Expert identification of visual primitives used by CNNs during mammogram classification.
Proceedings of SPIE(2018)
摘要
This work interprets the internal representations of deep neural networks trained for classification of diseased tissue in 2D mammograms. We propose an expert-in-the-loop interpretation method to label the behavior of internal units in convolutional neural networks (CNNs). Expert radiologists identify that the visual patterns detected by the units are correlated with meaningful medical phenomena such as mass tissue and calcificated vessels. We demonstrate that several trained CNN models are able to produce explanatory descriptions to support the final classification decisions. We view this as an important first step toward interpreting the internal representations of medical classification CNNs and explaining their predictions.
更多查看译文
关键词
medical image understanding,deep learning for diagnosis,interpretable machine learning,expert-in-the-loop methods
AI 理解论文
溯源树
样例
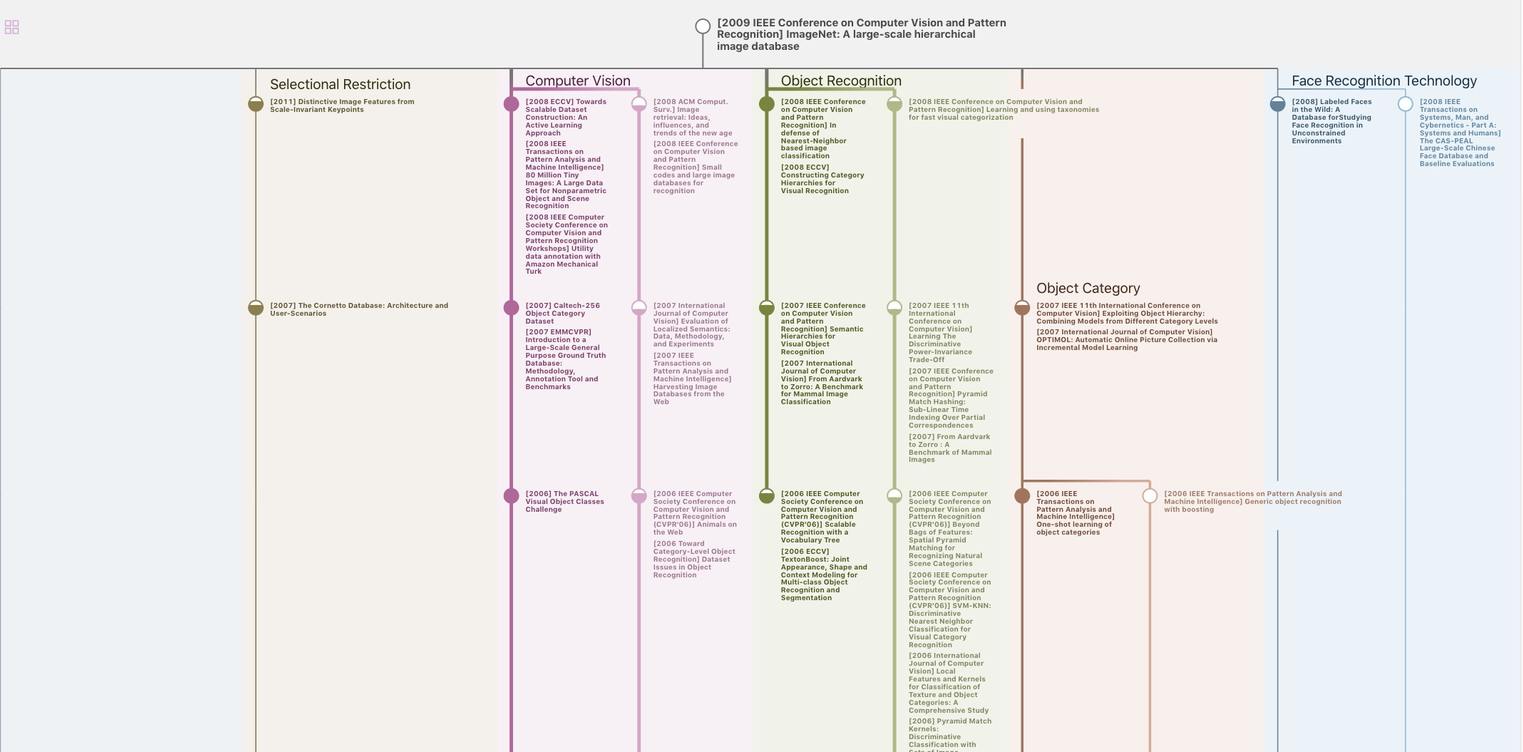
生成溯源树,研究论文发展脉络
Chat Paper
正在生成论文摘要