Improved Boundary Equilibrium Generative Adversarial Networks.
IEEE ACCESS(2018)
摘要
Boundary equilibrium generative adversarial networks (BEGANs) can generate impressively realistic face images, but there is a trade-off between the quality and the diversity of generated images. Based on BEGANs, we propose an effective approach to generate images with higher quality and better diversity. By adding a second loss function (a denoising loss) to the discriminator, the discriminator can learn more useful information about the distribution of real images. Naturally, the ability of discriminator in distinguishing between real and generated images is improved, which further guides the generator to produce more realistic images to confuse the discriminator. We also find that using technique of batch normalization in BEGANs architecture can improve the diversity of generated images. By using batch normalization and adding a denoising loss to the objective of discriminator, we achieve comparative generations on CIFAR-10 and CelebA data sets. In addition, we evaluate the effect of several techniques on BEGANs framework through "Inception-Score", a measure which has been found to correlate well with human assessment of generated samples.
更多查看译文
关键词
Generative adversarial networks (GANs),boundary equilibrium generative adversarial networks (BEGANs),deep generative model,image generation
AI 理解论文
溯源树
样例
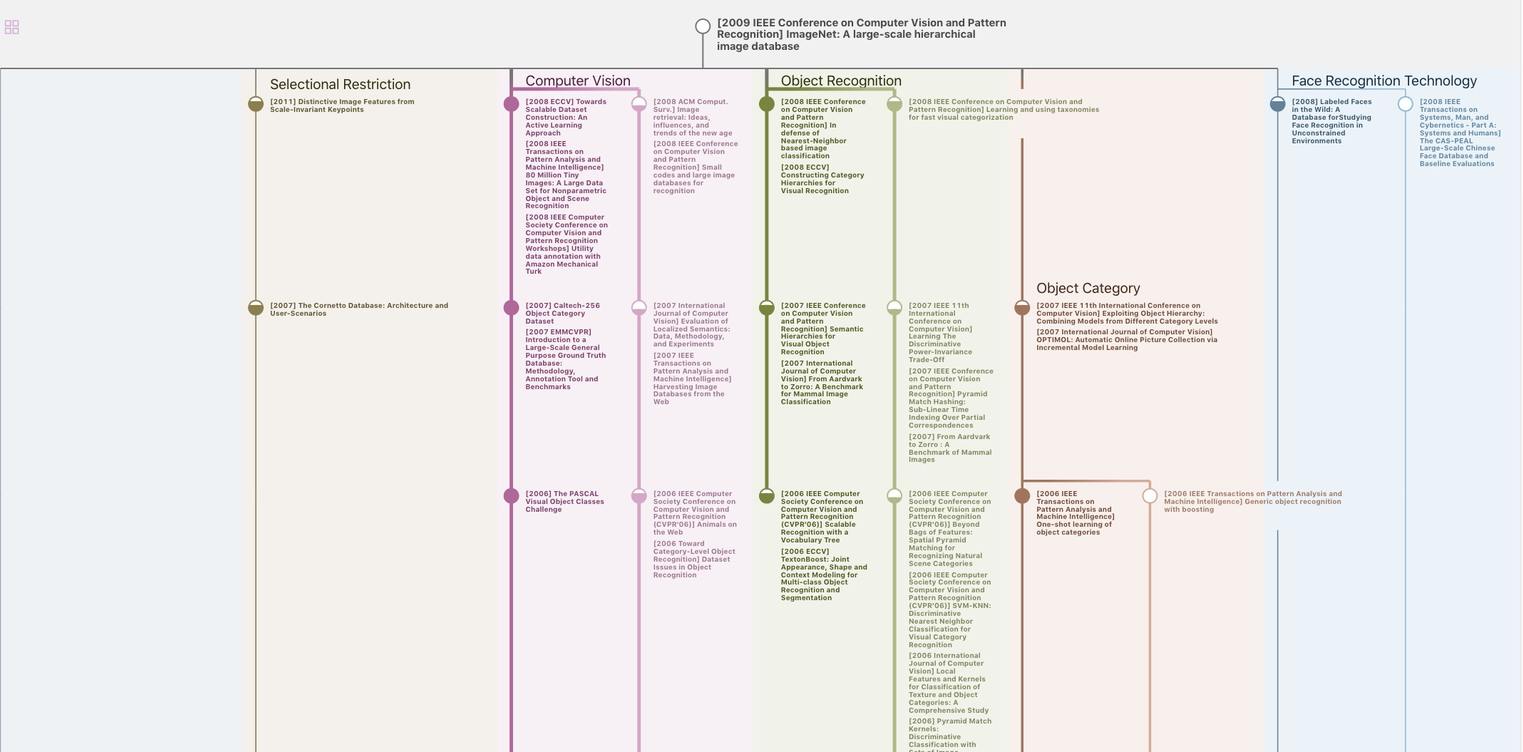
生成溯源树,研究论文发展脉络
Chat Paper
正在生成论文摘要