Deep SOMs for automated feature extraction and classification from big data streaming.
Proceedings of SPIE(2017)
摘要
In this paper, we proposed a deep self-organizing map model (Deep-SOMs) for automated features extracting and learning from big data streaming which we benefit from the framework Spark for real time streams and highly parallel data processing. The SOMs deep architecture is based on the notion of abstraction (patterns automatically extract from the raw data, from the less to more abstract). The proposed model consists of three hidden self-organizing layers, an input and an output layer. Each layer is made up of a multitude of SOMs, each map only focusing at local headmistress sub-region from the input image. Then, each layer trains the local information to generate more overall information in the higher layer. The proposed Deep-SOMs model is unique in terms of the layers architecture, the SOMs sampling method and learning. During the learning stage we use a set of unsupervised SOMs for feature extraction. We validate the effectiveness of our approach on large data sets such as Leukemia dataset and SRBCT. Results of comparison have shown that the Deep-SOMs model performs better than many existing algorithms for images classification.
更多查看译文
关键词
Deep SOMs,feature extraction,big data classification
AI 理解论文
溯源树
样例
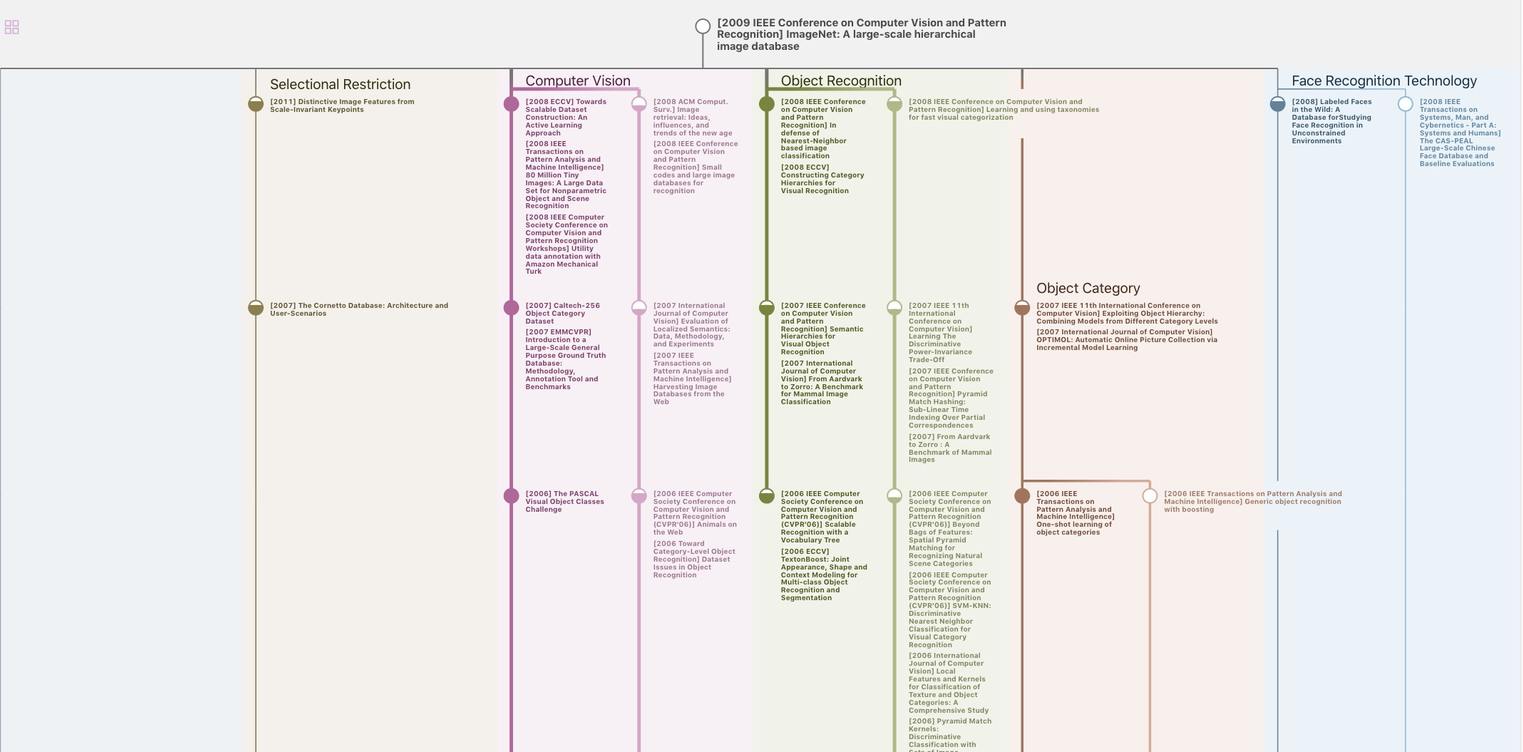
生成溯源树,研究论文发展脉络
Chat Paper
正在生成论文摘要