Bottom-Up Top-Down Cues for Weakly-Supervised Semantic Segmentation.
EMMCVPR(2017)
摘要
We consider the task of learning a classifier for semantic segmentation using weak supervision in the form of image labels specifying objects present in the image. Our method uses deep convolutional neural networks (cnns) and adopts an Expectation-Maximization (EM) based approach. We focus on the following three aspects of EM: (i) initialization; (ii) latent posterior estimation (E-step) and (iii) the parameter update (M-step). We show that saliency and attention maps, bottom-up and top-down cues respectively, of images with single objects (simple images) provide highly reliable cues to learn an initialization for the EM. Intuitively, given weak supervisions, we first learn to segment simple images and then move towards the complex ones. Next, for updating the parameters (M step), we propose to minimize the combination of the standard softmax loss and the KL divergence between the latent posterior distribution (obtained using the E-step) and the likelihood given by the cnn. This combination is more robust to wrong predictions made by the E step of the EM algorithm. Extensive experiments and discussions show that our method is very simple and intuitive, and outperforms the state-of-the-art method with a very high margin of 3.7% and 3.9% on the PASCAL VOC12 train and test sets respectively, thus setting new state-of-the-art results.
更多查看译文
关键词
Semantic Segmentation Task, Lateral Posterior, Image-level Labels, Weakly-supervised Setting, Saliency Map
AI 理解论文
溯源树
样例
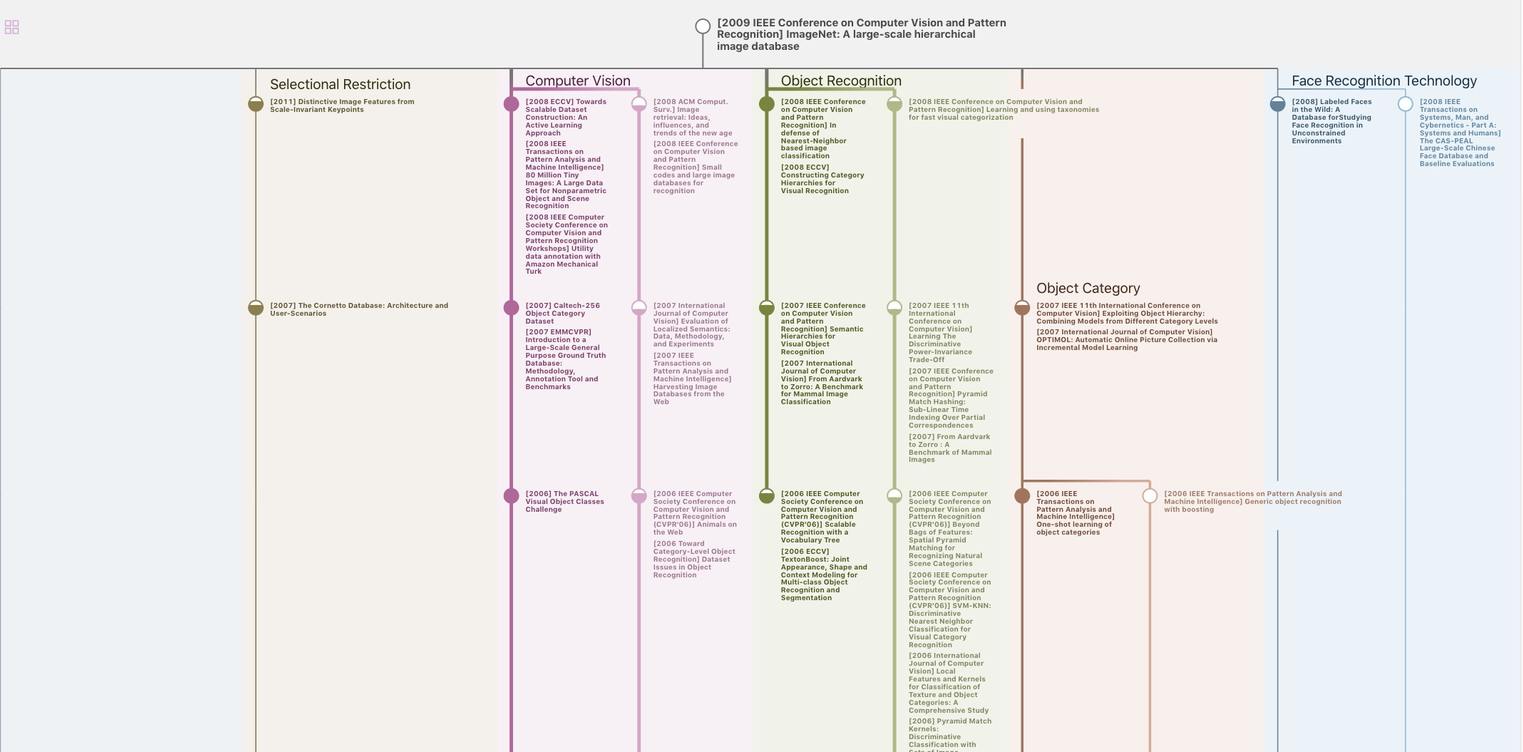
生成溯源树,研究论文发展脉络
Chat Paper
正在生成论文摘要