An Effective Two-Step Intrusion Detection Approach Based on Binary Classification and $k$ -NN.
IEEE Access(2018)
摘要
Intrusion detection has been an important countermeasure to secure computing infrastructures from malicious attacks. To improve detection performance and reduce bias towards frequent attacks, this paper proposes a two-step hybrid method based on binary classification and $k$ -NN technique. Step 1 employs several binary classifiers and one aggregation module to effectively detect the exact classes of network connections. After step 1, the connections whose classes are uncertain are sent to step 2 to further determine their classes by the $k$ -NN algorithm. Step 2 is based on the outcomes of step 1 and yields a beneficial supplement to step 1. By combining the two steps, the proposed method achieves reliable results on the NSL-KDD data set. The effectiveness of the proposed method is evaluated in comparison with five supervised learning techniques. Experimental results demonstrate that the proposed method outperforms baselines with respect to various evaluation criteria. In particular, for U2R and R2L attacks, the F1-scores of the proposed method are much higher than those of baselines. Furthermore, comparisons with some recent hybrid approaches are also listed. The results illustrate that the proposed method is competitive.
更多查看译文
关键词
Intrusion detection,hybrid method,binary classification,C4.5,k-nearest neighbors
AI 理解论文
溯源树
样例
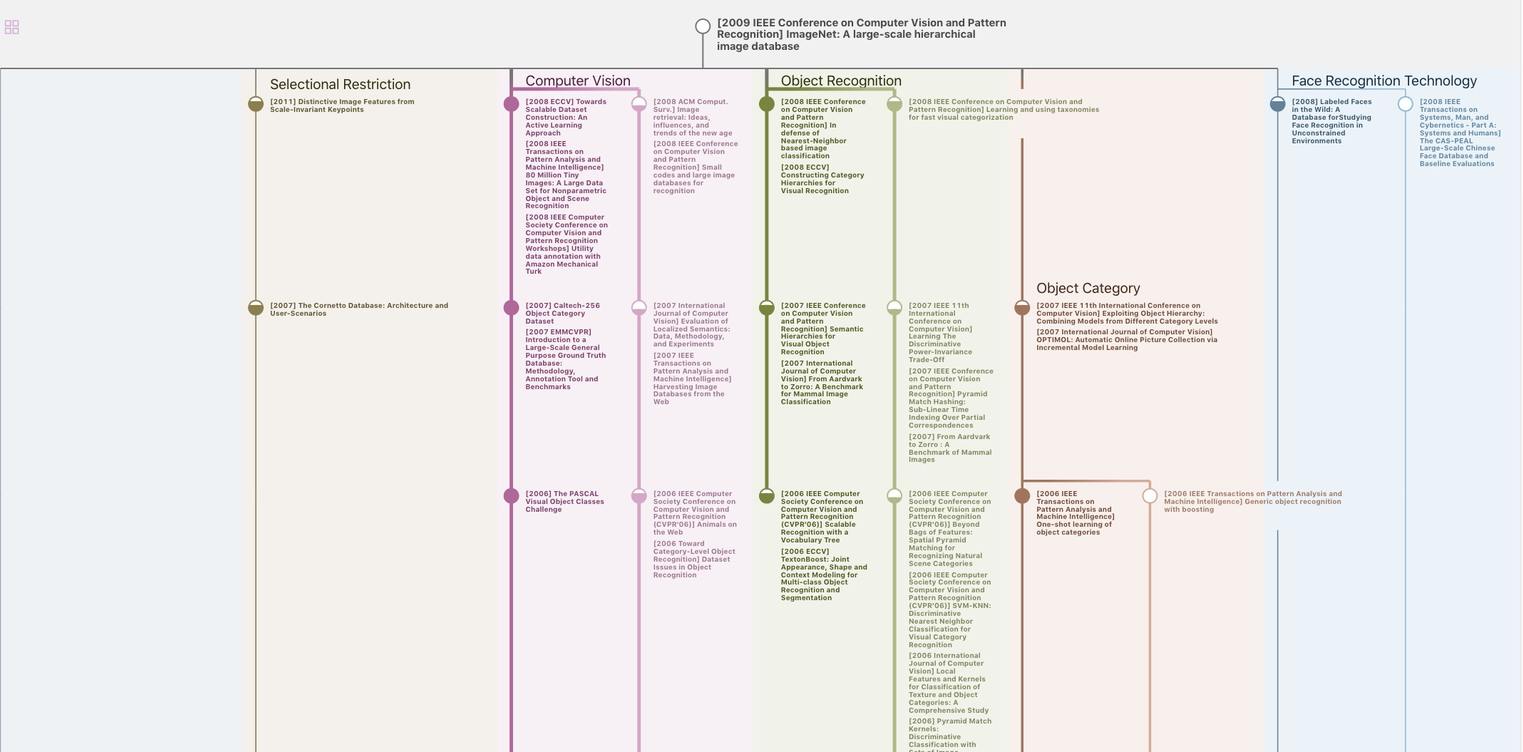
生成溯源树,研究论文发展脉络
Chat Paper
正在生成论文摘要