The δ-generalized multi-Bernoulli poisson filter in a multi-sensor application
2017 International Conference on Control, Automation and Information Sciences (ICCAIS)(2017)
摘要
This paper proposes a multi-target tracking strategy using a δ-Generalized Multi-Bernoulli Poisson (δ-GMBP) filter applied in a multi-sensor scenario. The δ-GMBP distribution is closed under the Chapman-Kolmogorov equation and Bayes rule, and also closed for a wide family of multi-target likelihood functions which allows implementations of different kinematic and measurement models. One difference between the δ-GMBP and the state of the art of multi-Bernoulli filters is that the birth process is modeled with a Poisson Random Finite Set (RFS), which can be more intuitive. Further, in order to obtain the posterior of the δ-GMBP filter recursion, it is not necessary to iterate over all the components of the prior mixture. The δ-GMBP filter, also maintains track labels in the multi-Bernoulli components, thus no other association method is necessary. The experiments carried out consist of people walking in an open place and two sensors recording the scene from a fixed position. The sensors used in the experiment are a 3D lidar and a single-beam mono-pulse radar. The δ-GMBP filter is compared with the classical Gaussian Mixture Probability Hypothesis Density (GM-PHD) filter, and the Marginal Multi-target Multi-Bernoulli (m-MeMBer) filter.
更多查看译文
关键词
random finite sets,multi-target tracking,multi-Bernoulli filter
AI 理解论文
溯源树
样例
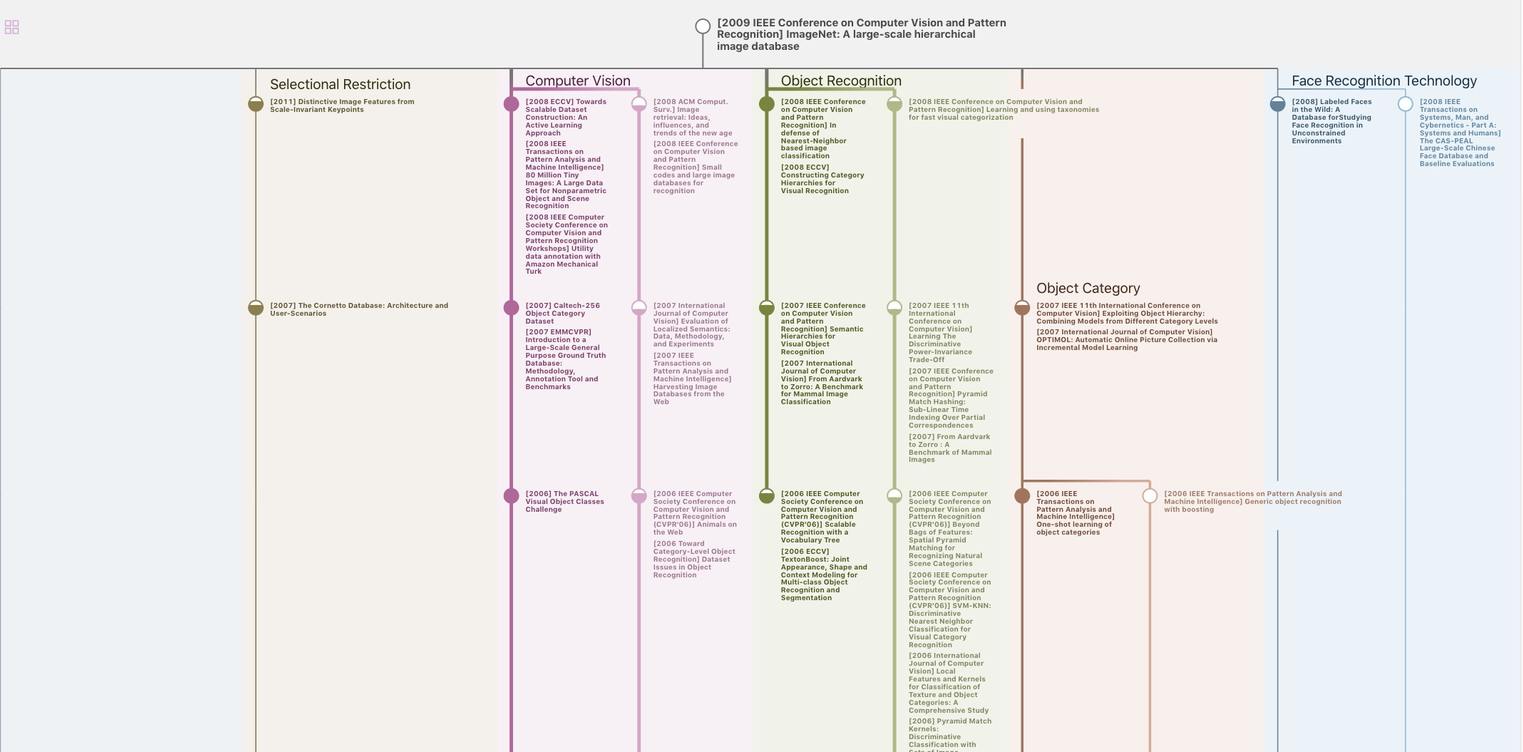
生成溯源树,研究论文发展脉络
Chat Paper
正在生成论文摘要