A convolutional neural network-based approach to rate control in HEVC intra coding
2017 IEEE Visual Communications and Image Processing (VCIP)(2017)
摘要
Rate control is an essential element for the practical use of video coding standards. A rate control scheme typically builds a model that characterizes the relationship between rate (R) and a coding parameter, e.g. quantization parameter or Lagrange multiplier (A). In such a scheme, the rate control performance depends highly on the modeling accuracy. For inter frames, the model parameters can be precisely updated to fit the video content, based on the information of previously coded frames. However, for intra frames, especially the first frame of a video sequence, there is no prior information to rely on. Therefore, intra frame rate control has remained a challenge. In this paper, we adopt the R-A model to characterize each coding tree unit (CTU) in an intra frame, and we propose a convolutional neural network (CNN) based approach to effectively predict the model parameters for every CTU. Then we develop a new CTU level bit allocation and bitrate control algorithm based on the R-A model for HEVC intra coding. The experimental results show that our proposed CNN-based approach outperforms the currently used rate control algorithm in HEVC reference software, leading to on average 0.46 percent decrease of rate control error and 0.7 percent BD-rate reduction.
更多查看译文
关键词
H.265/HEVC,Intra coding,Rate control,Convolutional neural network (CNN),R-A model
AI 理解论文
溯源树
样例
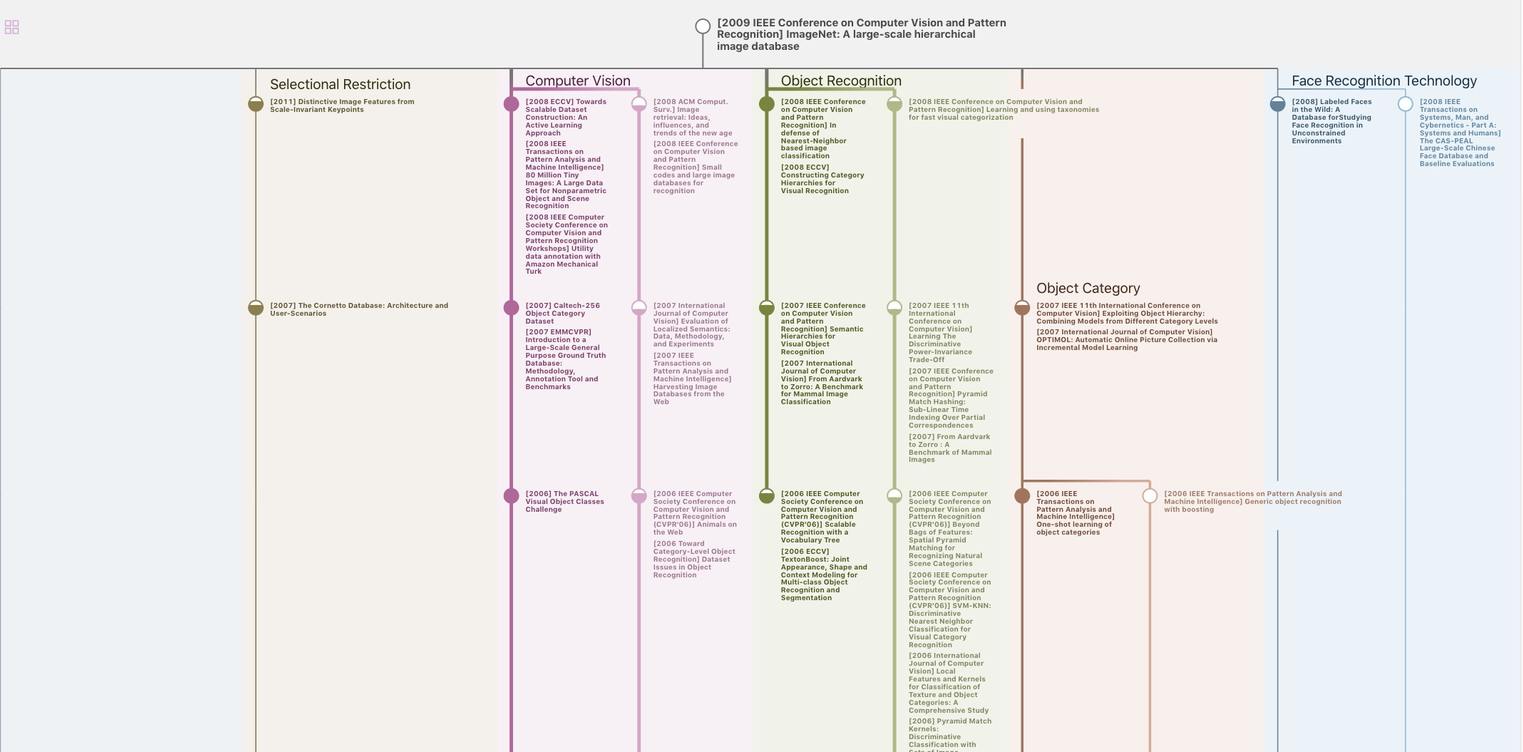
生成溯源树,研究论文发展脉络
Chat Paper
正在生成论文摘要