Matrix Completability Analysis Via Graph K-Connectivity
INTERNATIONAL CONFERENCE ON ARTIFICIAL INTELLIGENCE AND STATISTICS, VOL 84(2018)
摘要
The problem of low-rank matrix completion is continually attracting attention for its applicability to many real-world problems. Still, the large size, extreme sparsity, and non-uniformity of these matrices pose a challenge. In this paper, we make the observation that even when the observed matrix is not suitable for accurate completion there may be portions of the data where completion is still possible. We propose the CompleteID algorithm, which exploits the non-uniformity of the observation, to analyze the completability of the input instead of blindly applying completion. Balancing statistical accuracy with computational efficiency, we relate completability to edge-connectivity of the graph associated with the input partially-observed matrix. We develop the MaxKCD algorithm for finding maximally k-edge-connected components efficiently. Experiments across datasets from a variety of applications demonstrate not only the success of CompleteID but also the importance of completability analysis.
更多查看译文
AI 理解论文
溯源树
样例
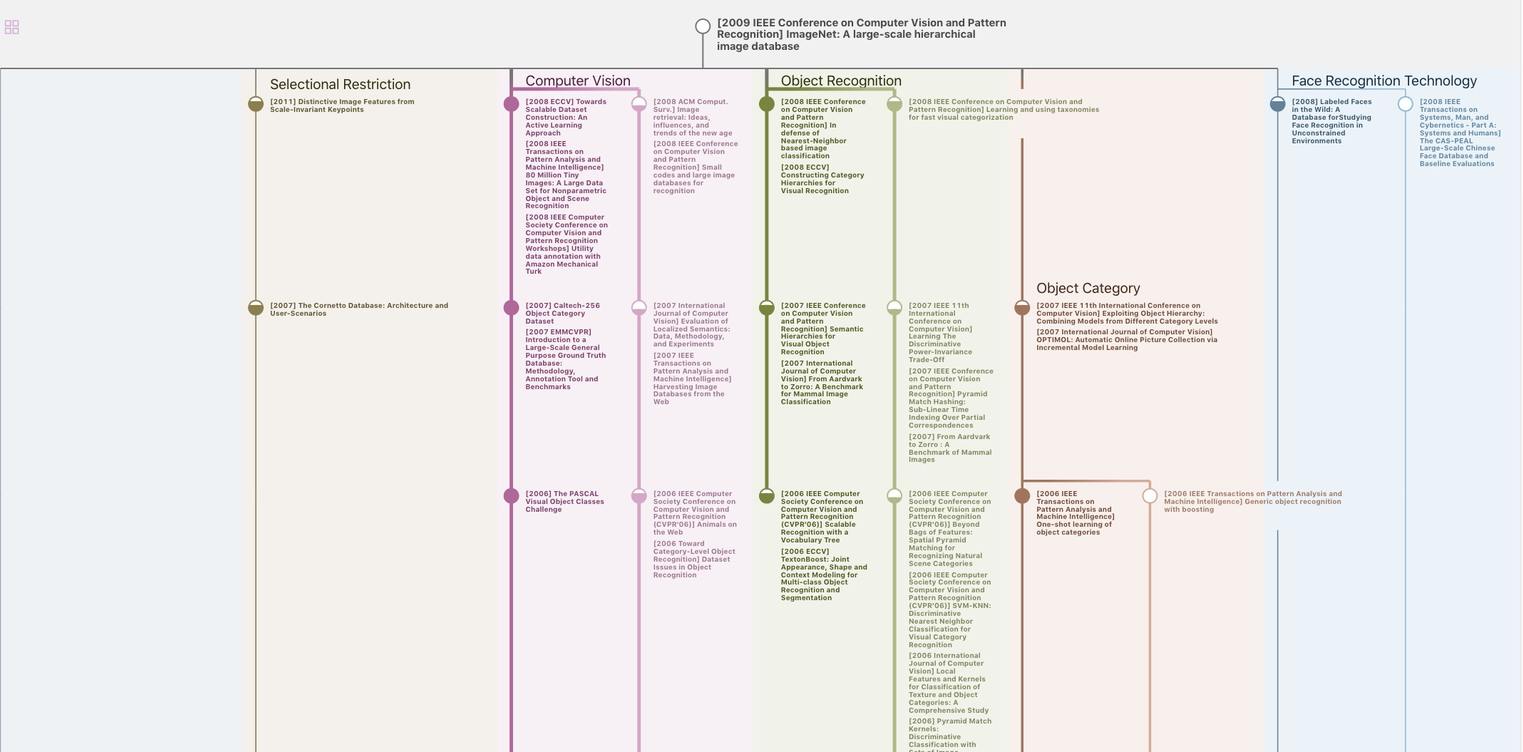
生成溯源树,研究论文发展脉络
Chat Paper
正在生成论文摘要