Short-Term Electricity Price Forecasting With Stacked Denoising Autoencoders
IEEE TRANSACTIONS ON POWER SYSTEMS(2017)
摘要
A short-term forecasting of the electricity price with data-driven algorithms is studied in this research. A stacked denoising autoencoder (SDA) model, a class of deep neural networks, and its extended version are utilized to forecast the electricity price hourly. Data collected in Nebraska, Arkansas, Louisiana, Texas, and Indiana hubs in U.S. are utilized. Two types of forecasting, the online hourly forecasting and day-ahead hourly forecasting, are examined. In online forecasting, SDA models are compared with data-driven approaches including the classical neural networks, support vector machine, multivariate adaptive regression splines, and least absolute shrinkage and selection operator. In the day-ahead forecasting, the effectiveness of SDA models is further validated through comparing with industrial results and a recently reported method. Computational results demonstrate that SDA models are capable to accurately forecast electricity prices and the extended SDA model further improves the forecasting performance.
更多查看译文
关键词
Comparative analysis, data mining, electricity price, hourly forecasting, neural networks
AI 理解论文
溯源树
样例
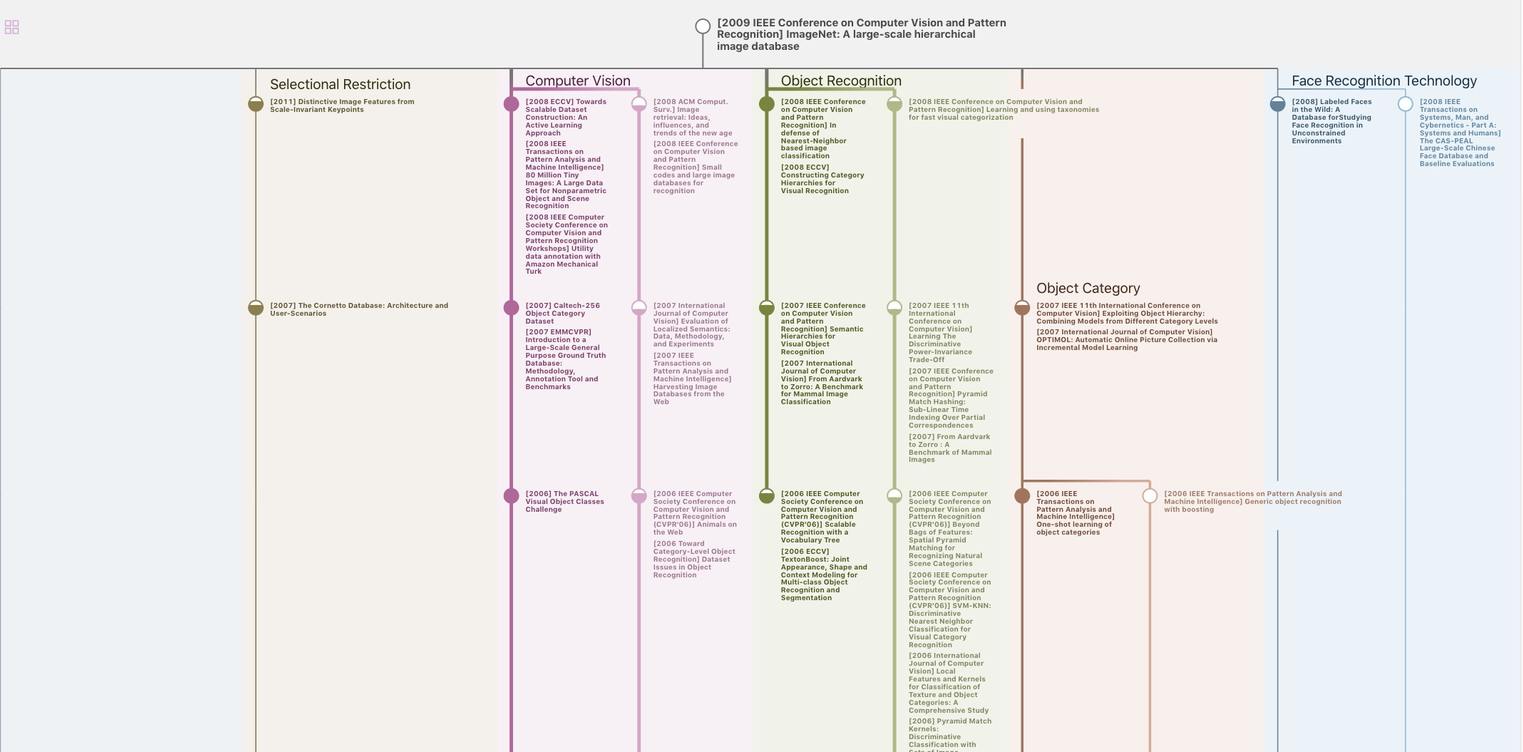
生成溯源树,研究论文发展脉络
Chat Paper
正在生成论文摘要