Transfer Learning For Handwriting Recognition On Historical Documents
PROCEEDINGS OF THE 7TH INTERNATIONAL CONFERENCE ON PATTERN RECOGNITION APPLICATIONS AND METHODS (ICPRAM 2018)(2018)
摘要
In this work, we investigate handwriting recognition on new historical handwritten documents using transfer learning. Establishing a manual ground-truth of a new collection of handwritten documents is time consuming but needed to train and to test recognition systems. We want to implement a recognition system without performing this annotation step. Our research deals with transfer learning from heterogeneous datasets with a ground-truth and sharing common properties with a new dataset that has no ground-truth. The main difficulties of transfer learning lie in changes in the writing style, the vocabulary, and the named entities over centuries and datasets. In our experiment, we show how a CNN-BLSTM-CTC neural network behaves, for the task of transcribing handwritten titles of plays of the Italian Comedy, when trained on combinations of various datasets such as RIMES, Georges Washington, and Los Esposalles. We show that the choice of the training datasets and the merging methods are determinant to the results of the transfer learning task.
更多查看译文
关键词
Handwriting Recognition, Historical Document, Transfer Learning, Deep Neural Network, Unlabeled Data
AI 理解论文
溯源树
样例
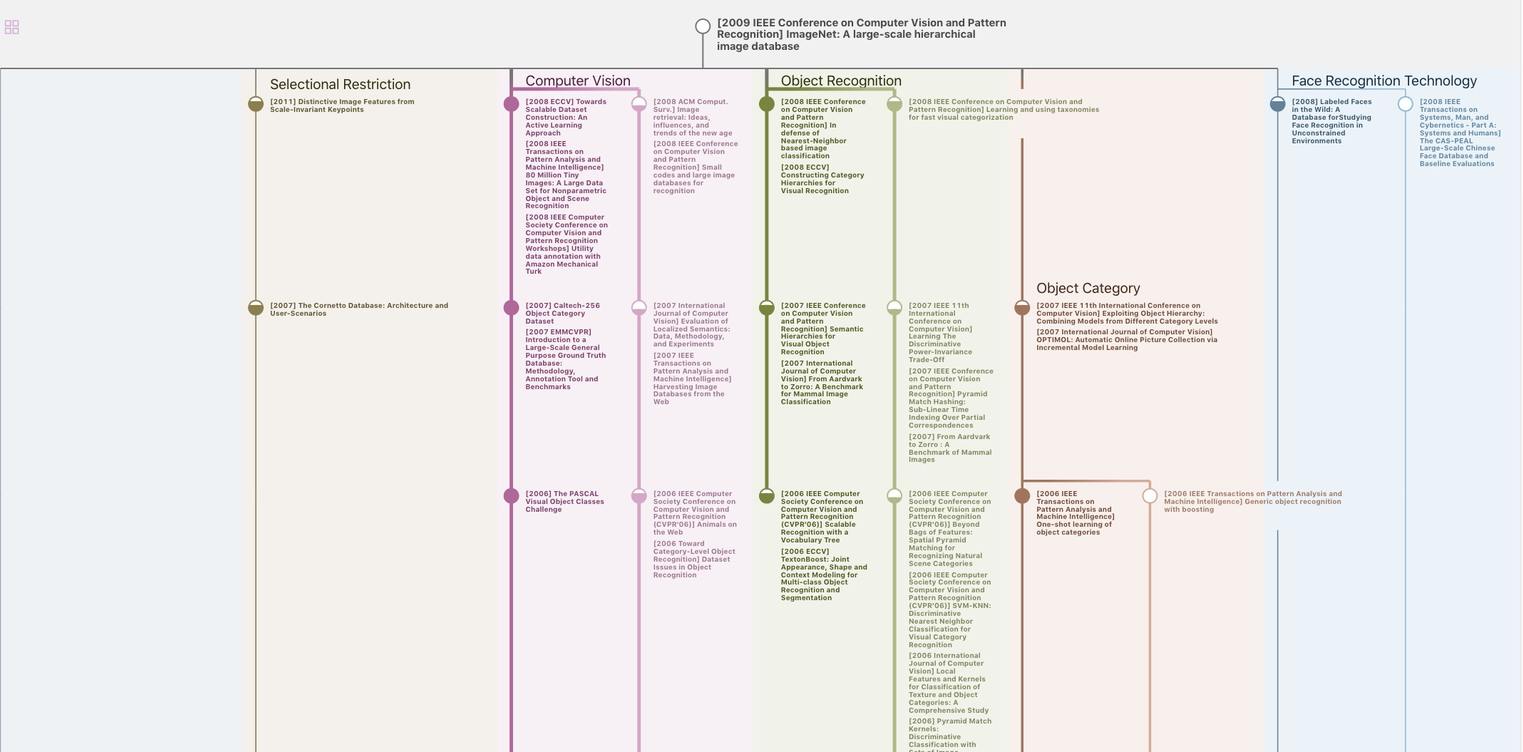
生成溯源树,研究论文发展脉络
Chat Paper
正在生成论文摘要