Ml Approach For Early Detection Of Sleep Apnea Treatment Abandonment: A Case Study
DH '18: PROCEEDINGS OF THE 2018 INTERNATIONAL CONFERENCE ON DIGITAL HEALTH(2018)
摘要
Sleep apnea is a growing problem in the country, with over 200,000 new cases being identified each year. Continuous positive airway pressure (CPAP) is the best treatment for obstructive sleep apnea (OSA), but is limited by low adherence to treatment. Fairview's Sleep program actively tracks CPAP usage and outcomes and employs tele-health coaching to improve adherence. This labor-intensive protocol is applied to those who are failing to meet early adherence targets. However, the implementation of this is based on heuristic rules which may not be matched to actual outcomes, contacting some patients too late and others unnecessarily. Machine learning can facilitate efficient contact strategies through early and accurate identification of therapy trajectories based on patient history, including EHR data, health information, questionnaires, and daily PAP metrics. Prediction models for classification of patients regarding CPAP adherence at a clinically-important time of 6 months of regular use were built. Using data from the first 30 days of CPAP usage, and a more aggressive decision scenario from the first 13 days of usage, the proposed approach results in an improvement in prediction significantly better than the current approach used by the hospital. Further, it is shown that a hospital can utilize this precise and earlier prediction by implementing appropriate actions based on the patient's predicted risk level.
更多查看译文
关键词
Machine Learning, Health Informatics, Sleep Apnea Treatment, CPAP Adherence
AI 理解论文
溯源树
样例
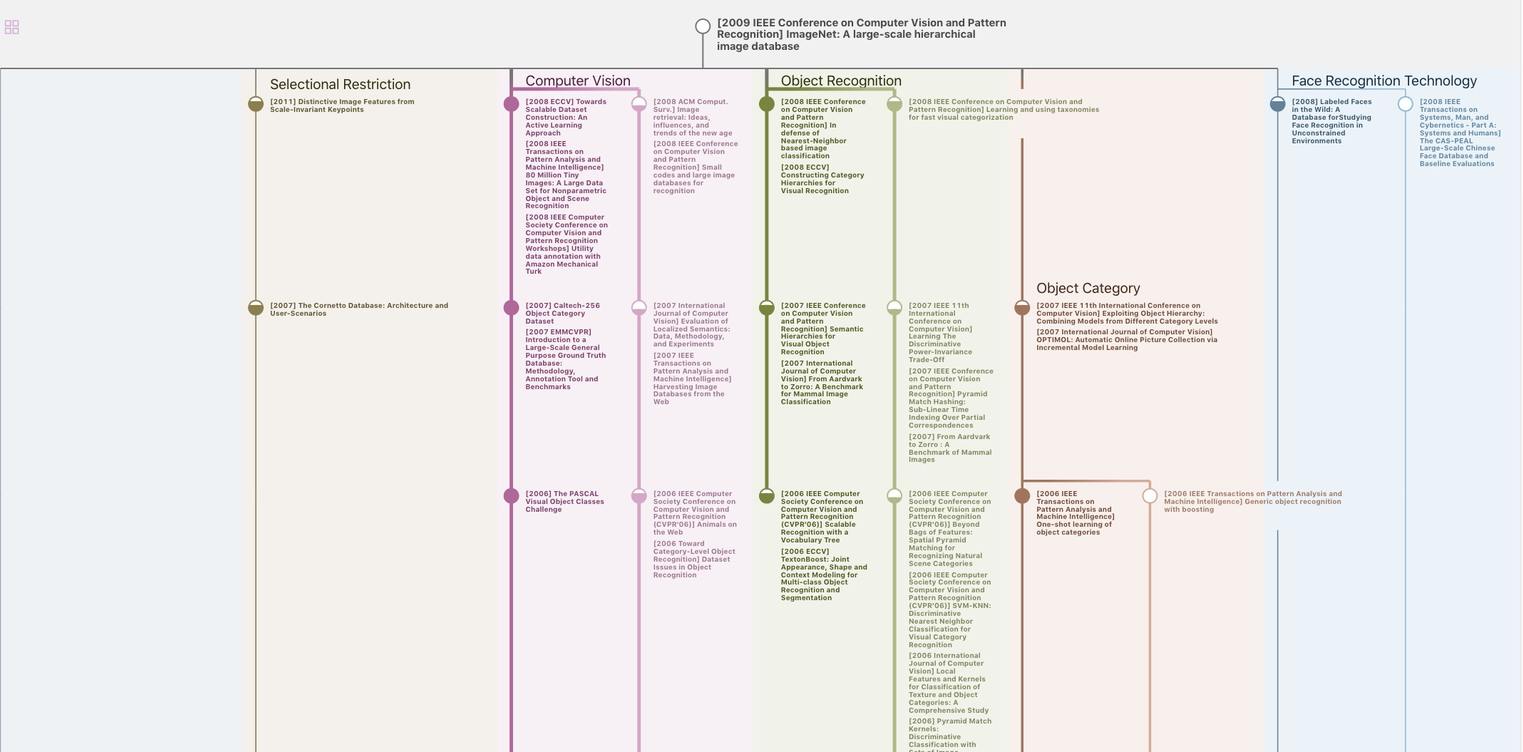
生成溯源树,研究论文发展脉络
Chat Paper
正在生成论文摘要