Kernel And Acquisition Function Setup For Bayesian Optimization Of Gradient Boosting Hyperparameters
INTELLIGENT INFORMATION AND DATABASE SYSTEMS, ACIIDS 2018, PT I(2018)
摘要
The application scenario investigated in the paper is the bank credit scoring based on a Gradient Boosting classifier. It is shown how one may exploit hyperparameter optimization based on the Bayesian Optimization paradigm. All the evaluated methods are based on the Gaussian Process model, but differ in terms of the kernel and the acquisition function. The main purpose of the research presented herein is to confirm experimentally that it is reasonable to tune both the kernel function and the acquisition function in order to optimize Bayesian Gradient Boosting hyperparameters. Moreover, the paper provides results indicating that, at least in the investigated application scenario, the superiority of some of the evaluated Bayesian Optimization methods over others strongly depends on the amount of the optimization budget.
更多查看译文
关键词
Binary classification, Gradient Boosting Hyperparameters, Bayesian Optimization, Gaussian Process Kernel function, Acquisition function, Bank credit scoring
AI 理解论文
溯源树
样例
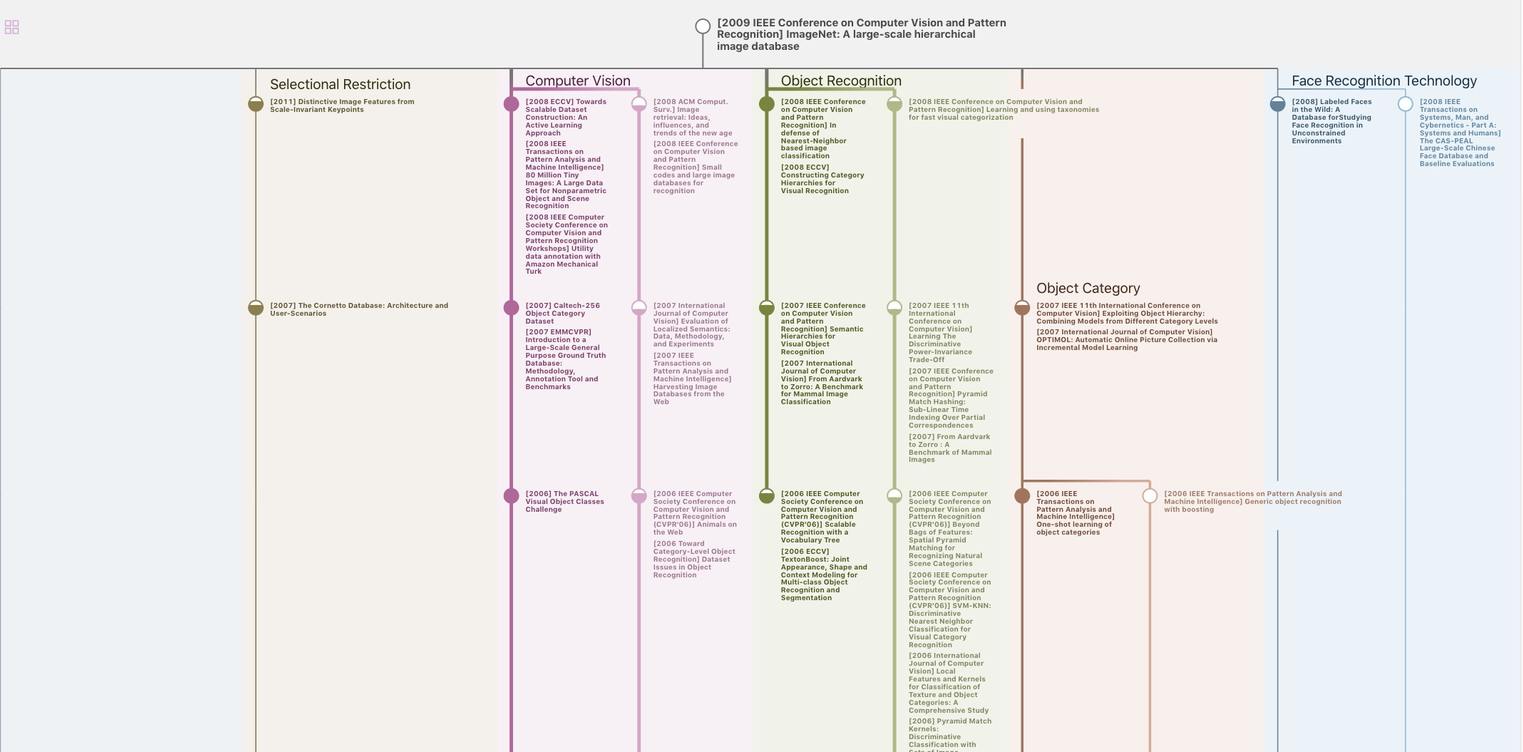
生成溯源树,研究论文发展脉络
Chat Paper
正在生成论文摘要