Benchmarking Bayesian Causal Discovery Methods for Downstream Treatment Effect Estimation
CoRR(2023)
摘要
The practical utility of causality in decision-making is widely recognized, with causal discovery and inference being inherently intertwined. Nevertheless, a notable gap exists in the evaluation of causal discovery methods, where insufficient emphasis is placed on downstream inference. To address this gap, we evaluate six established baseline causal discovery methods and a newly proposed method based on GFlowNets, on the downstream task of treatment effect estimation. Through the implementation of a robust evaluation procedure, we offer valuable insights into the efficacy of these causal discovery methods for treatment effect estimation, considering both synthetic and real-world scenarios, as well as low-data scenarios. Furthermore, the results of our study demonstrate that GFlowNets possess the capability to effectively capture a wide range of useful and diverse ATE modes.
更多查看译文
关键词
bayesian causal discovery methods,downstream treatment
AI 理解论文
溯源树
样例
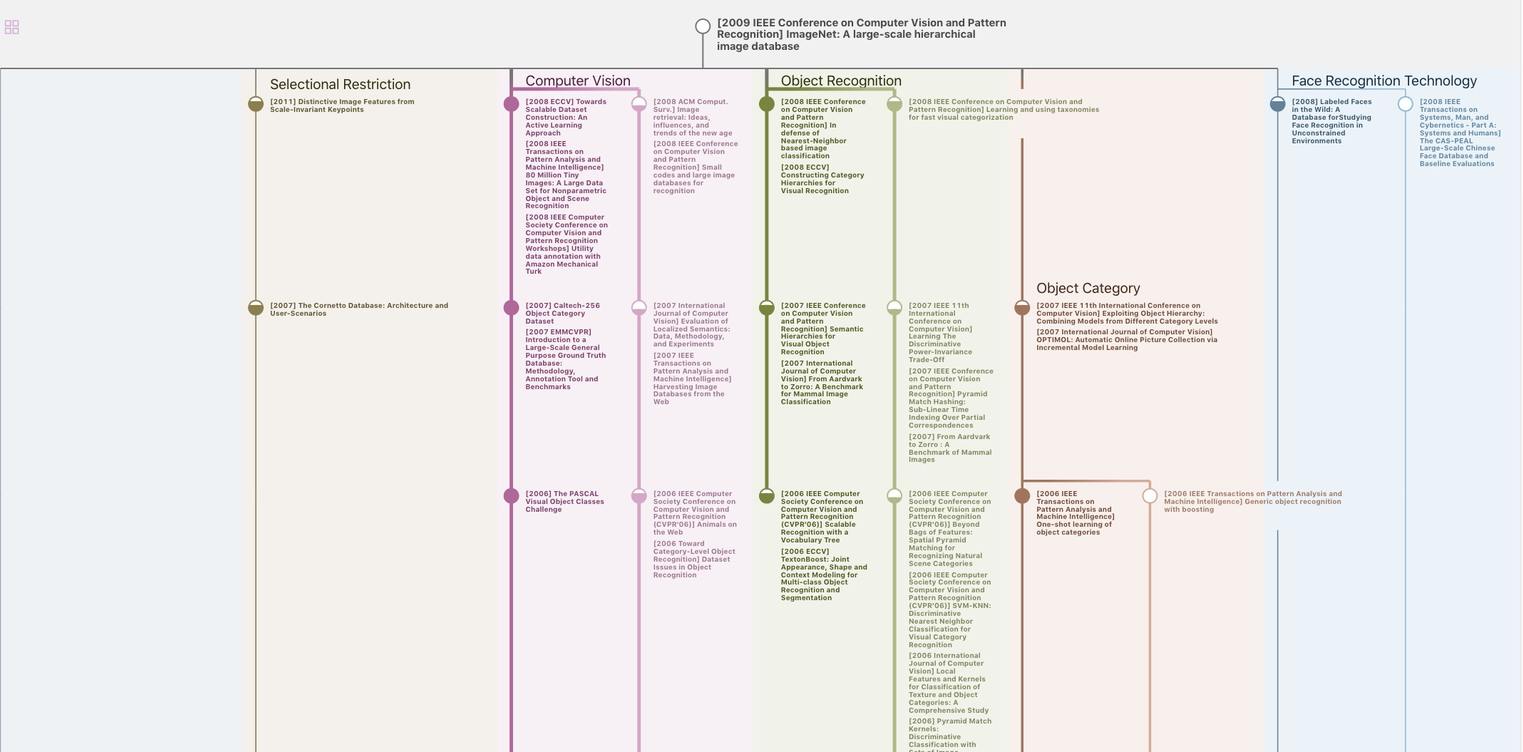
生成溯源树,研究论文发展脉络
Chat Paper
正在生成论文摘要