Fast Spatial Gaussian Process Maximum Likelihood Estimation via Skeletonization Factorizations.
Multiscale Modeling & Simulation(2017)
摘要
Maximum likelihood estimation for parameter fitting given observations from a Gaussian process in space is a computationally demanding task that restricts the use of such methods to moderately sized datasets. We present a framework for unstructured observations in two spatial dimensions that allows for evaluation of the log-likelihood and its gradient (i.e., the score equations) in $tilde O(n^{3/2})$ time under certain assumptions, where $n$ is the number of observations. Our method relies on the skeletonization procedure described by Martinsson and Rokhlin [J. Comput. Phys., 205 (2005), pp. 1--23] in the form of the recursive skeletonization factorization of Ho and Ying [Comm. Pure Appl. Math., 69 (2015), pp. 1415--1451]. Combining this with an adaptation of the matrix peeling algorithm of Lin, Lu, and Ying [J. Comput. Phys., 230 (2011), pp, 4071--4087] for constructing $mathcal{H}$-matrix representations of black-box operators, we obtain a framework that can be used in the context of any first-order o...
更多查看译文
关键词
process,spatial,factorizations,estimation
AI 理解论文
溯源树
样例
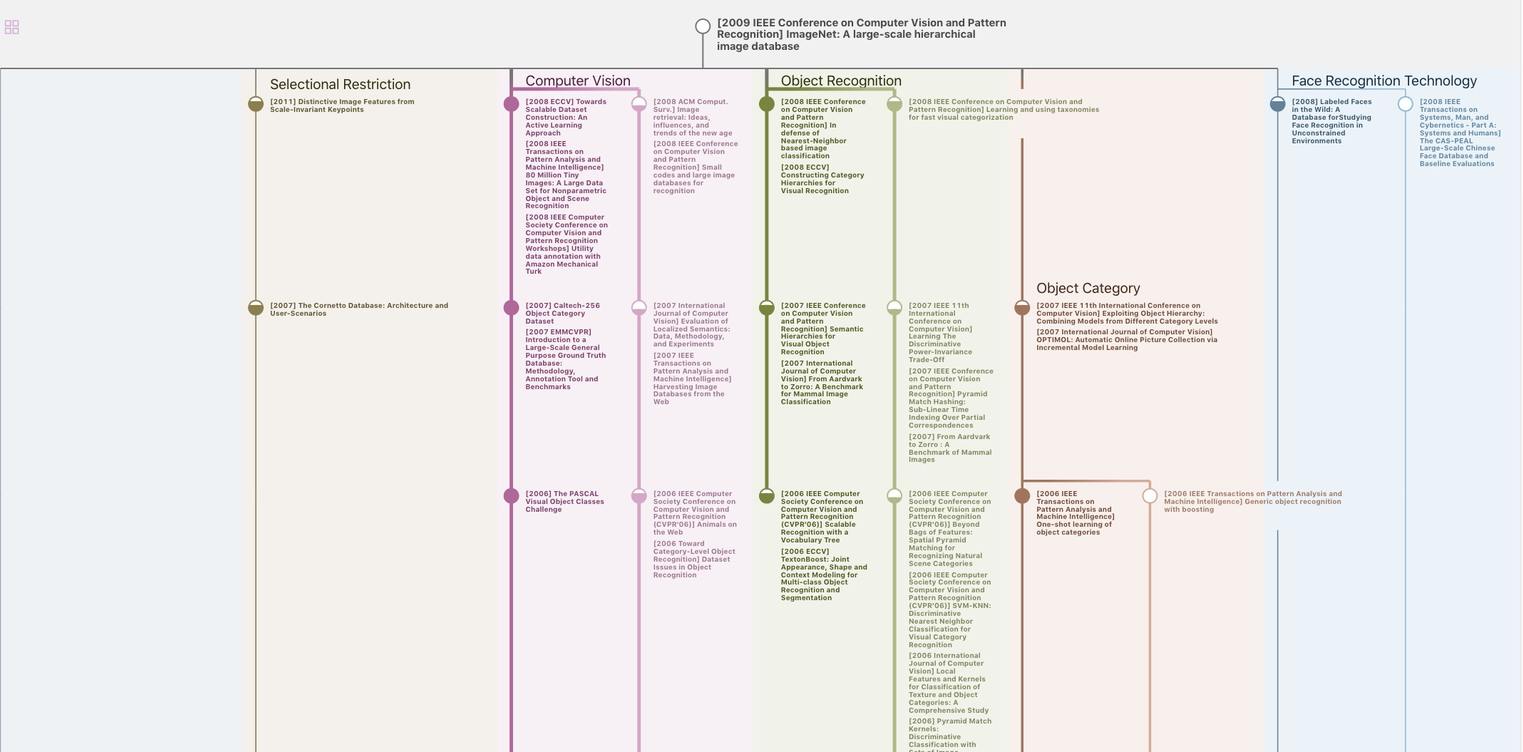
生成溯源树,研究论文发展脉络
Chat Paper
正在生成论文摘要