Aggregating Neural Word Embeddings for Document Representation.
ADVANCES IN INFORMATION RETRIEVAL (ECIR 2018)(2018)
摘要
Recent advances in natural language processing (NLP) have shown that semantically meaningful representations of words can be efficiently acquired by distributed models. In such a case, a text document can be viewed as a bag-of-word-embeddings (BoWE), and the remaining question is how to obtain a fixed-length vector representation of the document for efficient document process. Beyond those heuristic aggregation methods, recent work has shown that one can leverage the Fisher kernel (FK) framework to generate document representations based on BoWE in a principled way. In this work, words are embedded into a Euclidean space by latent semantic indexing (LSI), and a Gaussian Mixture Model (GMM) is employed as the generative model for nonlinear FK-based aggregation. In this work, we propose an alternate FK-based aggregation method for document representation based on neural word embeddings. As we know, neural embedding models have been proven significantly better performance in word representations than LSI, where semantic relations between neural word embeddings are typically measured by cosine similarity rather than Euclidean distance. Therefore, we introduce a mixture of Von Mises-Fisher distributions (moVMF) as the generative model of neural word embeddings, and derive a new FK-based aggregation method for document representation based on BoWE. We report document classification, clustering and retrieval experiments and demonstrate that our model can produce state-of-the-art performance as compared with existing baseline methods.
更多查看译文
AI 理解论文
溯源树
样例
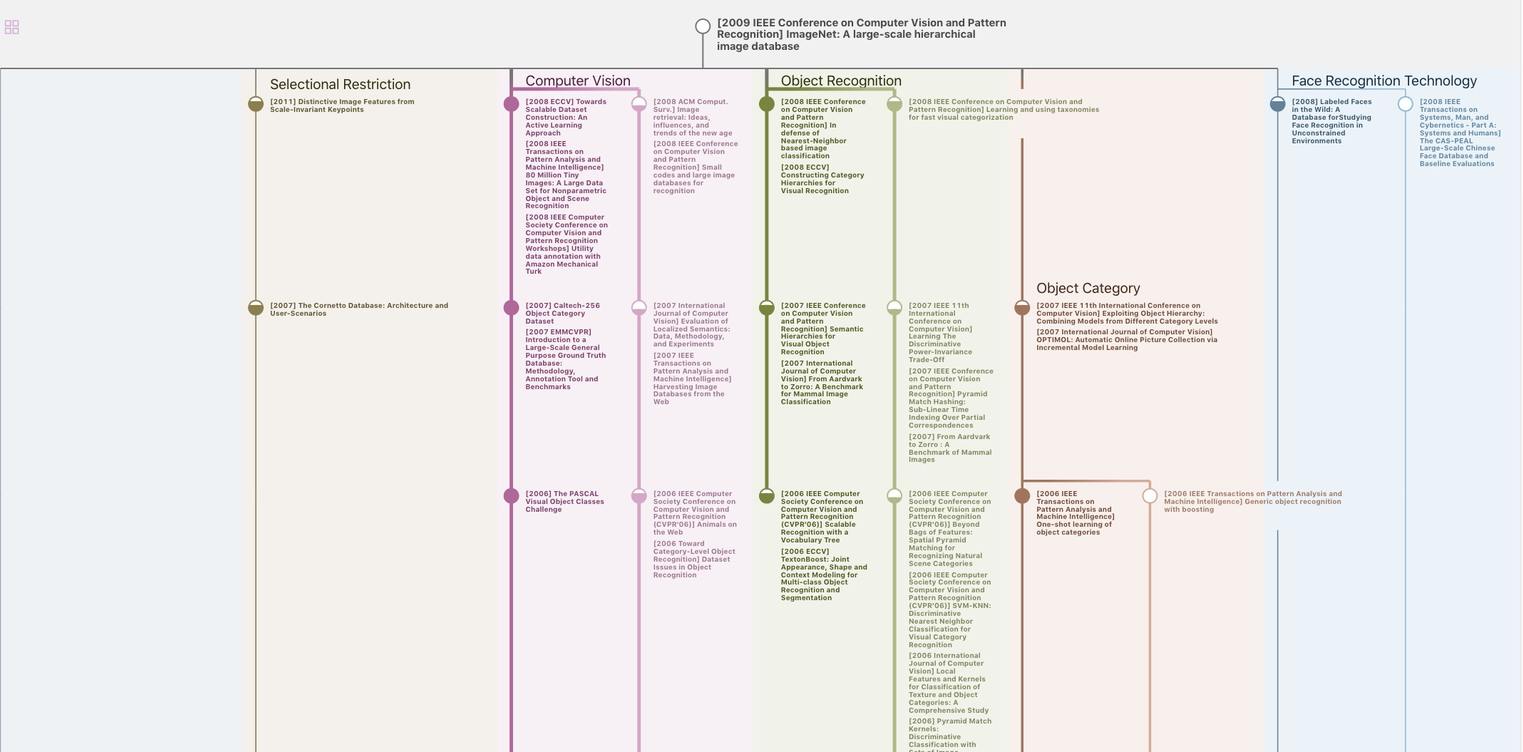
生成溯源树,研究论文发展脉络
Chat Paper
正在生成论文摘要