Joint-sparse dictionary learning: Denoising multiple measurement vectors
IEEE Global Conference on Signal and Information Processing(2017)
摘要
This work addresses the problem of denoising multiple measurement vectors having a common sparse support. Such problems arise in a variety of imaging problems, e.g. color imaging, multi-spectral and hyper-spectral imaging, multi-echo and multi-channel magnetic resonance imaging, etc. For such cases, denoising them piecemeal, one channel at a time, is not optimal; since it does not exploit the full structure (joint sparsity) of the problem. Joint-sparsity based methods have been used for solving such problems when the sparsifying transform is assumed to be fixed. In this work, we learn the sparsifying basis following the dictionary learning paradigm. Results on multi-spectral denoising and multi-echo MRI denoising demonstrates the superiority of our method over existing ones based on KSVD and BM4D.
更多查看译文
关键词
denoising,dictionary learning,joint sparsity
AI 理解论文
溯源树
样例
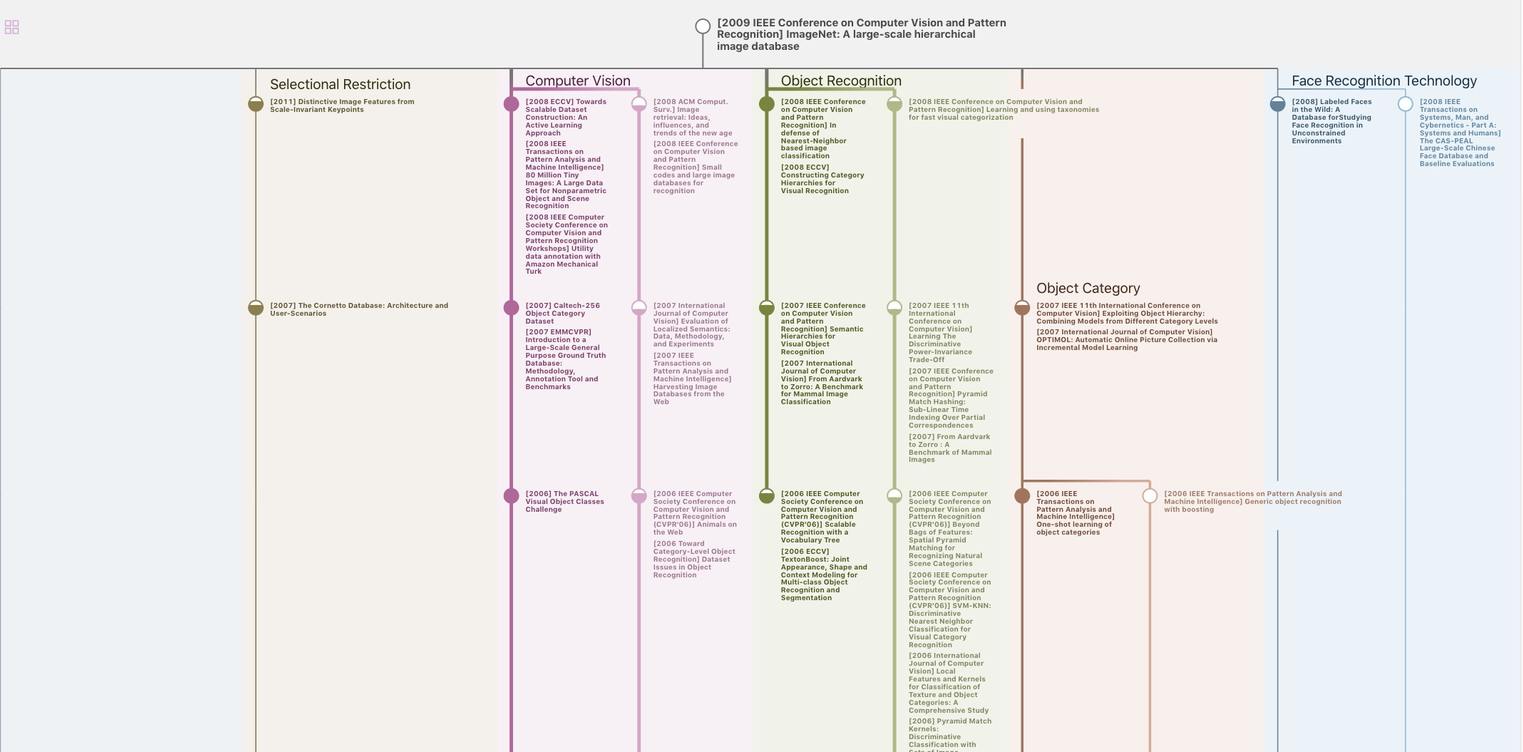
生成溯源树,研究论文发展脉络
Chat Paper
正在生成论文摘要