When Low Rank Representation Based Hyperspectral Imagery Classification Meets Segmented Stacked Denoising Auto-Encoder Based Spatial-Spectral Feature.
REMOTE SENSING(2018)
摘要
When confronted with limited labelled samples, most studies adopt an unsupervised feature learning scheme and incorporate the extracted features into a traditional classifier (e.g., support vector machine, SVM) to deal with hyperspectral imagery classification. However, these methods have limitations in generalizing well in challenging cases due to the limited representative capacity of the shallow feature learning model, as well as the insufficient robustness of the classifier which only depends on the supervision of labelled samples. To address these two problems simultaneously, we present an effective low-rank representation-based classification framework for hyperspectral imagery. In particular, a novel unsupervised segmented stacked denoising auto-encoder-based feature learning model is proposed to depict the spatial-spectral characteristics of each pixel in the imagery with deep hierarchical structure. With the extracted features, a low-rank representation based robust classifier is then developed which takes advantage of both the supervision provided by labelled samples and unsupervised correlation (e.g., intra-class similarity and inter-class dissimilarity, etc.) among those unlabelled samples. Both the deep unsupervised feature learning and the robust classifier benefit, improving the classification accuracy with limited labelled samples. Extensive experiments on hyperspectral imagery classification demonstrate the effectiveness of the proposed framework.
更多查看译文
关键词
deep unsupervised feature learning,segmented stacked denoising auto-encoder,low rank representation,hyperspectral imagery classification
AI 理解论文
溯源树
样例
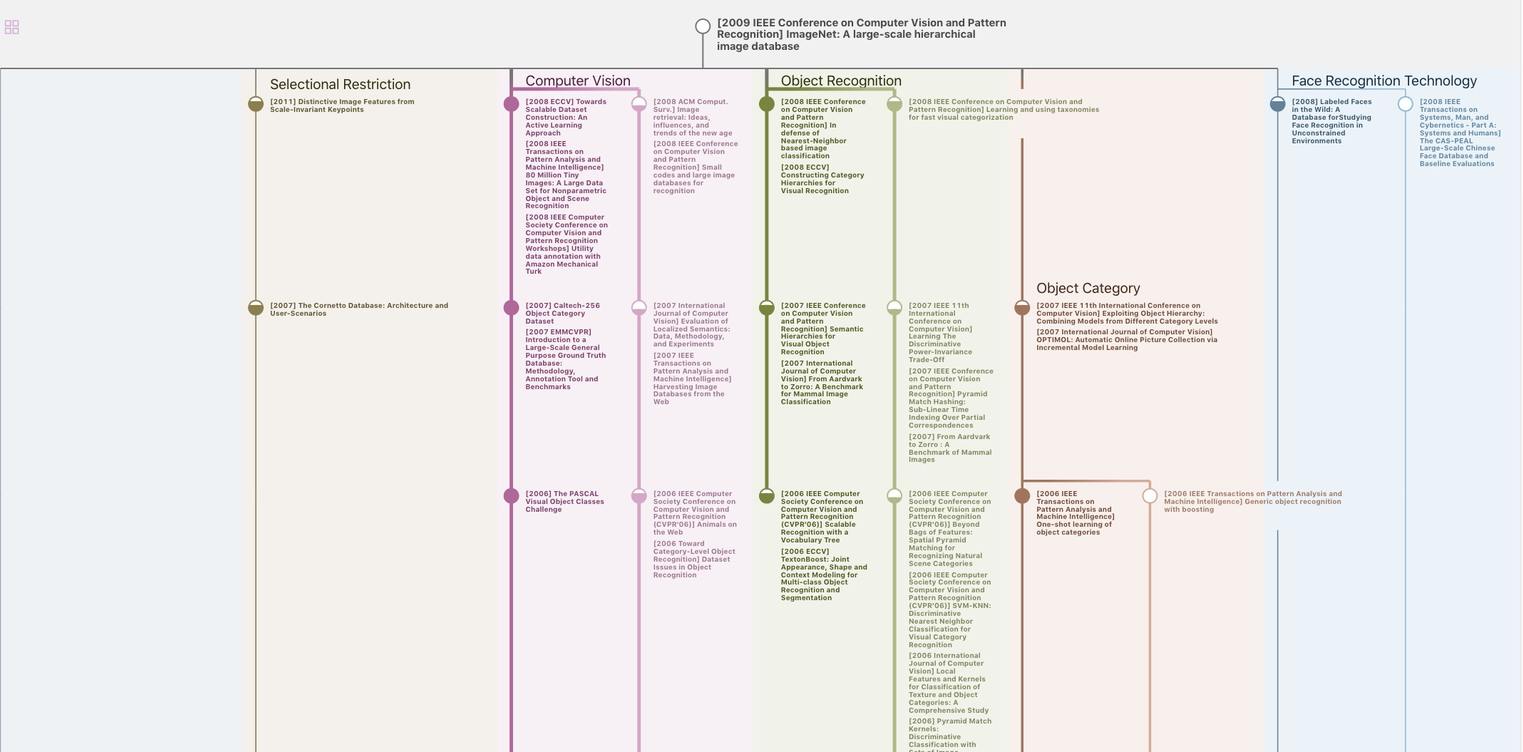
生成溯源树,研究论文发展脉络
Chat Paper
正在生成论文摘要