Aecid: A Self-Learning Anomaly Detection Approach Based On Light-Weight Log Parser Models
ICISSP: PROCEEDINGS OF THE 4TH INTERNATIONAL CONFERENCE ON INFORMATION SYSTEMS SECURITY AND PRIVACY(2018)
摘要
In recent years, new forms of cyber attacks with an unprecedented sophistication level have emerged. Additionally, systems have grown to a size and complexity so that their mode of operation is barely understandable any more, especially for chronically understaffed security teams. The combination of ever increasing exploitation of zero day vulnerabilities, malware auto-generated from tool kits with varying signatures, and the still problematic lack of user awareness is alarming. As a consequence signature-based intrusion detection systems, which look for signatures of known malware or malicious behavior studied in labs, do not seem fit for future challenges. New, flexibly adaptable forms of intrusion detection systems (IDS), which require just minimal maintenance and human intervention, and rather learn themselves what is considered normal in an infrastructure, are a promising means to tackle today's serious security situation. This paper introduces AECID, a new anomaly-based IDS approach, that incorporates many features motivated by recent research results, including the automatic classification of events in a network, their correlation, evaluation, and interpretation up to a dynamically-configurable alerting system. Eventually, we foresee AECID to be a smart sensor for established SIEM solutions. Parts of AECID are open source and already included in Debian Linux and Ubuntu. This paper provides vital information on its basic design, deployment scenarios and application cases to support the research community as well as early adopters of the software package.
更多查看译文
关键词
Anomaly Detection, Intrusion Detection System, Machine Learning, Log Analysis
AI 理解论文
溯源树
样例
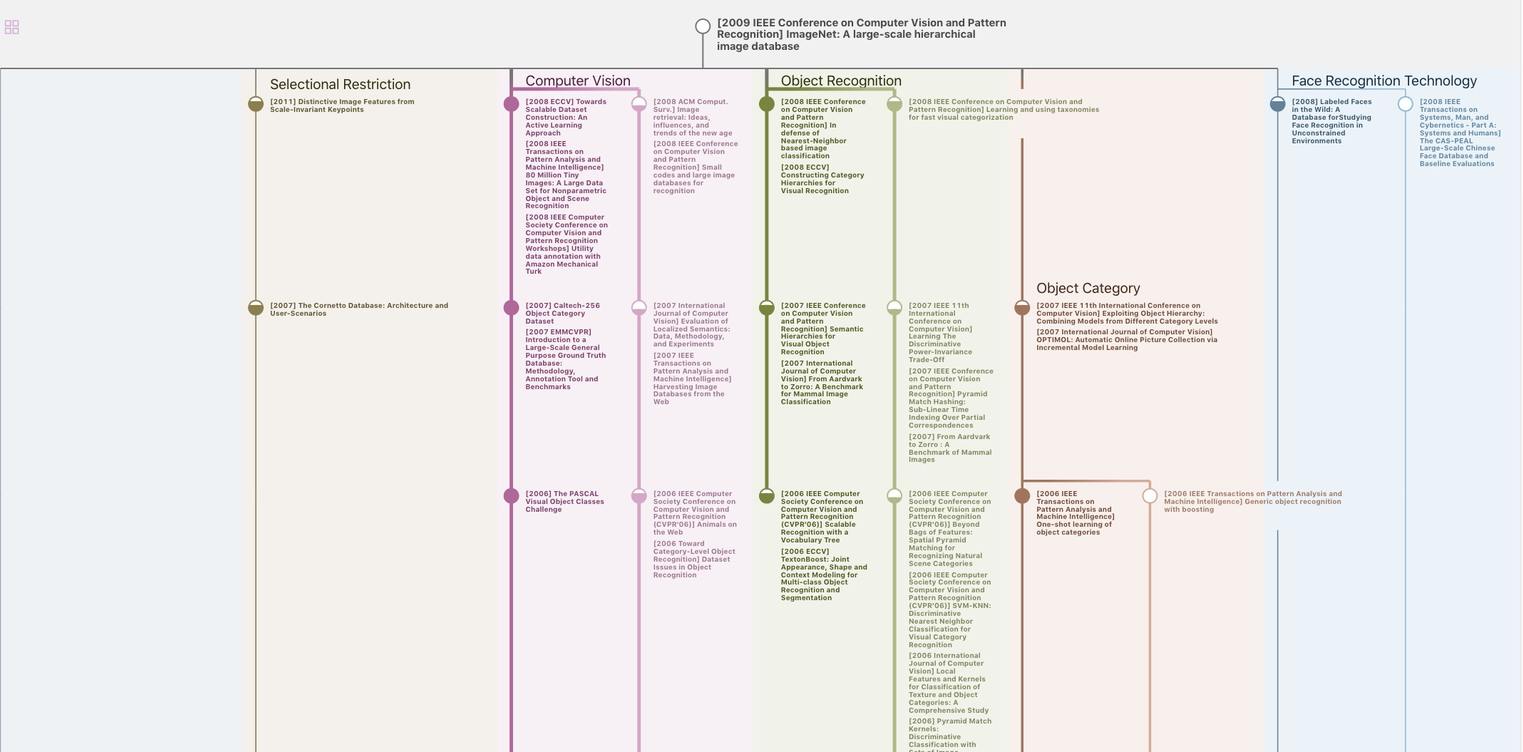
生成溯源树,研究论文发展脉络
Chat Paper
正在生成论文摘要