A safe screening based framework for support vector regression.
Neurocomputing(2018)
摘要
Support vector regression (SVR) is popular and efficient for regression problems. However, it is time-consuming to solve it, especially for large datasets. Inspired by the sparse solutions of SVR, a safe screening based framework for SVR (SVR-SBF), including both linear and nonlinear cases, is proposed in this paper to improve its training speed. This SBF has two steps: First, the constant solutions of SVR along the regularization path of parameter C are deleted before training; Second, a safe screening rule via variational inequalities (SSR-VI) is embedded into the grid search method to further discard the inactive solutions of SVR. This SBF can efficiently accelerate the training speed of SVR without affecting its solutions. Compared to existing safe rules, our SVR-SBF can identify more inactive solutions by finding constant solutions beforehand. In addition, our SBF is further expanded to more situations and models. To be specific, a modified SSR-VI is proposed to be adapted to other parameter selection methods, and models including variants of SVR and classical SVM are analyzed. Experiments on both synthetic and real datasets are conducted to demonstrate the superiority of SVR-SBF.
更多查看译文
关键词
Support vector regression,Safe screening,Grid search,Sample selection
AI 理解论文
溯源树
样例
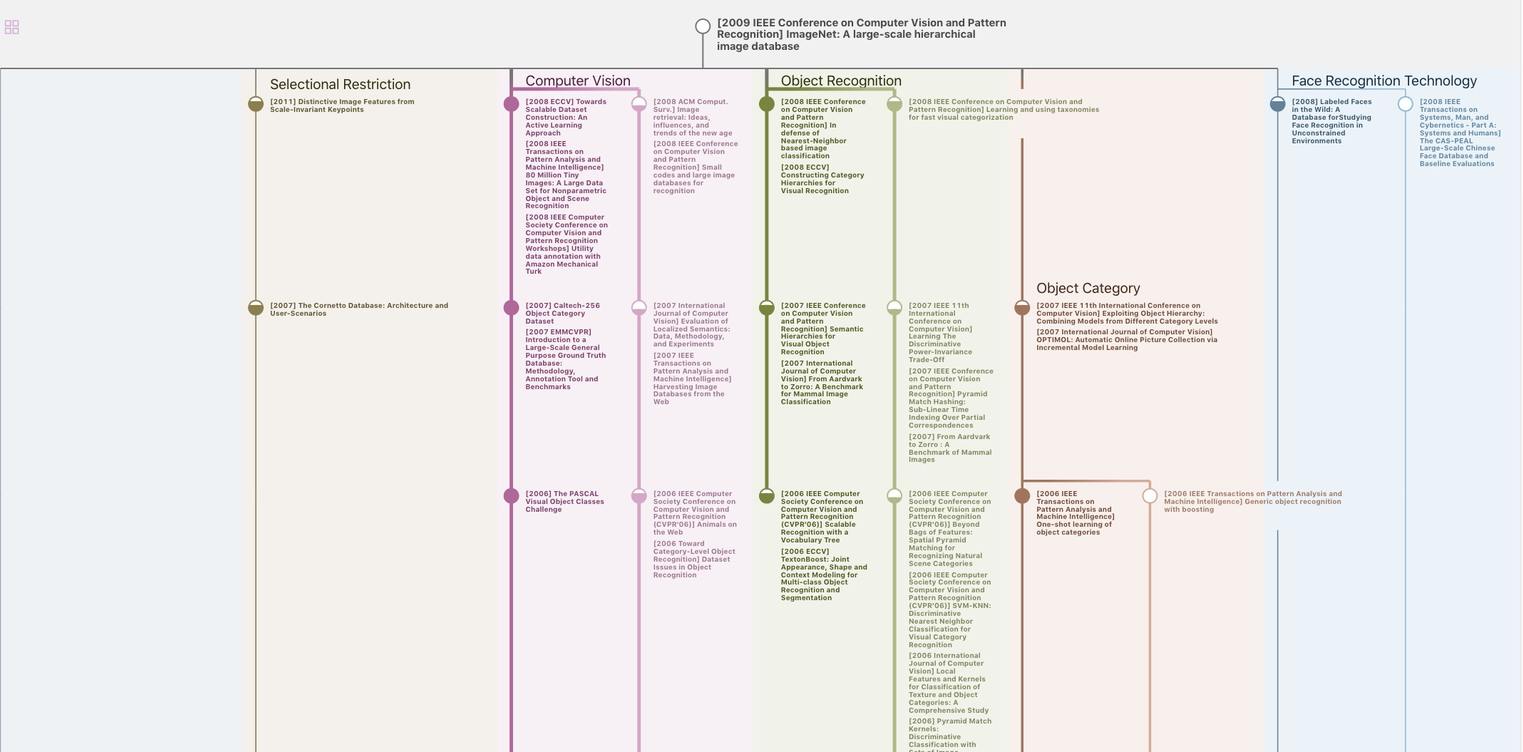
生成溯源树,研究论文发展脉络
Chat Paper
正在生成论文摘要