Automatic liver volume segmentation and fibrosis classification.
Proceedings of SPIE(2018)
摘要
In this work, we present an automatic method for liver segmentation and fibrosis classification in liver computed-tomography (CT) portal phase scans. The input is a full abdomen CT scan with an unknown number of slices, and the output is a liver volume segmentation mask and a fibrosis grade. A multi-stage analysis scheme is applied to each scan, including: volume segmentation, texture features extraction and SVM based classification. Data contains portal phase CT examinations from 80 patients, taken with different scanners. Each examination has a matching Fibroscan grade. The dataset was subdivided into two groups: first group contains healthy cases and mild fibrosis, second group contains moderate fibrosis, severe fibrosis and cirrhosis. Using our automated algorithm, we achieved an average dice index of 0.93 +/- 0.05 for segmentation and a sensitivity of 0.92 and specificity of 0.81 for classification. To the best of our knowledge, this is a first end to end automatic framework for liver fibrosis classification; an approach that, once validated, can have a great potential value in the clinic.
更多查看译文
AI 理解论文
溯源树
样例
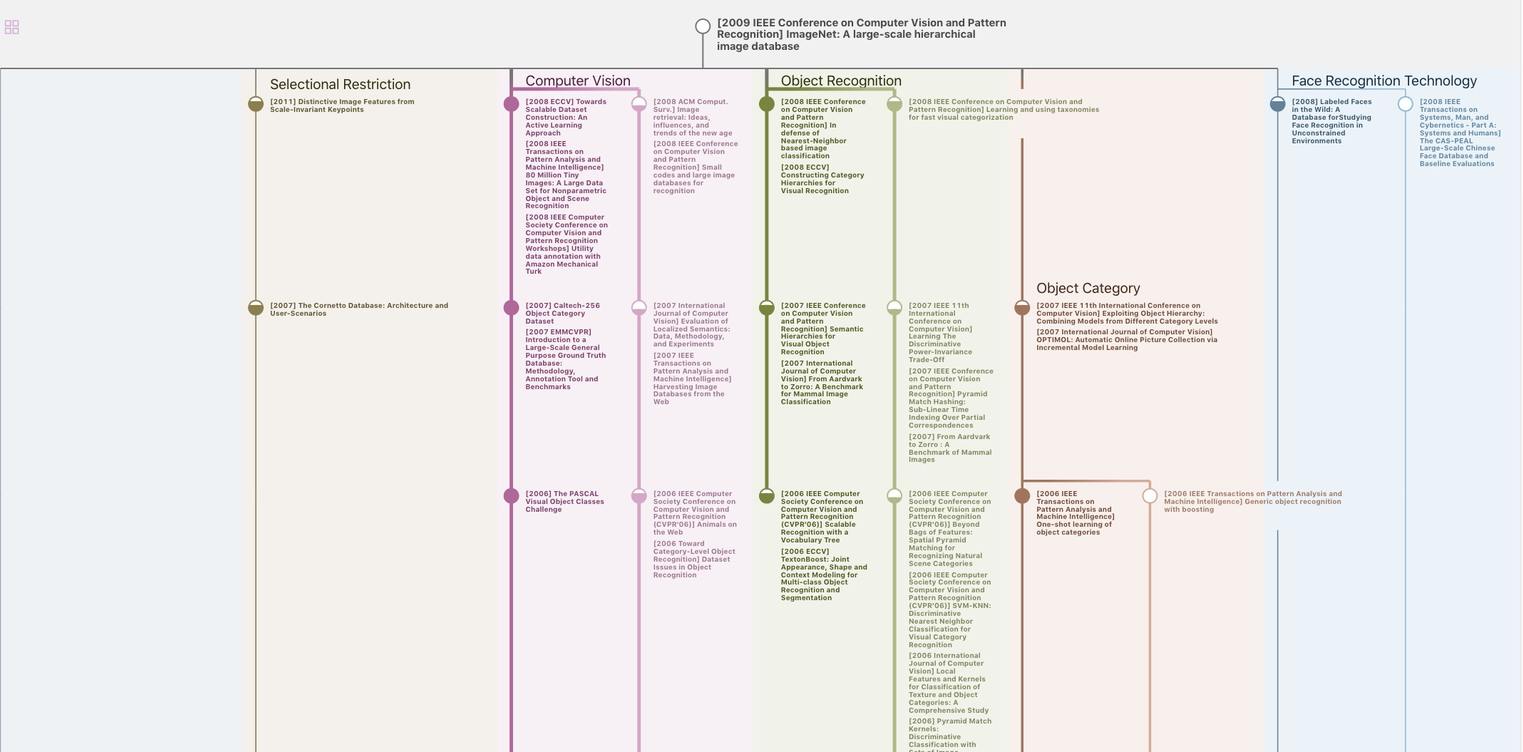
生成溯源树,研究论文发展脉络
Chat Paper
正在生成论文摘要