Investigating the Effect of the Multiple Comparisons Problem in Visual Analysis.
CHI(2018)
摘要
The goal of a visualization system is to facilitate dataset-driven insight discovery. But what if the insights are spurious? Features or patterns in visualizations can be perceived as relevant insights, even though they may arise from noise. We often compare visualizations to a mental image of what we are interested in: a particular trend, distribution or an unusual pattern. As more visualizations are examined and more comparisons are made, the probability of discovering spurious insights increases. This problem is well-known in Statistics as the multiple comparisons problem (MCP) but overlooked in visual analysis. We present a way to evaluate MCP in visualization tools by measuring the accuracy of user reported insights on synthetic datasets with known ground truth labels. In our experiment, over 60% of user insights were false. We show how a confirmatory analysis approach that accounts for all visual comparisons, insights and non-insights, can achieve similar results as one that requires a validation dataset.
更多查看译文
关键词
Multiple Comparisons Problem, Visual Analysis, Visualization, Statistics, Experiment
AI 理解论文
溯源树
样例
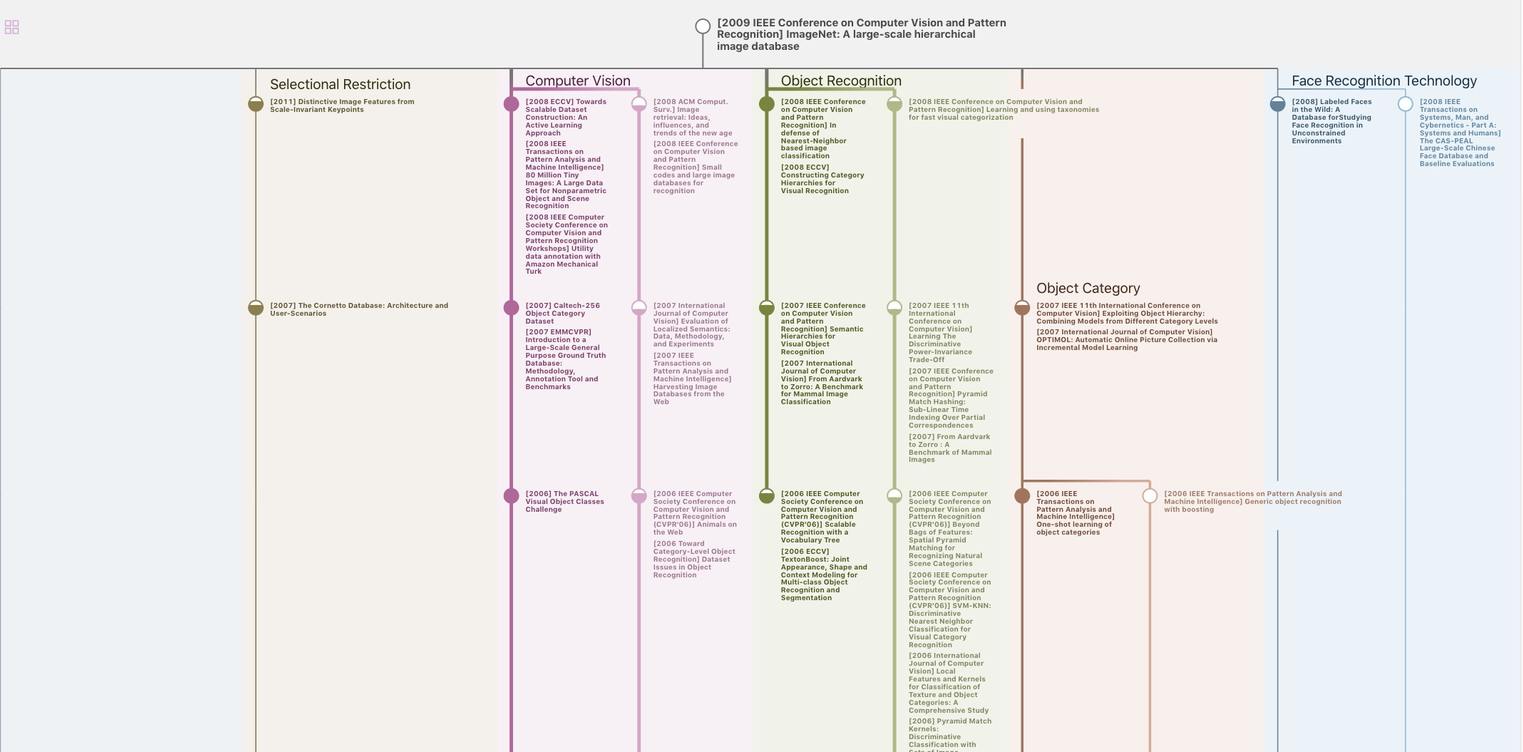
生成溯源树,研究论文发展脉络
Chat Paper
正在生成论文摘要