Improved TDNNs using Deep Kernels and Frequency Dependent Grid-RNNs
2018 IEEE International Conference on Acoustics, Speech and Signal Processing (ICASSP)(2018)
摘要
Time delay neural networks (TDNNs) are an effective acoustic model for large vocabulary speech recognition. The strength of the model can be attributed to its ability to effectively model long temporal contexts. However, current TDNN models are relatively shallow, which limits the modelling capability. This paper proposes a method of increasing the network depth by deepening the kernel used in the TDNN temporal convolutions. The best performing kernel consists of three fully connected layers with a residual (ResNet) connection from the output of the first to the output of the third. The addition of spectro-temporal processing as the input to the TDNN in the form of a convolutional neural network (CNN) and a newly designed Grid-RNN was investigated. The Grid-RNN strongly outperforms a CNN if different sets of parameters for different frequency bands are used and can be further enhanced by using a bi-directional Grid-RNN. Experiments using the multi-genre broadcast (MGB3) English data (275h) show that deep kernel TDNNs reduces the word error rate (WER) by 6% relative and when combined with the frequency dependent Grid-RNN gives a relative WER reduction of 9%.
更多查看译文
关键词
Time Delay Neural Network,Grid Recurrent Neural Network,Speech Recognition,ResNet
AI 理解论文
溯源树
样例
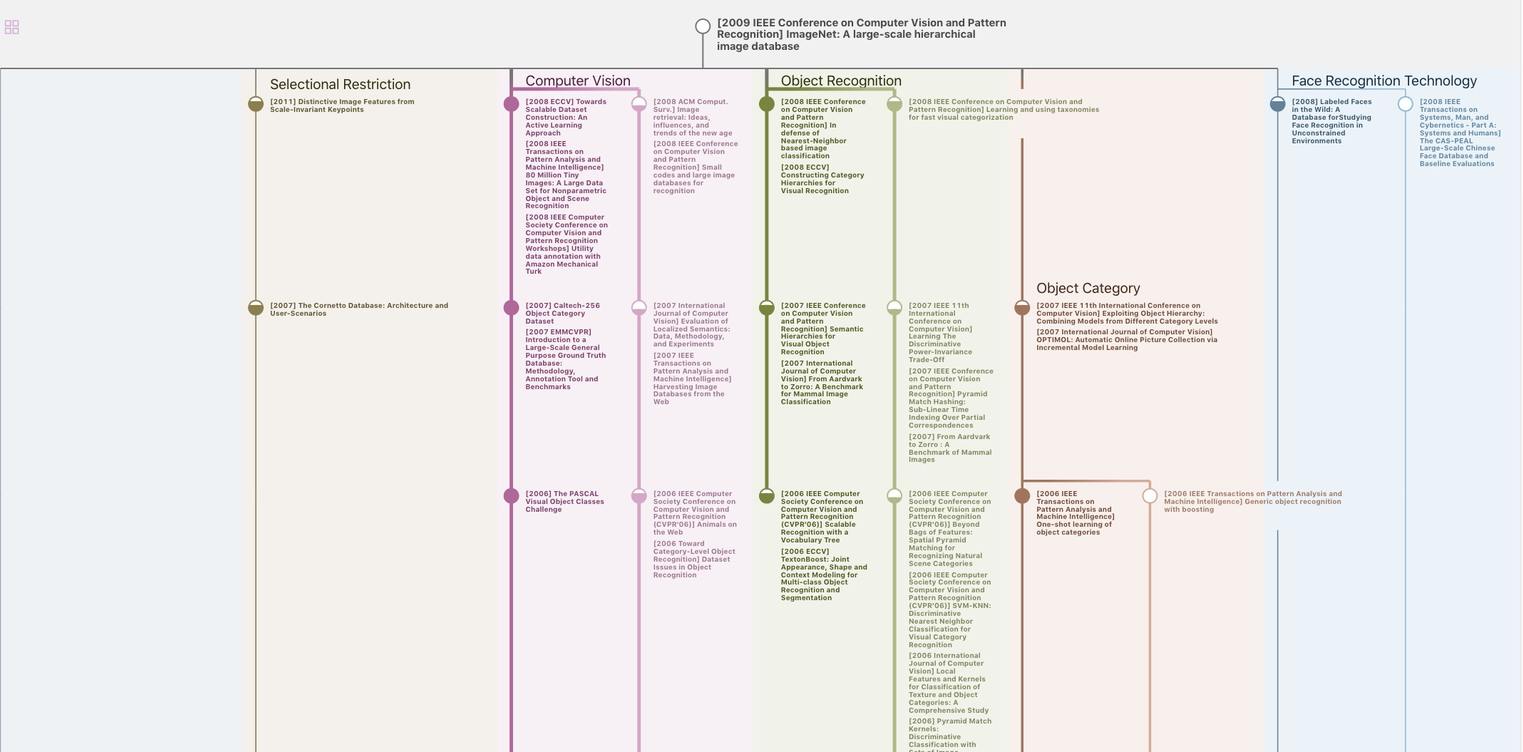
生成溯源树,研究论文发展脉络
Chat Paper
正在生成论文摘要