理解论文
溯源树
样例
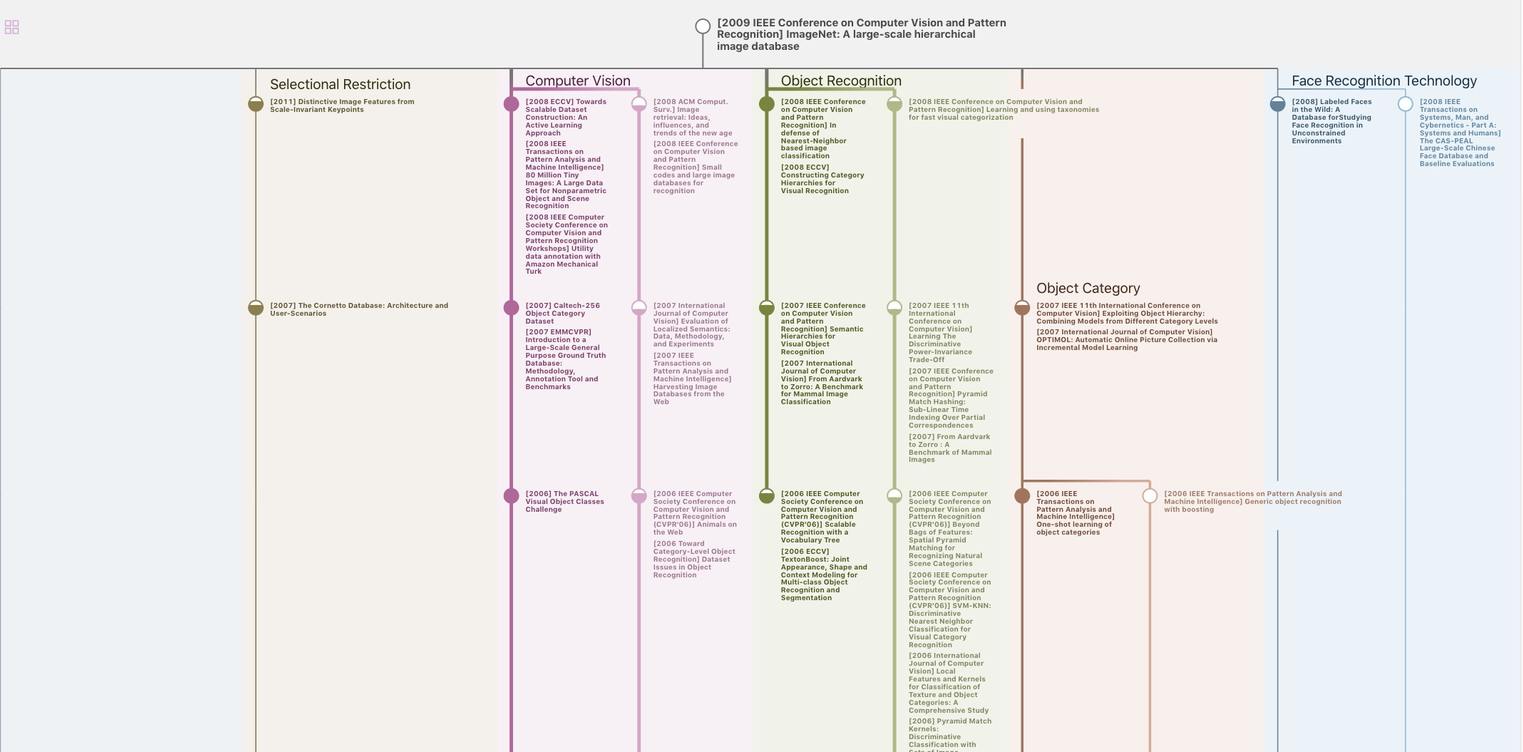
生成溯源树,研究论文发展脉络
Chat Paper
正在生成论文摘要