The De-Anonymization Method Based On User Spatio-Temporal Mobility Trace
INFORMATION AND COMMUNICATIONS SECURITY, ICICS 2017(2017)
摘要
Nowadays user mobility traces are more and more likely to de-anonymize users in addition to other types of data. Among them, model-based approaches usually provide more accurate de-anonymize than that of feature-locations-based approaches. More recently, Hidden Markov Model (HMM) based approaches are proposed to find out the mobility pattern of user mobility traces. However, in the key step of the hidden state definition, existing models rely on the fixed classification of time, space or number, which can hardly suit for all various users. In this paper, we propose a user de-anonymization method based on HMM. Different from current approaches, the method utilizes a novel density-based HMM, which uses the density-based clustering to obtain hidden states of HMM from three-dimensional (time, latitude and longitude) spatio-temporal data, and provide much better performance. Furthermore, we also propose a frequent spatio-temporal cube filter (FSTC-Filter) which significantly reduces the number of candidate models and thus improves the efficiency.
更多查看译文
关键词
De-anonymization, Density-based HMM, Frequent spatio-temporal cube, Mobility trace
AI 理解论文
溯源树
样例
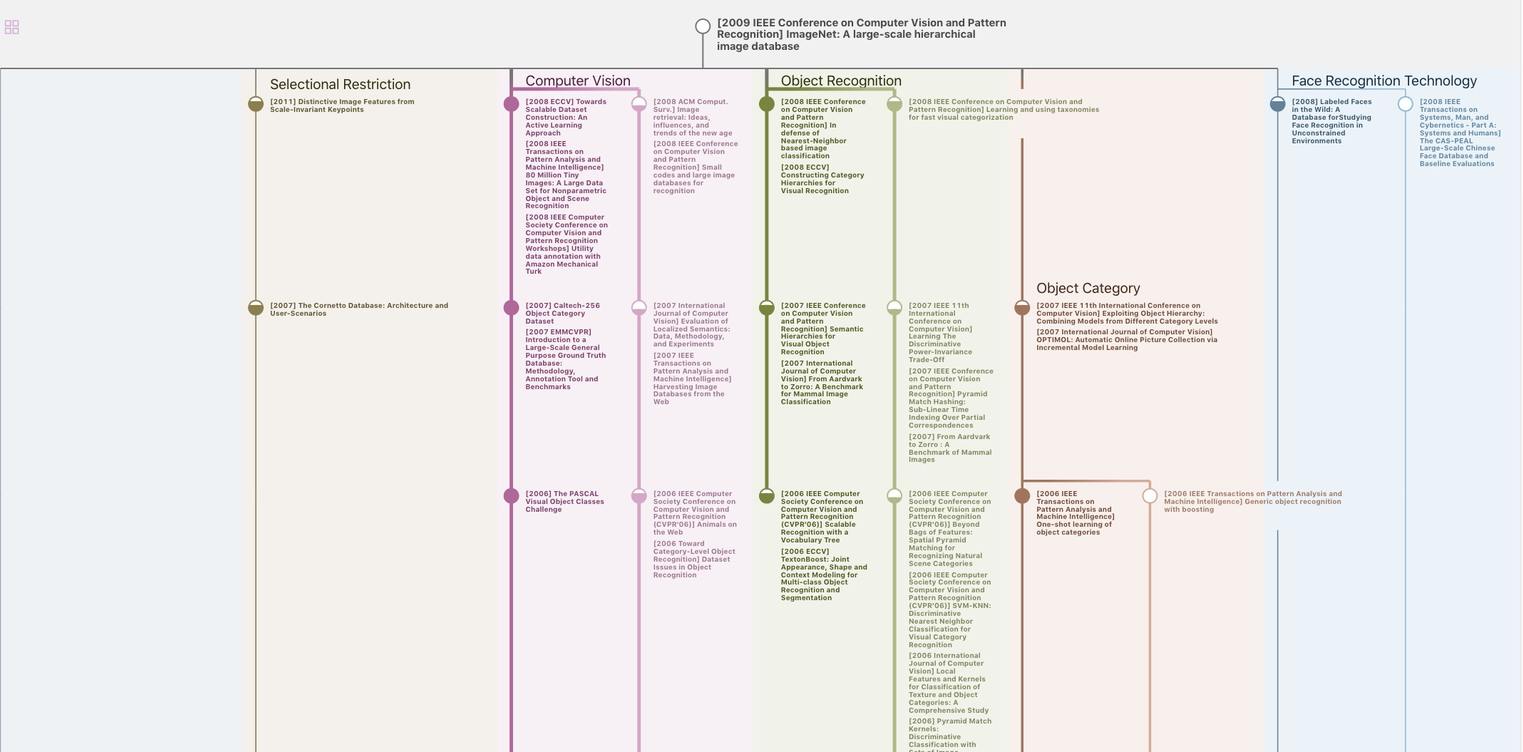
生成溯源树,研究论文发展脉络
Chat Paper
正在生成论文摘要